Fair Influence Maximization in Social Networks: A Community-Based Evolutionary Algorithm.
CoRR(2023)
摘要
Influence Maximization (IM) has been extensively studied in network science,
which attempts to find a subset of users to maximize the influence spread. A
new variant of IM, Fair Influence Maximization (FIM), which primarily enhances
the fair propagation of information, attracts increasing attention in academic.
However, existing algorithms for FIM suffer from a trade-off between fairness
and running time. Since it is a tough task to ensure that users are fairly
influenced in terms of sensitive attributes, such as race or gender, while
maintaining a high influence spread. To tackle this problem, in this paper, we
propose an effective and efficient Community-based Evolutionary Algorithm for
FIM (named CEA-FIM). In CEA-FIM, a community-based node selection strategy is
proposed to identify potential nodes, which not only considers the size of the
community but also the attributes of the nodes in the community. Subsequently,
we design an evolutionary algorithm based on the proposed node selection
strategy to hasten the search for the optimal solution, including the novel
initialization, crossover and mutation strategies. We validate the proposed
algorithm CEA-FIM by performing experiments on real-world and synthetic
networks. The experimental results show that the proposed CEA-FIM achieves a
better balance between effectiveness and efficiency, compared to the
state-of-the-art baseline algorithms.
更多查看译文
AI 理解论文
溯源树
样例
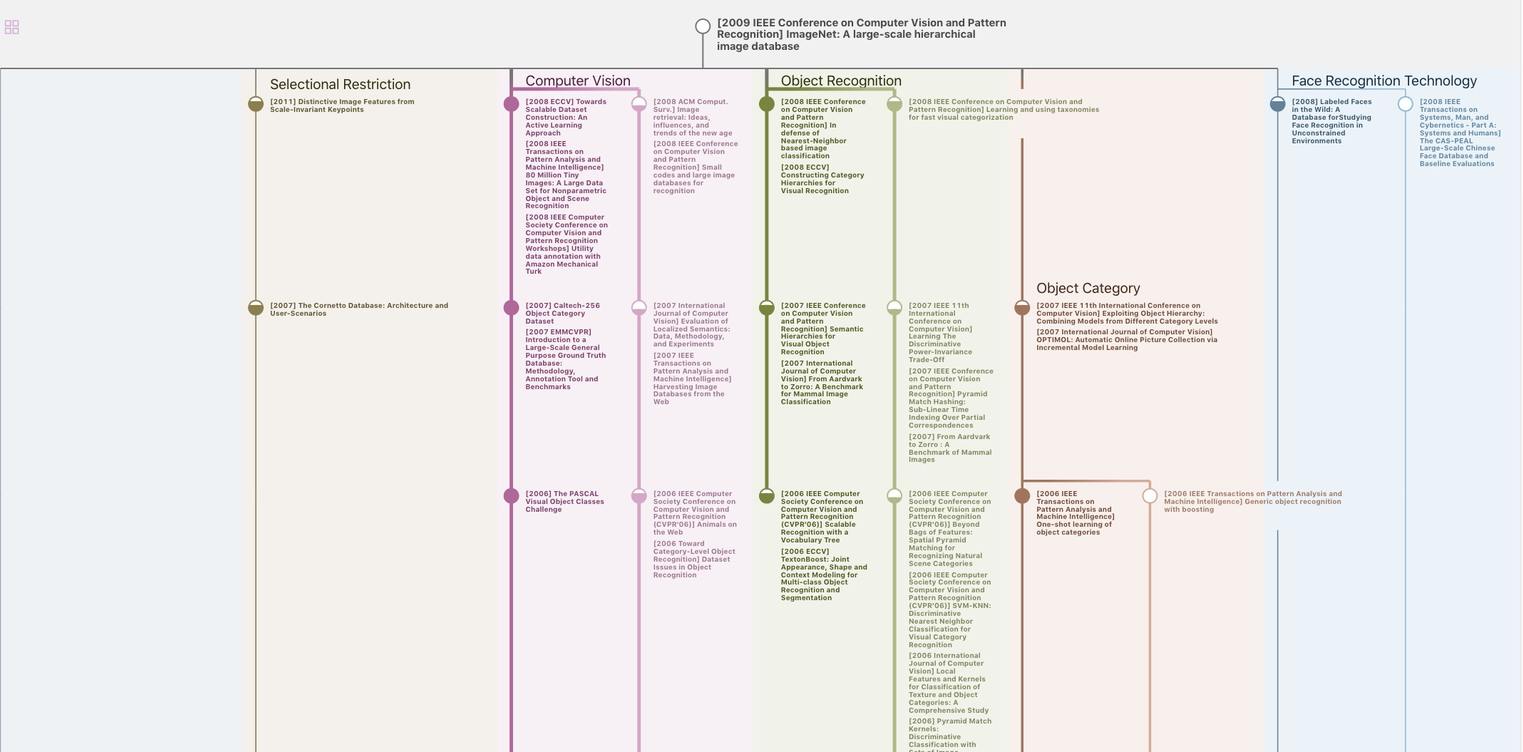
生成溯源树,研究论文发展脉络
Chat Paper
正在生成论文摘要