Crossed Siamese Vision Graph Neural Network for Remote-Sensing Image Change Detection
IEEE TRANSACTIONS ON GEOSCIENCE AND REMOTE SENSING(2023)
摘要
The development of deep learning in remote-sensing (RS) visual tasks has led to remarkable progress in RS image change detection (CD). However, RS bi-temporal images cover complex and confusing scenes due to natural environmental factors, which presents challenges for CD tasks. How to effectively exploit long-range dependencies and sensitively discriminate real changes with various scales from pseudo-changes are urgent problems. It is especially obvious for the changes in building structures man-made. This article presents a CD approach named CSViG, which utilizes a Siamese vision graph neural network (SViG) with crossed feature fusion. SViG acts as a feature extractor to capture richer short- and long-range dependencies. Crossed feature fusion consists of a horizontal feature fusion module (HFFM) and a vertical feature fusion module (VFFM). HFFM designs a cross-concatenation (CC) way to reveal real changes from pseudo-changes in the same horizontal stage, after which global and local features are extracted by using an attention mechanism and multiscale depth-wise separable convolution (DSConv). VFFM further fuses complementary content from vertical multiple stages to effectively represent change regions of different sizes (tiny or huge) by using an attention mechanism. Extensive comparative experiments conducted on three available building CD datasets demonstrate that the proposed method achieves better CD performance than previous counterparts.
更多查看译文
关键词
Attention mechanism,change detection (CD),deep learning,feature fusion,graph neural networks,remote-sensing (RS)
AI 理解论文
溯源树
样例
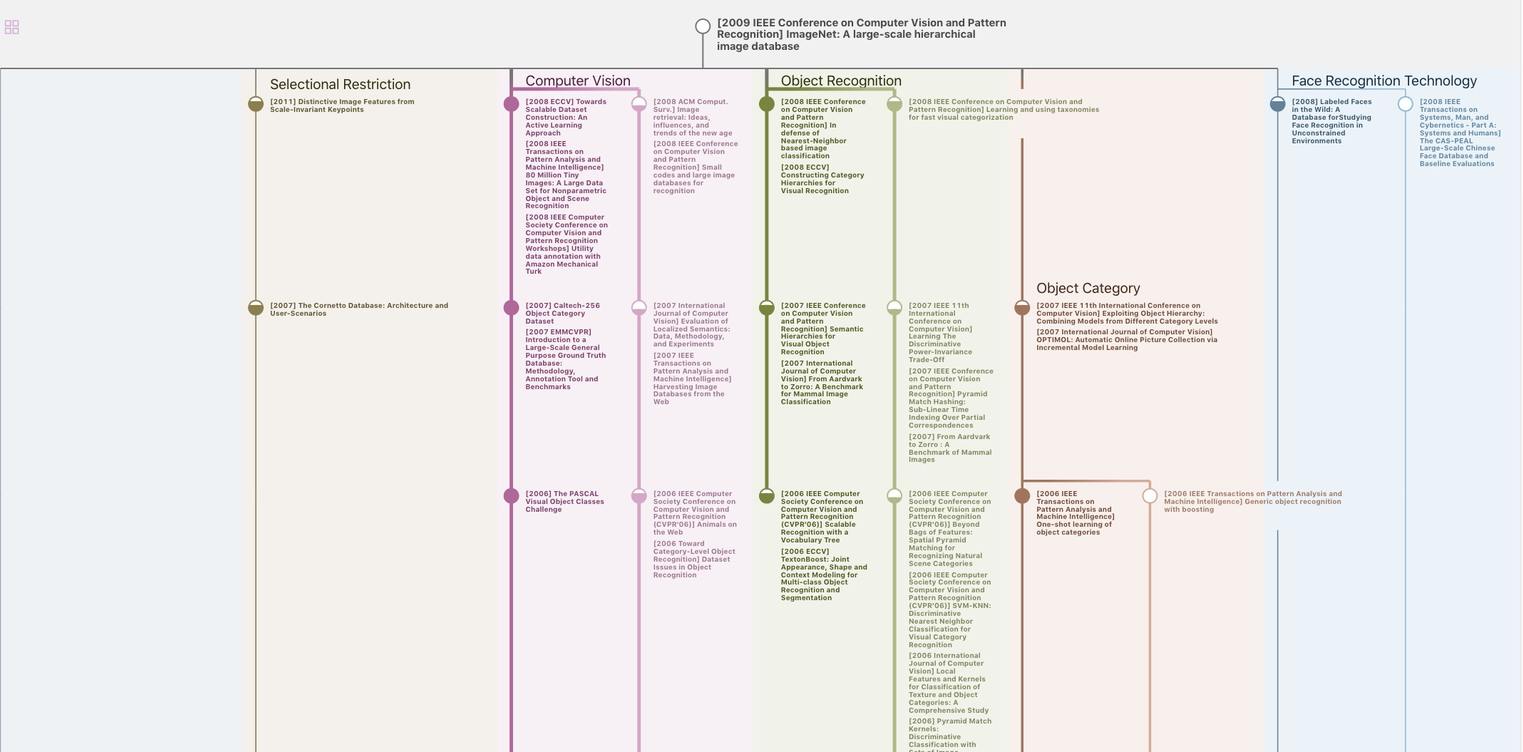
生成溯源树,研究论文发展脉络
Chat Paper
正在生成论文摘要