Singular layer physics informed neural network method for plane parallel flows
Computers & Mathematics with Applications(2024)
摘要
We construct in this article the semi-analytic Physics Informed Neural Networks (PINNs), called singular layer PINNs (or sl-PINNs), that are suitable to predict the stiff solutions of plane-parallel flows at a small viscosity. Recalling the boundary layer analysis, we first find the corrector for the problem which describes the singular behavior of the viscous flow inside boundary layers. Then, using the components of the corrector and its curl, we build our new sl-PINN predictions for the velocity and the vorticity by either embedding the explicit expression of the corrector (or its curl) in the structure of PINNs or by training the implicit parts of the corrector (or its curl) together with the PINN predictions. Numerical experiments confirm that our new sl-PINNs produce stable and accurate predicted solutions for the plane-parallel flows at a small viscosity.
更多查看译文
关键词
Singular perturbations,Physics-informed neural networks,Plane-parallel flow,Boundary layers
AI 理解论文
溯源树
样例
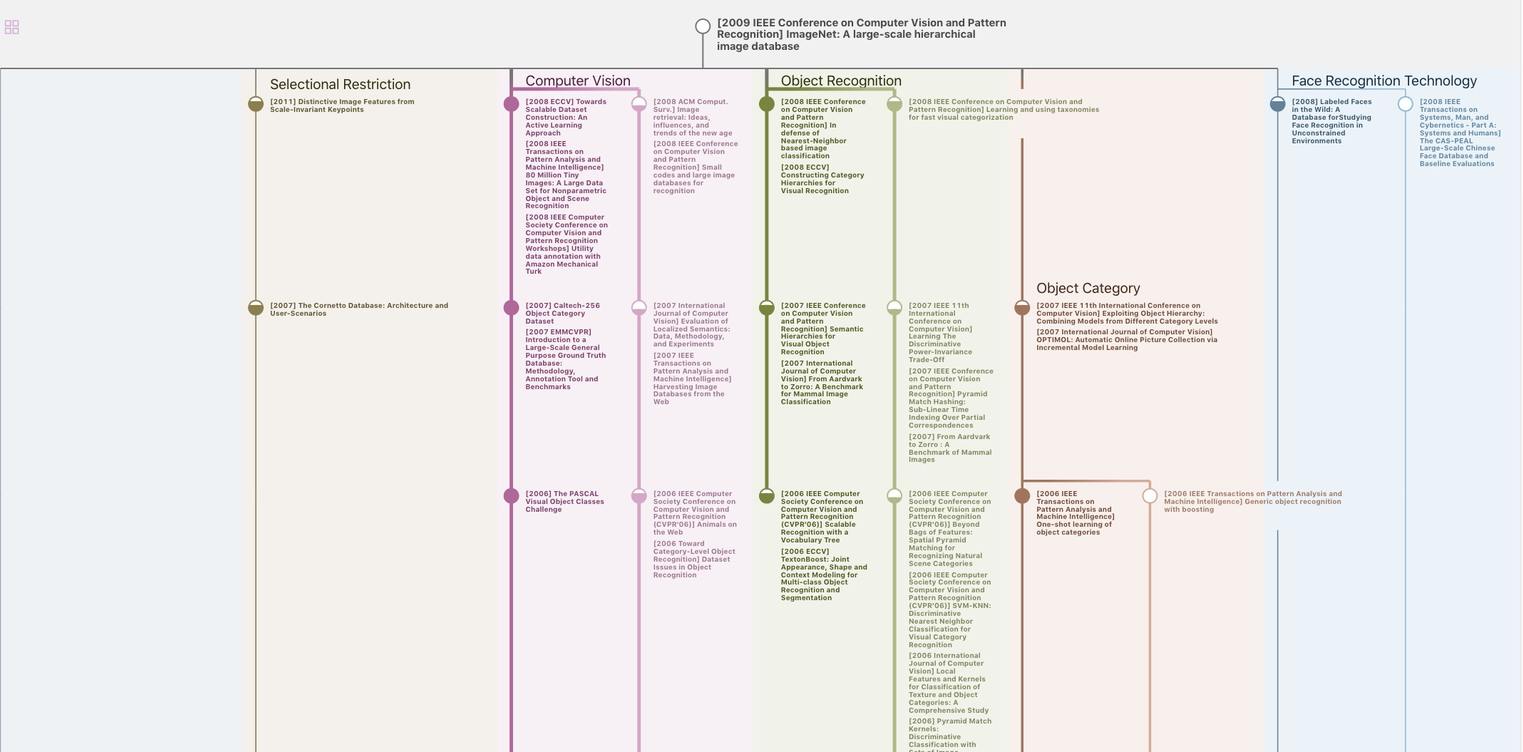
生成溯源树,研究论文发展脉络
Chat Paper
正在生成论文摘要