Radial magnetic resonance image reconstruction with a deep unrolled projected fast iterative soft-thresholding network
COMPUTERS IN BIOLOGY AND MEDICINE(2024)
摘要
Radially sampling of magnetic resonance imaging (MRI) is an effective way to accelerate the imaging. How to preserve the image details in reconstruction is always challenging. In this work, a deep unrolled neural network is designed to emulate the iterative sparse image reconstruction process of a projected fast soft-threshold algorithm (pFISTA). The proposed method, an unrolled pFISTA network for Deep Radial MRI (pFISTA-DR), include the preprocessing module to refine coil sensitivity maps and initial reconstructed image, the learnable convolution filters to extract image feature maps, and adaptive threshold to robustly remove image artifacts. Experimental results show that, among the compared methods, pFISTA-DR provides the best reconstruction and achieved the highest PSNR, the highest SSIM and the lowest reconstruction errors.
更多查看译文
关键词
Image reconstruction,Magnetic resonance imaging,Deep learning,Radial sampling
AI 理解论文
溯源树
样例
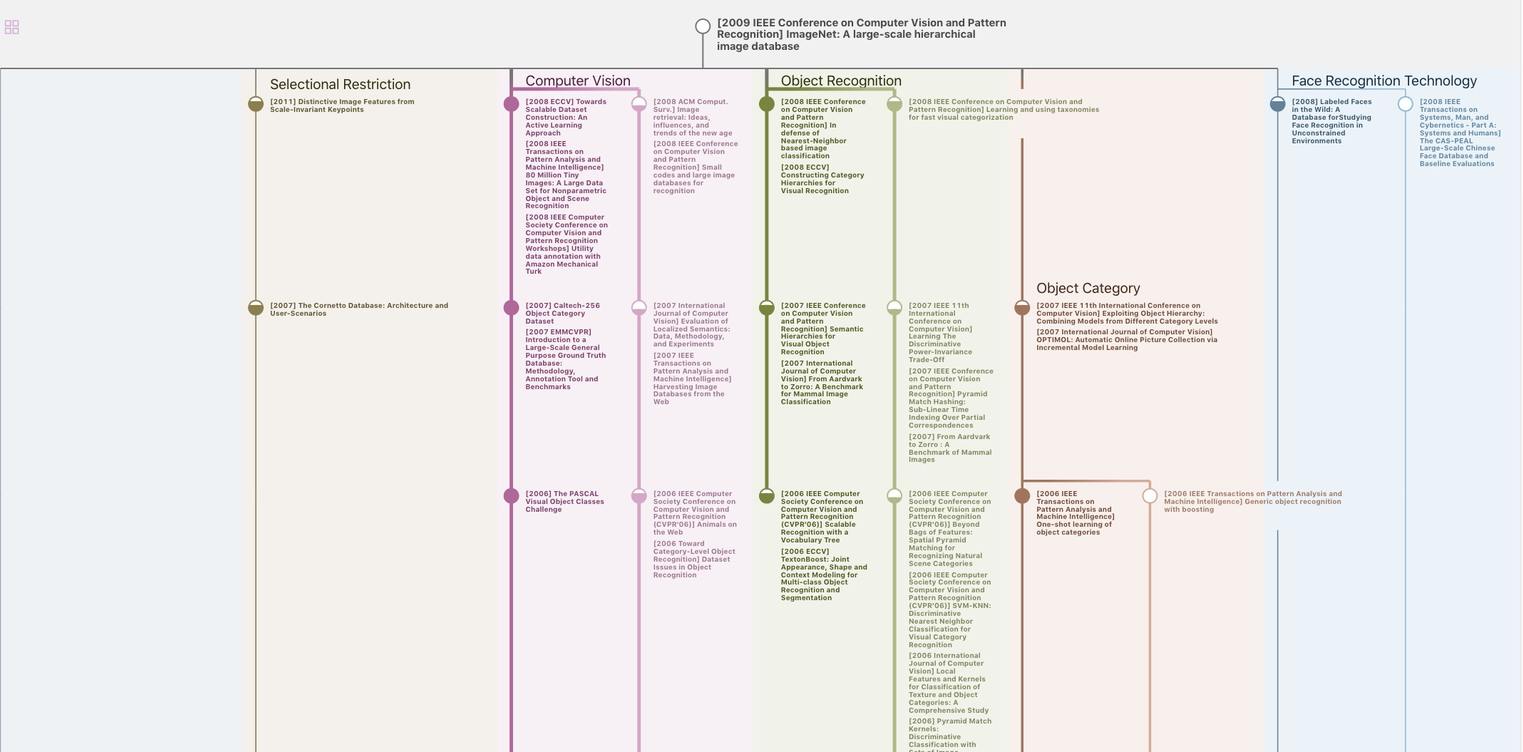
生成溯源树,研究论文发展脉络
Chat Paper
正在生成论文摘要