Windformer:Bi-Directional Long-Distance Spatio-Temporal Network For Wind Speed Prediction
CoRR(2023)
摘要
Wind speed prediction is critical to the management of wind power generation.
Due to the large range of wind speed fluctuations and wake effect, there may
also be strong correlations between long-distance wind turbines. This
difficult-to-extract feature has become a bottleneck for improving accuracy.
History and future time information includes the trend of airflow changes,
whether this dynamic information can be utilized will also affect the
prediction effect. In response to the above problems, this paper proposes
Windformer. First, Windformer divides the wind turbine cluster into multiple
non-overlapping windows and calculates correlations inside the windows, then
shifts the windows partially to provide connectivity between windows, and
finally fuses multi-channel features based on detailed and global information.
To dynamically model the change process of wind speed, this paper extracts time
series in both history and future directions simultaneously. Compared with
other current-advanced methods, the Mean Square Error (MSE) of Windformer is
reduced by 0.5\% to 15\% on two datasets from NERL.
更多查看译文
AI 理解论文
溯源树
样例
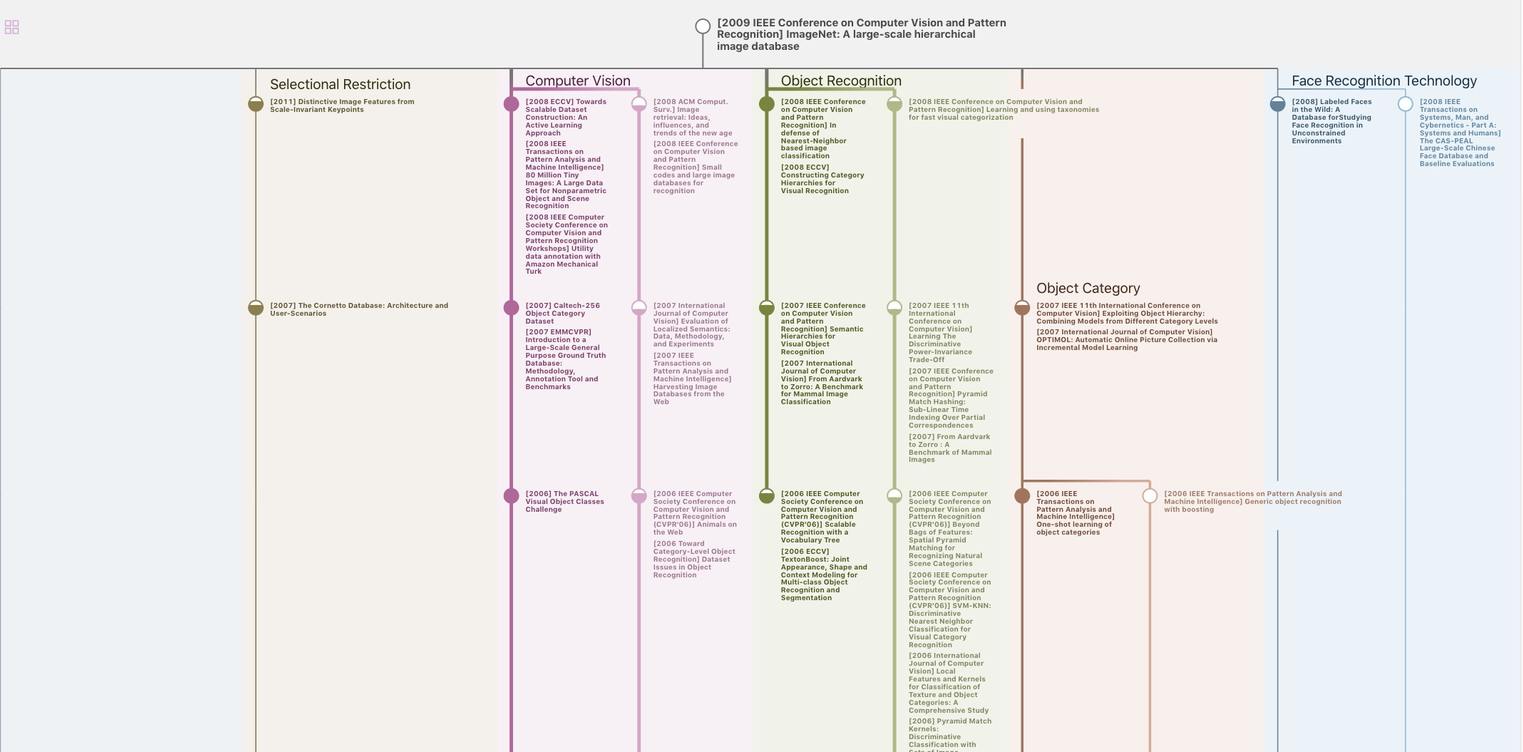
生成溯源树,研究论文发展脉络
Chat Paper
正在生成论文摘要