Video-Based Visible-Infrared Person Re-Identification With Auxiliary Samples
IEEE TRANSACTIONS ON INFORMATION FORENSICS AND SECURITY(2024)
摘要
Visible-infrared person re-identification (VI-ReID) aims to match persons captured by visible and infrared cameras, allowing person retrieval and tracking in 24-hour surveillance systems. Previous methods focus on learning from cross-modality person images in different cameras. However, temporal information and single-camera samples tend to be neglected. To crack this nut, in this paper, we first contribute a large-scale VI-ReID dataset named BUPTCampus. Different from most existing VI-ReID datasets, it 1) collects tracklets instead of images to introduce rich temporal information, 2) contains pixel-aligned cross-modality sample pairs for better modality-invariant learning, 3) provides one auxiliary set to help enhance the optimization, in which each identity only appears in a single camera. Based on our constructed dataset, we present a two-stream framework as baseline and apply Generative Adversarial Network (GAN) to narrow the gap between the two modalities. To exploit the advantages introduced by the auxiliary set, we propose a curriculum learning based strategy to jointly learn from both primary and auxiliary sets. Moreover, we design a novel temporal k-reciprocal re-ranking method to refine the ranking list with fine-grained temporal correlation cues. Experimental results demonstrate the effectiveness of the proposed methods. We also reproduce 9 state-of-the-art image-based and video-based VI-ReID methods on BUPTCampus and our methods show substantial superiority to them. The codes and dataset are available at: https://github.com/dyhBUPT/BUPTCampus.
更多查看译文
关键词
Task analysis,Cameras,Training,Optimization,Generative adversarial networks,Representation learning,Radar tracking,Visible-infrared person re-identification,curriculum learning,re-ranking
AI 理解论文
溯源树
样例
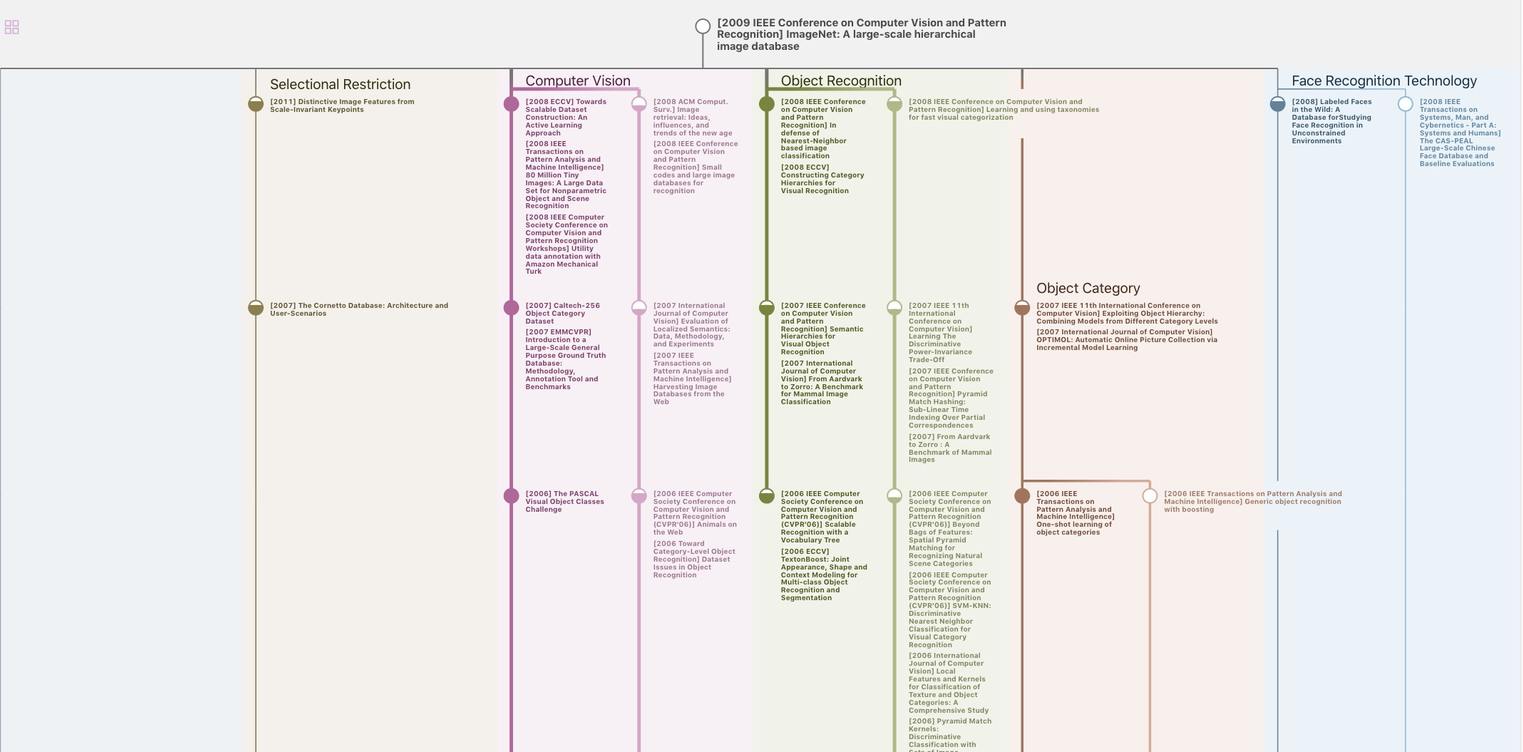
生成溯源树,研究论文发展脉络
Chat Paper
正在生成论文摘要