Multiscale Encoder-Decoder Network for DAS Data Simultaneous Denoising and Reconstruction
IEEE TRANSACTIONS ON GEOSCIENCE AND REMOTE SENSING(2023)
摘要
Distributed acoustic sensing (DAS) has been considered a breakthrough technique in seismic data collection owing to its advantages in acquisition cost and accuracy. However, the existence of complex background noise combined with a tough exploration environment always results in incomplete data with a low signal-to-noise ratio (SNR), posing a big challenge for the subsequent processing of DAS data. To improve the quality of DAS data, convolutional neural networks (CNNs) have gradually been utilized to deal with the denoising and reconstruction tasks. Meanwhile, some successful applications have verified that CNN-based methods can significantly alleviate the impacts of DAS background noise and missing trace records, compared with conventional approaches. Nonetheless, in most research, the denoising and reconstruction tasks are accomplished independently, severely affecting the processing efficiency. In this study, a multiscale encoder-decoder network (MEDN) is proposed to simultaneously achieve the DAS background noise suppression and weak signal recovery through a unified model. Generally, MEDN can extract the different-scale features through both a multiscale network architecture and a multiscale residual (MSR) block. The captured different-scale features are then fused to enhance the effective feature. In addition, the encoder-decoder scheme is also utilized in the design of the network architecture to further enhance the reconstruction performance. Moreover, depthwise separable convolution (DSC) blocks are also utilized to ease the computational burden and improve the processing efficiency. Theoretical and field data processing results show that MEDN can provide better denoising and reconstruction performance than conventional methods and popular CNN-based frameworks.
更多查看译文
关键词
Noise reduction,Optimized production technology,Task analysis,Computational efficiency,Training,Feature extraction,Background noise,Convolutional neural network (CNN),distributed acoustic sensing (DAS) noise suppression,multiscale network,noisy incomplete seismic data,seismic data interpolation
AI 理解论文
溯源树
样例
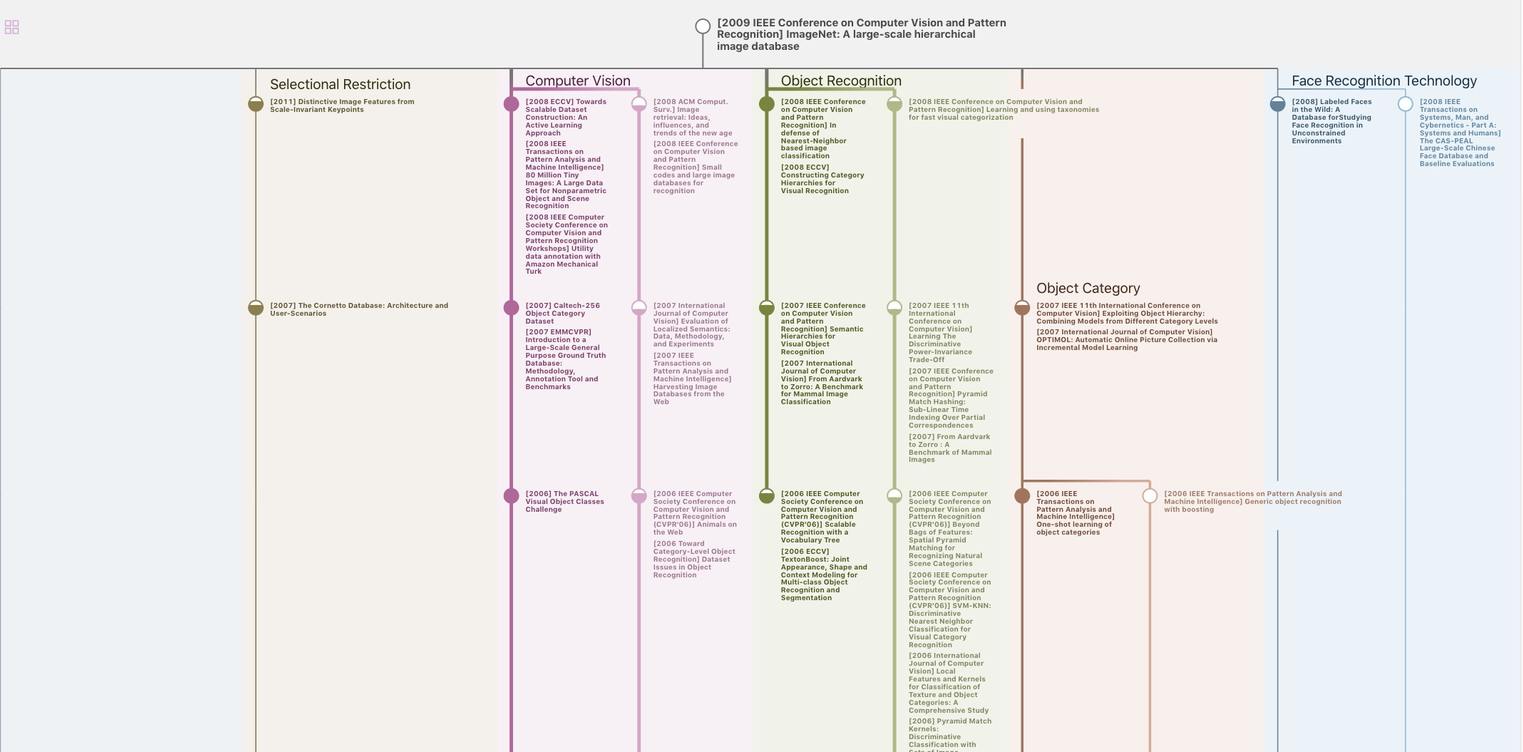
生成溯源树,研究论文发展脉络
Chat Paper
正在生成论文摘要