A Convolutional De-Quantization Network for Harmonics Suppression in One-Bit SAR Imaging
IEEE TRANSACTIONS ON GEOSCIENCE AND REMOTE SENSING(2023)
摘要
One-bit synthetic aperture radar (SAR) imaging, which collects echoes into one-bit quantized samples, is a highly promising technique for the faster SAR images acquisition and simplified implementations in numerous applications. Harmonics, accompanied with one-bit sampling operation, are one of the fundamental factors that trigger severe aliasing artifacts problem in frequency domain. For one-bit SAR imaging, rather than sampling the echoes with extremely high rate to alleviate the effects of harmonics, a convolutional de-quantization network, termed as CDQOB-net, is proposed to establish de-aliasing in a transformed imagery domain. The proposed method starts with a fresh interpretation on the regular range-Doppler (RD) imaging, which is also referred to as the 2-D transformed domain of SAR echo. Then, in the light of deep learning framework for image restoration, an alternative method designed in the perspective of harmonics is specifically analyzed, wherein the degradation caused by harmonics in transformed domain is sufficiently estimated. Consequently, as a data-driven method, our two-stage CDQOB-net is proposed and trained to handle aliasing harmonics and multiple distortions, which can significantly improve the quality of one-bit SAR imaging. The proposed method is easy to implement and computationally efficient. Extensive experiments on simulated and real SAR data also demonstrate its validation and effectiveness.
更多查看译文
关键词
Deep neural networks,Fourier image restoration,harmonic suppression,one-bit sampling,synthetic aperture radar (SAR) imaging
AI 理解论文
溯源树
样例
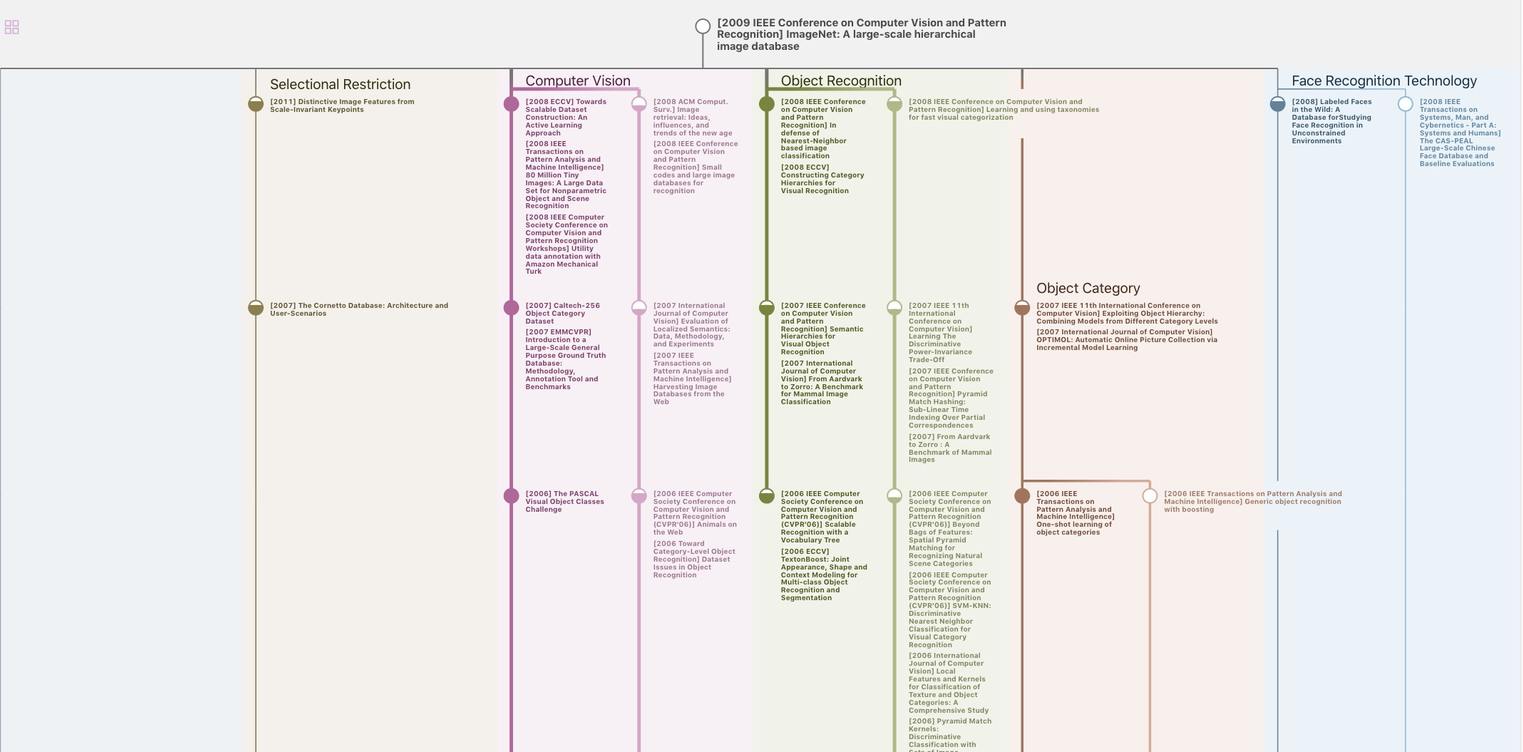
生成溯源树,研究论文发展脉络
Chat Paper
正在生成论文摘要