Operational greenhouse-gas emissions of deep learning in digital pathology: a modelling study
LANCET DIGITAL HEALTH(2024)
摘要
Background Deep learning is a promising way to improve health care. Image-processing medical disciplines, such as pathology, are expected to be transformed by deep learning. The first clinically applicable deep-learning diagnostic support tools are already available in cancer pathology, and their number is increasing. However, data on the environmental sustainability of these tools are scarce. We aimed to conduct an environmental-sustainability analysis of a theoretical implementation of deep learning in patient-care pathology.Methods For this modelling study, we first assembled and calculated relevant data and parameters of a digital-pathology workflow. Data were breast and prostate specimens from the university clinic at the Institute of Pathology of the Rheinisch-Westfalische Technische Hochschule Aachen (Aachen, Germany), for which commercially available deep learning was already available. Only specimens collected between Jan 1 and Dec 31, 2019 were used, to omit potential biases due to the COVID-19 pandemic. Our final selection was based on 2 representative weeks outside holidays, covering different types of specimens. To calculate carbon dioxide (CO2) or CO2 equivalent (CO2eq) emissions of deep learning in pathology, we gathered relevant data for exact numbers and sizes of whole-slide images (WSIs), which were generated by scanning histopathology samples of prostate and breast specimens. We also evaluated different data input scenarios (including all slide tiles, only tiles containing tissue, or only tiles containing regions of interest). To convert estimated energy consumption from kWh to CO2eq, we used the internet protocol address of the computational server and the Electricity Maps database to obtain information on the sources of the local electricity grid (ie, renewable vs non-renewable), and estimated the number of trees and proportion of the local and world's forests needed to sequester the CO2eq emissions. We calculated the computational requirements and CO2eq emissions of 30 deep-learning models that varied in task and size. The first scenario represented the use of one commercially available deep-learning model for one task in one case (1-task), the second scenario considered two deep-learning models for two tasks per case (2-task), the third scenario represented a future, potentially automated workflow that could handle 7 tasks per case (7-task), and the fourth scenario represented the use of a single potential, large, computer-vision model that could conduct multiple tasks (multitask). We also compared the performance (ie, accuracy) and CO2eq emissions of different deep-learning models for the classification of renal cell carcinoma on WSIs, also from Rheinisch-Westfalische Technische Hochschule Aachen. We also tested other approaches to reducing CO2eq emissions, including model pruning and an alternative method for histopathology analysis (pathomics). Findings The pathology database contained 35 552 specimens (237 179 slides), 6420 of which were prostate specimens (10 115 slides) and 11 801 of which were breast specimens (19 763 slides). We selected and subsequently digitised 140 slides from eight breast-cancer cases and 223 slides from five prostate-cancer cases. Applying large deep-learning models on all WSI tiles of prostate and breast pathology cases would result in yearly CO2eq emissions of 7 center dot 65 metric tons (t; 95% CI 7 center dot 62-7 center dot 68) with the use of a single deep-learning model per case; yearly CO2eq emissions were up to 100 center dot 56 t (100 center dot 21-100 center dot 99) with the use of seven deep-learning models per case. CO2eq emissions for different deep-learning model scenarios, data inputs, and deep-learning model sizes for all slides varied from 3 center dot 61 t (3 center dot 59-3 center dot 63) to 2795 center dot 30 t (1177 center dot 51-6482 center dot 13. For the estimated number of overall pathology cases worldwide, the yearly CO2eq emissions varied, reaching up to 16 megatons (Mt) of CO2eq, requiring up to 86 590 km2 (0 center dot 22%) of world forest to sequester the CO2eq emissions. Use of the 7-task scenario and small deep-learning models on slides containing tissue only could substantially reduce CO2eq emissions worldwide by up to 141 times (0 center dot 1 Mt, 95% CI 0 center dot 1-0 center dot 1). Considering the local environment in Aachen, Germany, the maximum CO2eq emission from the use of deep learning in digital pathology only would require 32 center dot 8% (95% CI 13 center dot 8-76 center dot 6) of the local forest to sequester the CO2eq emissions. A single pathomics run on a tissue could provide information that was comparable to or even better than the output of multitask deep-learning models, but with 147 times reduced CO2eq emissions.Interpretation Our findings suggest that widespread use of deep learning in pathology might have considerable global-warming potential. The medical community, policy decision makers, and the public should be aware of this potential and encourage the use of CO2eq emissions reduction strategies where possible. Copyright (c) 2023 The Author(s). Published by Elsevier Ltd. This is an Open Access article under the CC BY 4.0 license.
