Intrinsic Decomposition Model-Guided Two-Stream Coupled Autoencoder for Unsupervised Hyperspectral Image Change Detection
IEEE Geoscience and Remote Sensing Letters(2023)
摘要
Hyperspectral image change detection (HSI-CD) is one of the main research topics in remote sensing. Theoretically, the ground objects can be considered to have changed when their spectral features behave differently. However, in practical scenarios, this presupposition does not hold as the collected features are affected by many factors, such as illumination conditions, atmospheric effects, and topographic changes. Inspired by the intrinsic image decomposition, we propose a novel unsupervised deep-learning (DL) framework based on a two-stream coupled autoencoder (TSCA) to cope with bi-temporal co-registered HSI-CD. The network consists of two symmetric encoders and a decoder, which can jointly decompose bi-temporal images into abundance coefficients corresponding to the same set of spectral bases. As our network separates the component of spectral variation from multiple images, the extracted abundance features with inherent properties of materials can provide better performance for change detection (CD). Moreover, to enforce alignment of the feature space, a reasonable consistency loss is devised to constrain the solution space, by cross-reconstruction in both branches. Experimental results demonstrate its superiority over the recently developed state of the arts.
更多查看译文
关键词
model-guided,two-stream
AI 理解论文
溯源树
样例
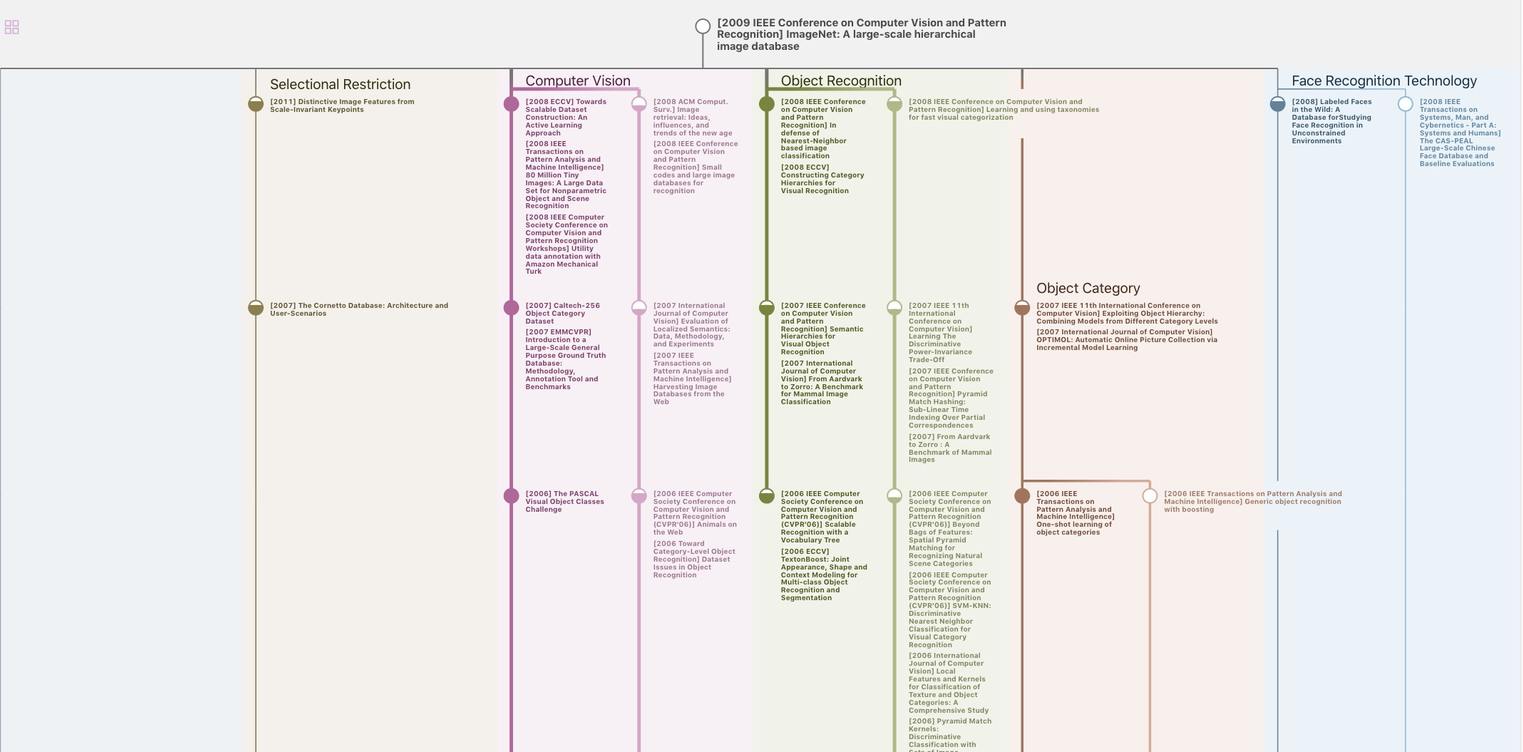
生成溯源树,研究论文发展脉络
Chat Paper
正在生成论文摘要