Making predictions using poorly identified mathematical models
biorxiv(2024)
摘要
Many mathematical models that are commonly used in the field of mathematical biology involve challenges of parameter non-identifiability. Practical non-identifiability, where the quality and quantity of data does not provide sufficiently precise parameter estimates is often encountered, even with relatively simple models. In particular, the situation where some parameters are identifiable and others are not identifiable is often encountered. In this work we apply a recent likelihood-based workflow, called Profile-Wise Analysis (PWA), to non-identifiable models for the first time. The PWA workflow addresses identifiability, parameter estimation, and prediction in a unified framework that is simple to implement and interpret. Previous implementations of the workflow have dealt with idealised identifiable problems only. In this work we illustrate how the PWA workflow can be applied to both structurally non-identifiable and practically non-identifiable models in the context of widely used, simple population growth models. Dealing with simple mathematical models allows us to present the PWA workflow in a didactic, self--contained document. Furthermore, working with simple mathematical models allows the PWA workflow prediction intervals to be compared with gold standard full likelihood prediction intervals. Together, our examples illustrate how the PWA workflow provides us with a systematic way of dealing with non-identifiability, especially compared to other approaches, such as seeking ad hoc parameter combinations, or simply setting parameter values to some arbitrary default value. Importantly, we show that the PWA workflow provides insight into the commonly--encountered situation where some parameters are identifiable and others are not, allowing us to explore how uncertainty in some parameters, and combinations of some parameters, regardless of their identifiability status, influences model predictions in a way that is insightful and interpretable.
### Competing Interest Statement
The authors have declared no competing interest.
更多查看译文
AI 理解论文
溯源树
样例
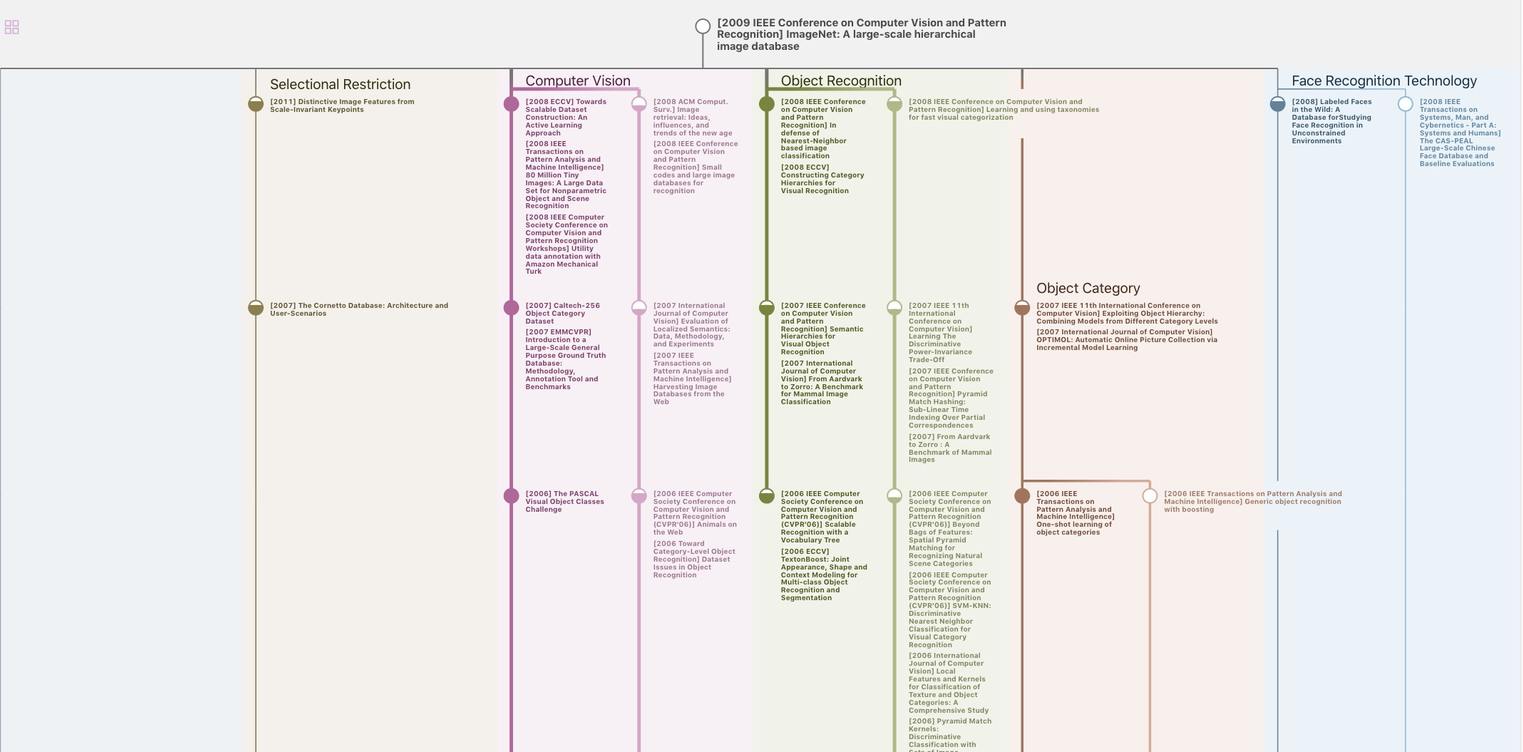
生成溯源树,研究论文发展脉络
Chat Paper
正在生成论文摘要