MSDU: Multi-step Delayed Communication for Efficient Distributed Deep Learning
2023 4th International Conference on Big Data, Artificial Intelligence and Internet of Things Engineering (ICBAIE)(2023)
摘要
Distributed deep learning has emerged as the principal training paradigm in recent years. However, significant communication overhead often leads to a severe degradation of performance in data parallelism, which limits the scalability of distributed training. To address this problem, this paper introduce a novel MSDU (Multi-Step Delayed Update) method. MSDU mitigates the negative impact of communication overhead on training efficiency by introducing a delay in the parameter aggregation process. Which allowing for overlap between computation and communication. As a result, MSDU can improve the performance of distributed deep learning, particularly in scenarios with limited communication bandwidth, such as PCIe-based communication. Experimental results demonstrate that employing the MSDU method in data parallelism can significantly reduce training time by up to 45.7%, with only a limited loss of model accuracy.
更多查看译文
关键词
component,data parallel,distributed deep learning,communication optimize,synchronization
AI 理解论文
溯源树
样例
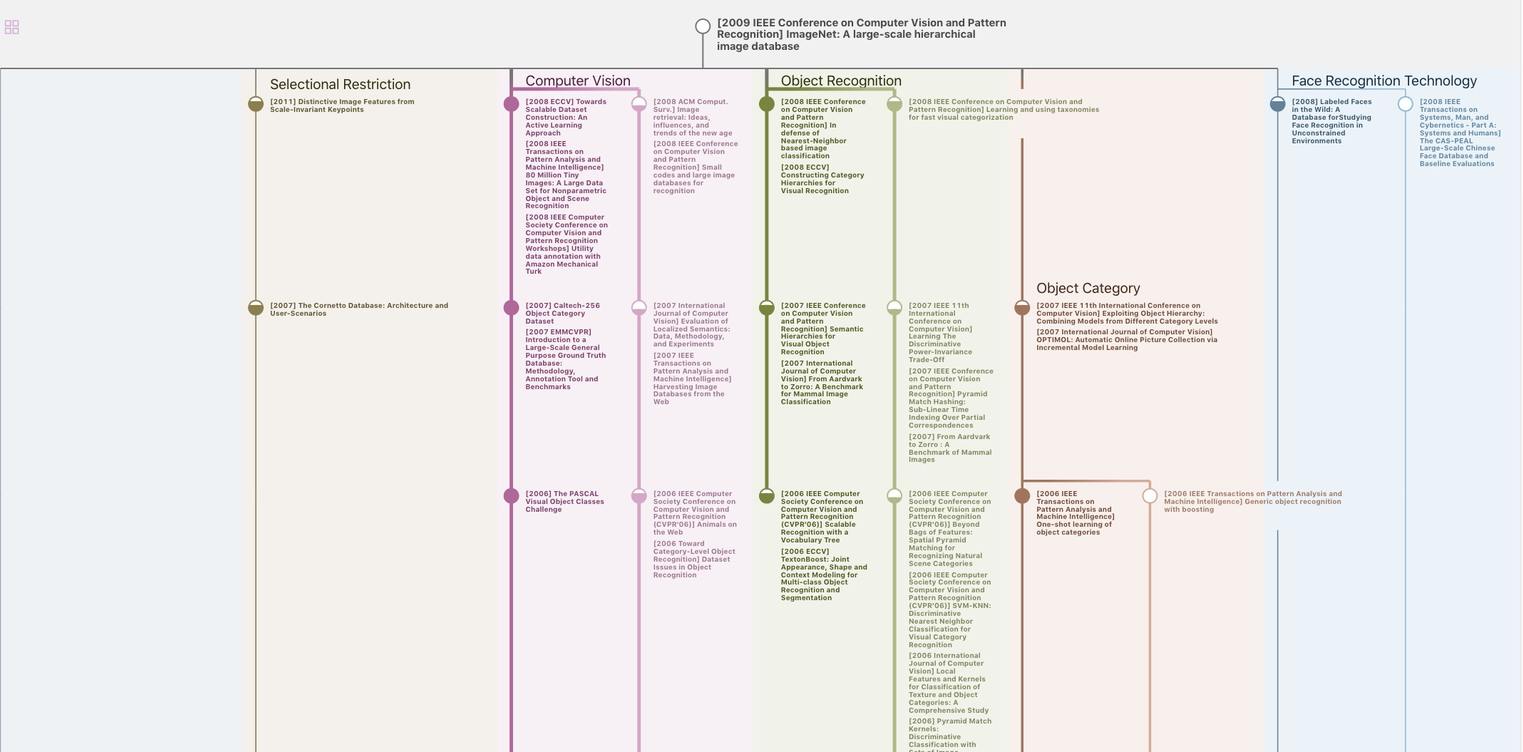
生成溯源树,研究论文发展脉络
Chat Paper
正在生成论文摘要