更多查看译文
AI 理解论文
溯源树
样例
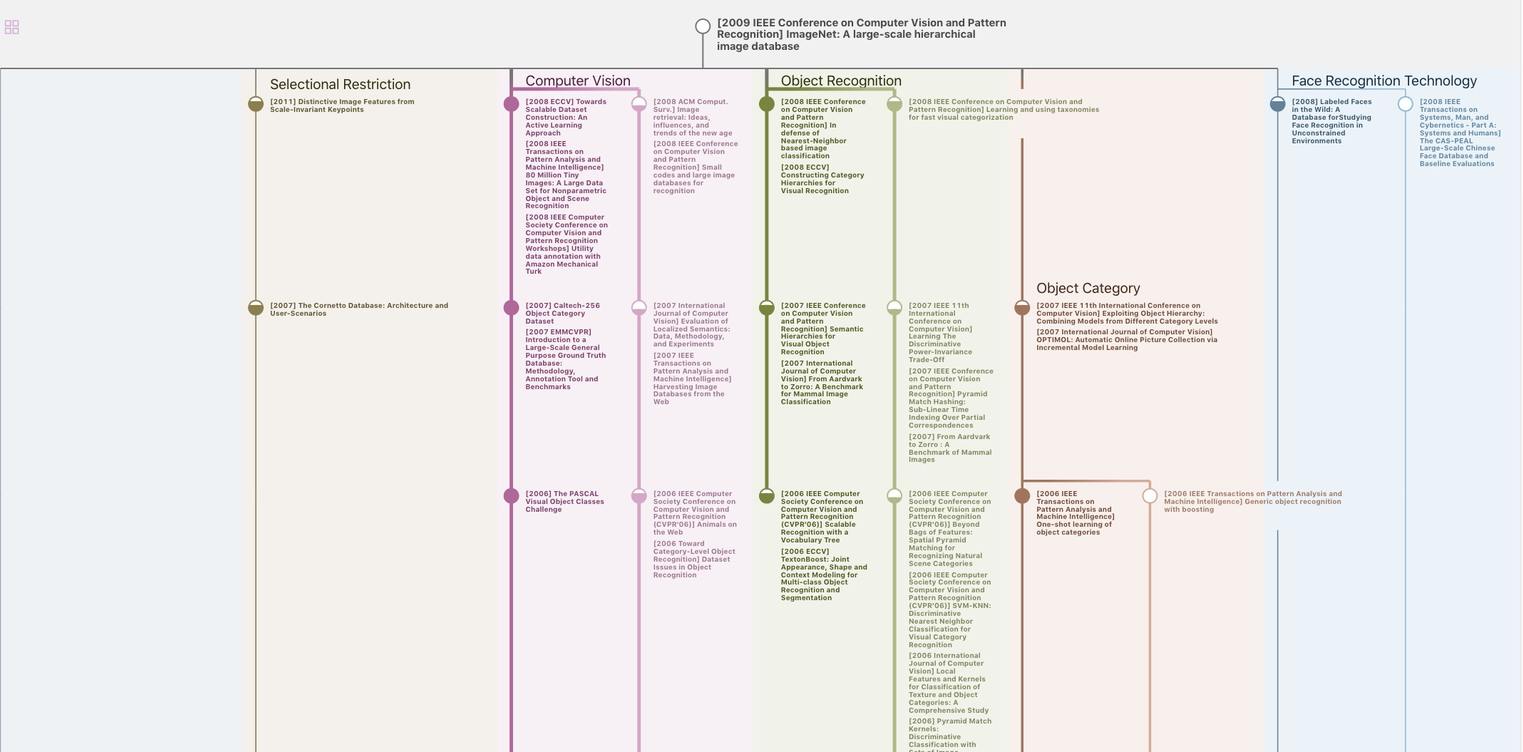
生成溯源树,研究论文发展脉络
Chat Paper
正在生成论文摘要