EEG based emotion recognition by hierarchical bayesian spectral regression framework
JOURNAL OF NEUROSCIENCE METHODS(2024)
摘要
Spectral regression (SR), a graph-based learning regression model, can be used to extract features from graphs to realize efficient dimensionality reduction. However, due to the SR method remains a regularized least squares problem and being defined in L2-norm space, the effect of artifacts in EEG signals cannot be efficiently resisted. In this work, to further improve the robustness of the graph-based regression models, we propose to utilize the prior distribution estimation in the Bayesian framework and develop a robust hierarchical Bayesian spectral regression framework (named HB-SR), which is designed with the hierarchical Bayesian ensemble strategies. In the proposed HB-SR, the impact of noises can be effectively reduced by the adaptive adjustment approach in model parameters with the data-driven manner. Specifically, in the current work, three different distributions have been elaborately designed to enhance the universality of the proposed HB-SR, i.e., Gaussian distribution, Laplace distribution, and Student-t distribution. To objectively evaluate the performance of the HB-SR framework, we conducted both simulation studies and emotion recognition experiments based on emotional EEG signals. Experimental results have consistently indicated that compared with other existing spectral regression methods, the proposed HB-SR can effectively suppress the influence of noises and achieve robust EEG emotion recognition.
更多查看译文
关键词
Spectral regression,Hierarchical Bayesian,Dimensionality reduction,EEG signals,Brain network,Emotion recognition
AI 理解论文
溯源树
样例
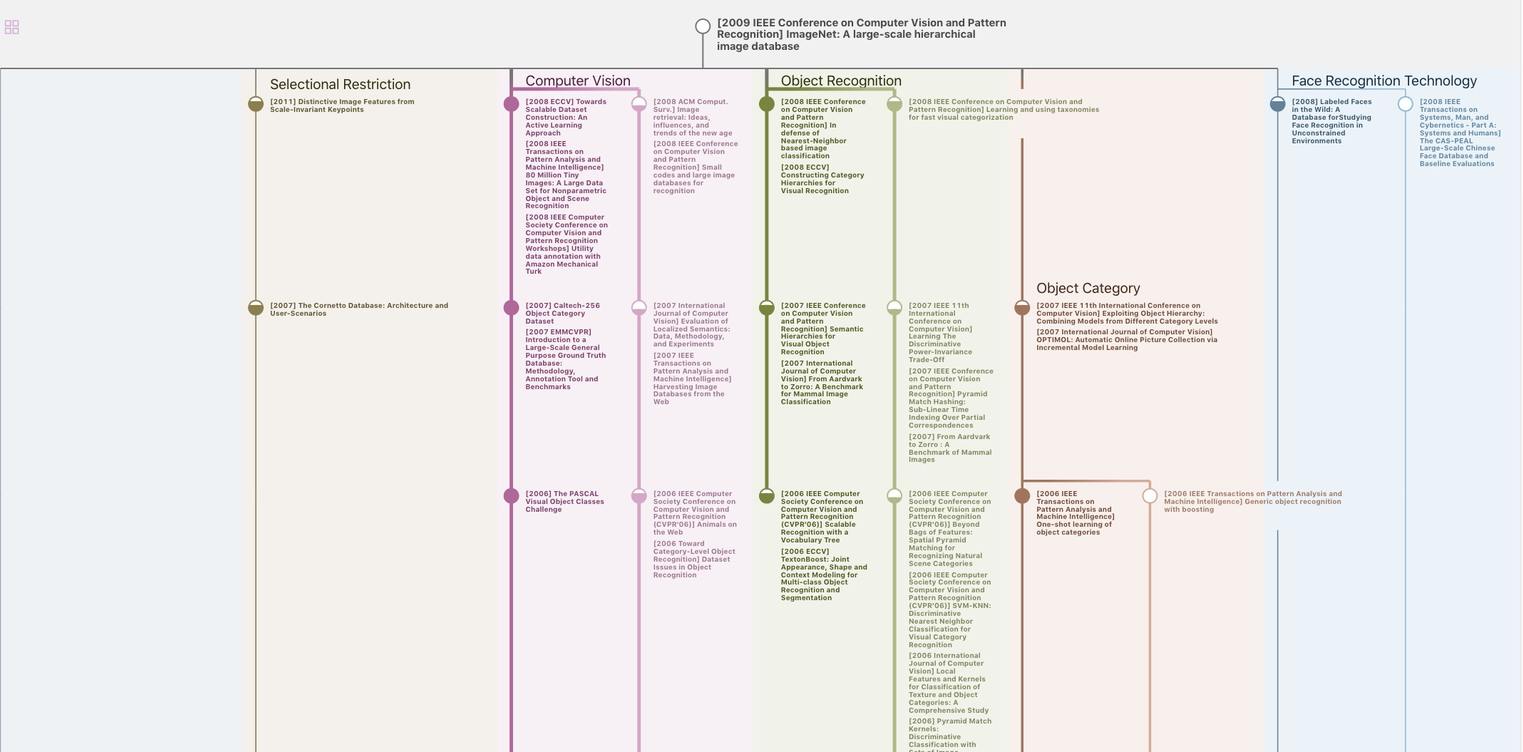
生成溯源树,研究论文发展脉络
Chat Paper
正在生成论文摘要