Bias and Multiscale Correction Methods for Variational State Estimation Algorithms.
CoRR(2023)
摘要
The integration of experimental data into mathematical and computational
models is crucial for enhancing their predictive power in real-world scenarios.
However, the performance of data assimilation algorithms can be significantly
degraded when measurements are corrupted by biased noise, altering the signal
magnitude, or when the system dynamics lack smoothness, such as in the presence
of fast oscillations or discontinuities. This paper focuses on variational
state estimation using the so-called Parameterized Background Data Weak method,
which relies on a parameterized background by a set of constraints, enabling
state estimation by solving a minimization problem on a reduced-order
background model, subject to constraints imposed by the input measurements. To
address biased noise in observations, a modified formulation is proposed,
incorporating a correction mechanism to handle rapid oscillations by treating
them as slow-decaying modes based on a two-scale splitting of the classical
reconstruction algorithm. The effectiveness of the proposed algorithms is
demonstrated through various examples, including discontinuous signals and
simulated Doppler ultrasound data.
更多查看译文
AI 理解论文
溯源树
样例
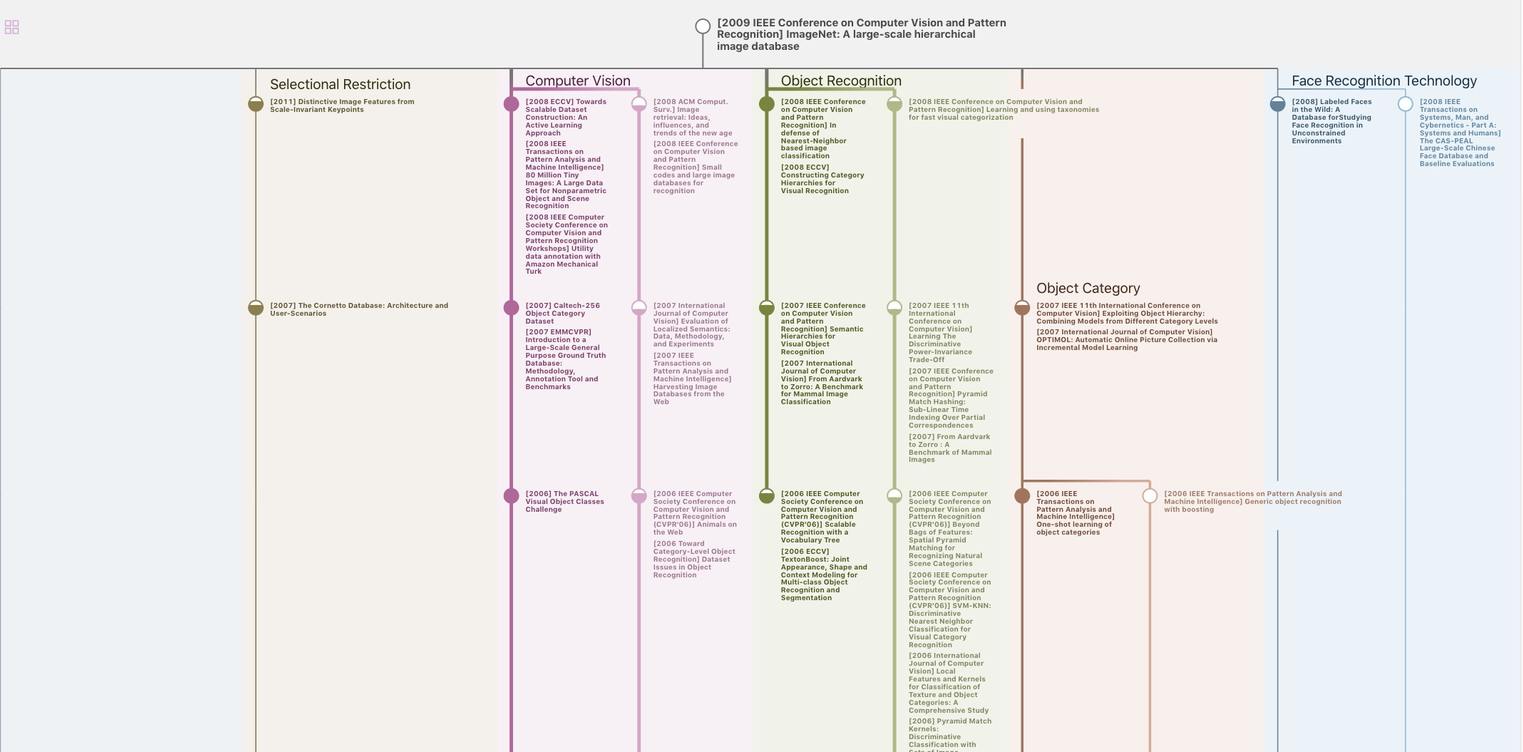
生成溯源树,研究论文发展脉络
Chat Paper
正在生成论文摘要