Spatio-temporal insights for wind energy harvesting in South Africa
arxiv(2023)
摘要
Understanding complex spatial dependency structures is a crucial
consideration when attempting to build a modeling framework for wind speeds.
Ideally, wind speed modeling should be very efficient since the wind speed can
vary significantly from day to day or even hour to hour. But complex models
usually require high computational resources. This paper illustrates how to
construct and implement a hierarchical Bayesian model for wind speeds using the
Weibull density function based on a continuously-indexed spatial field. For
efficient (near real-time) inference the proposed model is implemented in the r
package R-INLA, based on the integrated nested Laplace approximation (INLA).
Specific attention is given to the theoretical and practical considerations of
including a spatial component within a Bayesian hierarchical model. The
proposed model is then applied and evaluated using a large volume of real data
sourced from the coastal regions of South Africa between 2011 and 2021. By
projecting the mean and standard deviation of the Matern field, the results
show that the spatial modeling component is effectively capturing variation in
wind speeds which cannot be explained by the other model components. The mean
of the spatial field varies between $\pm 0.3$ across the domain. These insights
are valuable for planning and implementation of green energy resources such as
wind farms in South Africa. Furthermore, shortcomings in the spatial sampling
domain is evident in the analysis and this is important for future sampling
strategies. The proposed model, and the conglomerated dataset, can serve as a
foundational framework for future investigations into wind energy in South
Africa.
更多查看译文
AI 理解论文
溯源树
样例
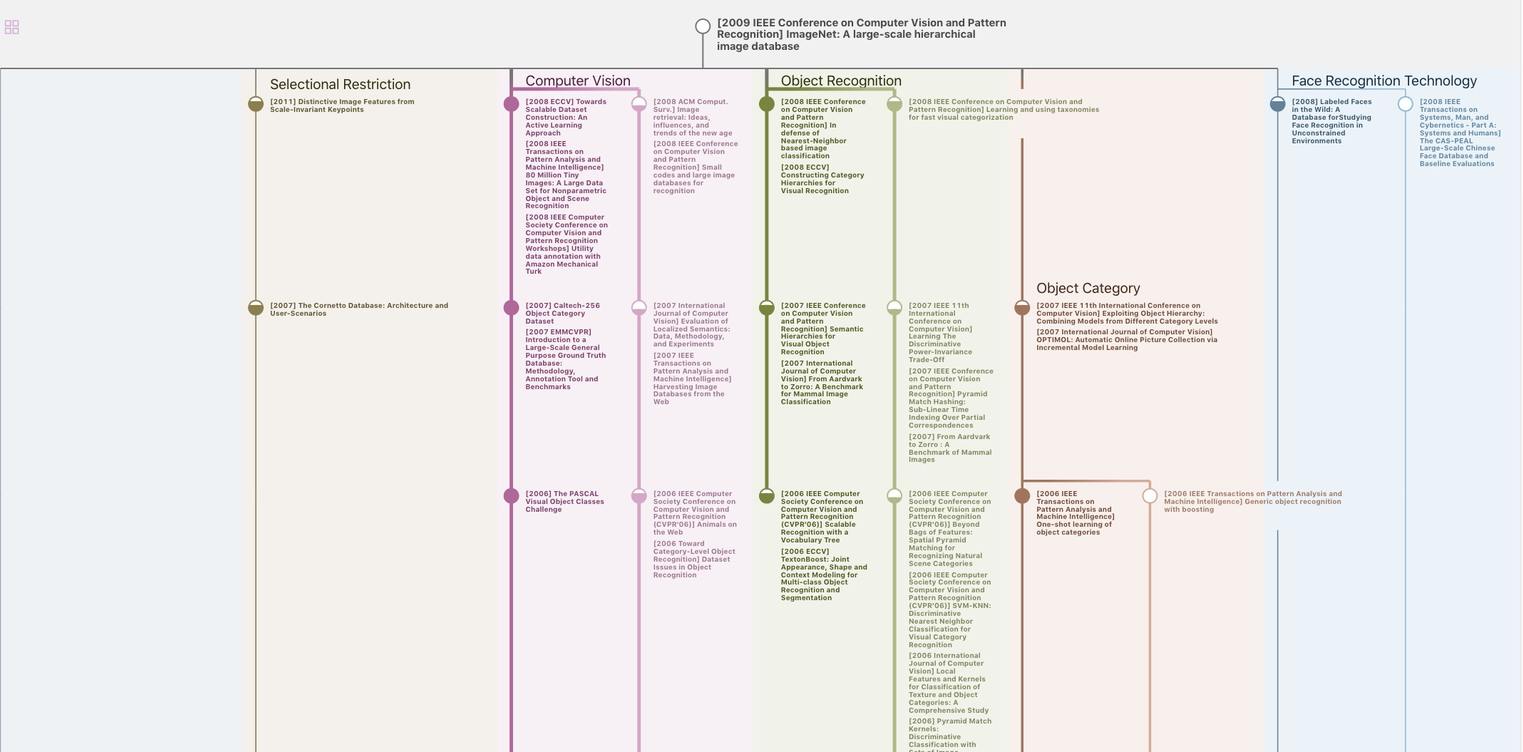
生成溯源树,研究论文发展脉络
Chat Paper
正在生成论文摘要