Prediction of protein-ligand binding affinity with deep learning
COMPUTATIONAL AND STRUCTURAL BIOTECHNOLOGY JOURNAL(2023)
摘要
The prediction of binding affinities between target proteins and small molecule drugs is essential for speeding up the drug research and design process. To attain precise and effective affinity prediction, computer-aided methods are employed in the drug discovery pipeline. In the last decade, a variety of computational methods has been developed, with deep learning being the most commonly used approach. We have gathered several deep learning methods and classified them into convolutional neural networks (CNNs), graph neural networks (GNNs), and Transformers for analysis and discussion. Initially, we conducted an analysis of the different deep learning methods, focusing on their feature construction and model architecture. We discussed the advantages and disadvantages of each model. Subsequently, we conducted experiments using four deep learning methods on the PDBbind v.2016 core set. We evaluated their prediction capabilities in various affinity intervals and statistically and visually analyzed the samples of correct and incorrect predictions for each model. Through visual analysis, we attempted to combine the strengths of the four models to improve the Root Mean Square Error (RMSE) of predicted affinities by 1.6% (reducing the absolute value to 1.101) and the Pearson Correlation Coefficient (R) by 2.9% (increasing the absolute value to 0.894) compared to the current state-of-the-art method. Lastly, we discussed the challenges faced by current deep learning methods in affinity prediction and proposed potential solutions to address these issues.
更多查看译文
关键词
Protein-ligand affinity,Binding affinity prediction,Deep learning,Assemble model
AI 理解论文
溯源树
样例
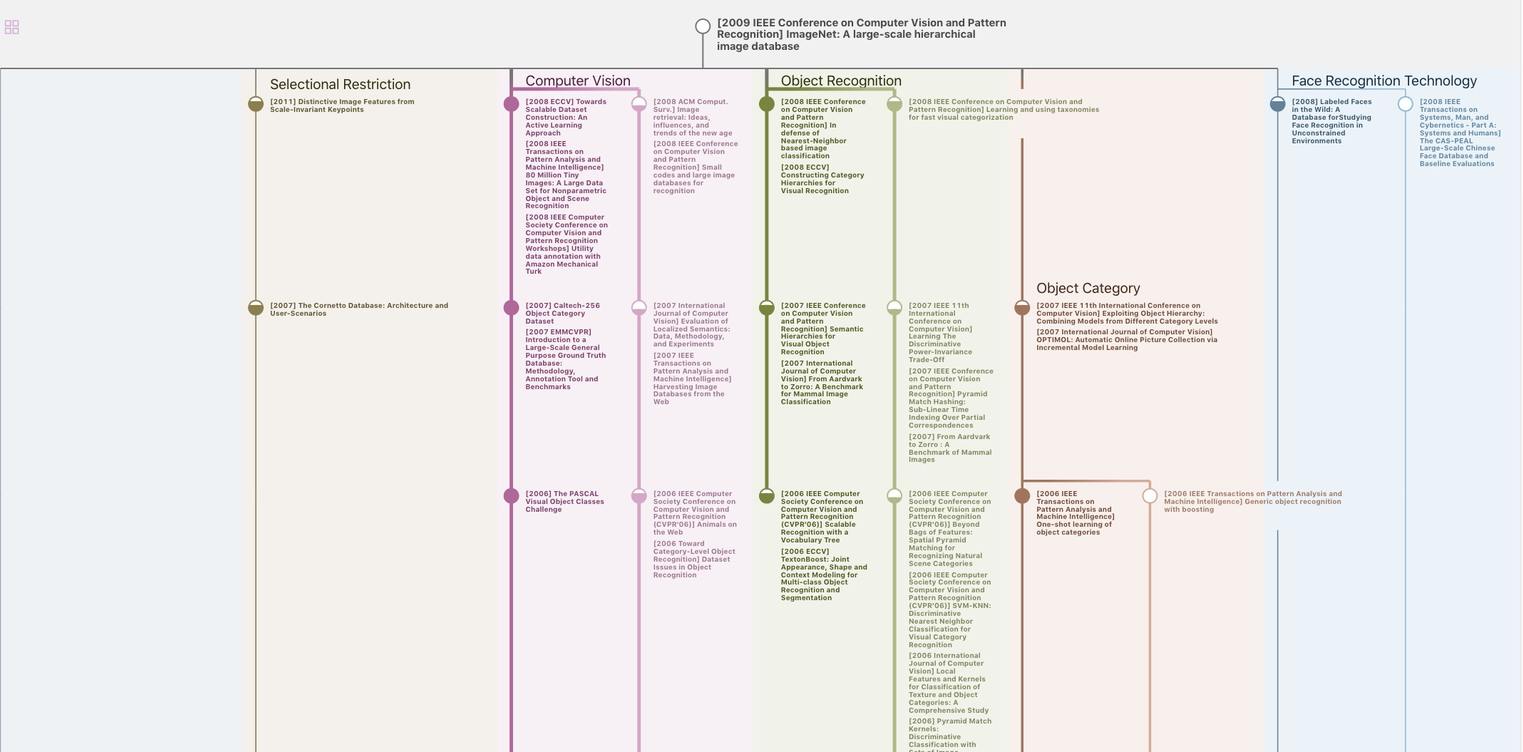
生成溯源树,研究论文发展脉络
Chat Paper
正在生成论文摘要