Toward the Production of Spatiotemporally Consistent Annual Land Cover Maps Using Sentinel-2 Time Series
IEEE GEOSCIENCE AND REMOTE SENSING LETTERS(2023)
摘要
Land cover (LC) maps generated by the classification of remote-sensing (RS) data allow for monitoring Earth processes and the dynamics of objects and phenomena. For accurate LC variability quantification in environmental monitoring, maps need to be spatiotemporally consistent, continually updated, and indicate permanent changes. However, producing frequent and spatiotemporally consistent LC maps is challenging because it involves balancing the need for temporal consistency with the risk of missing real changes. In this work, we propose a scalable and semiautomatic method for generating annual LC maps with labels that are consistently applied from one year to the next. It uses a Transformer deep-learning (DL) model as a classifier, which is trained on satellite time series (TS) of images using high performance computing (HPC). The trained model can generate stable maps by shifting the prediction window along the temporal direction. The effectiveness of the proposed approach is tested qualitatively and quantitatively on a multiannual Sentinel-2 dataset acquired over a three-year period in a study area located in the southern Italian Alps.
更多查看译文
关键词
Deep learning (DL),high-performance comput-ing,remote sensing (RS),spatiotemporally consistent land cover (LC) maps,supervised classification,time series (TS)
AI 理解论文
溯源树
样例
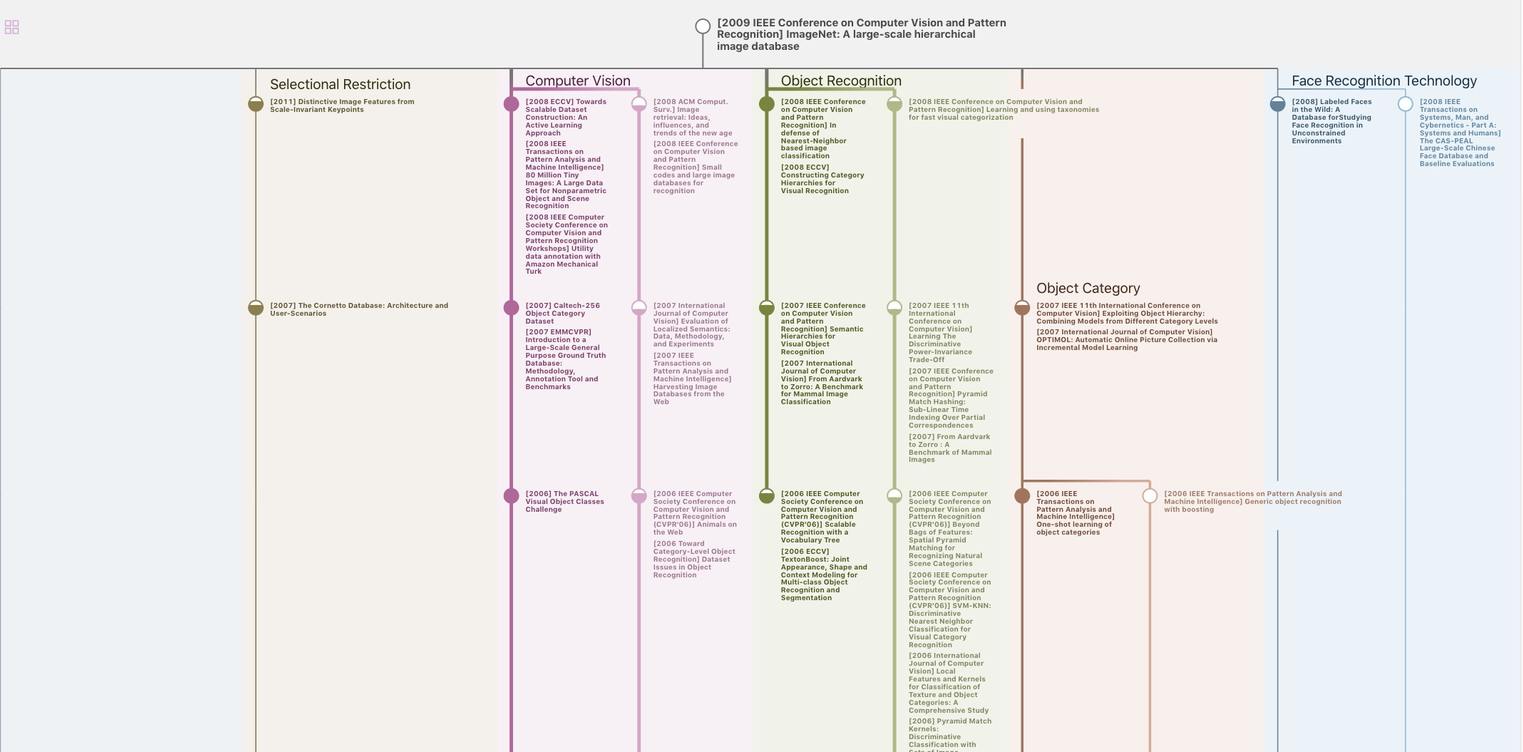
生成溯源树,研究论文发展脉络
Chat Paper
正在生成论文摘要