Image Recovery Matters: A Recovery-Extraction Framework for Robust Fetal Brain Extraction From MR Images.
IEEE journal of biomedical and health informatics(2024)
摘要
The extraction of the fetal brain from magnetic resonance (MR) images is a challenging task. In particular, fetal MR images suffer from different kinds of artifacts introduced during the image acquisition. Among those artifacts, intensity inhomogeneity is a common one affecting brain extraction. In this work, we propose a deep learning-based recovery-extraction framework for fetal brain extraction, which is particularly effective in handling fetal MR images with intensity inhomogeneity. Our framework involves two stages. First, the artifact-corrupted images are recovered with the proposed generative adversarial learning-based image recovery network with a novel region-of-darkness discriminator that enforces the network focusing on artifacts of the images. Second, we propose a brain extraction network for more effective fetal brain segmentation by strengthening the association between lower- and higher-level features as well as suppressing task-irrelevant features. Thanks to the proposed recovery-extraction strategy, our framework is able to accurately segment fetal brains from artifact-corrupted MR images. The experiments show that our framework achieves promising performance in both quantitative and qualitative evaluations, and outperforms state-of-the-art methods in both image recovery and fetal brain extraction.
更多查看译文
关键词
Fetal MRI,Brain extraction,Intensity inhomogeneity,Image recovery,Image segmentation
AI 理解论文
溯源树
样例
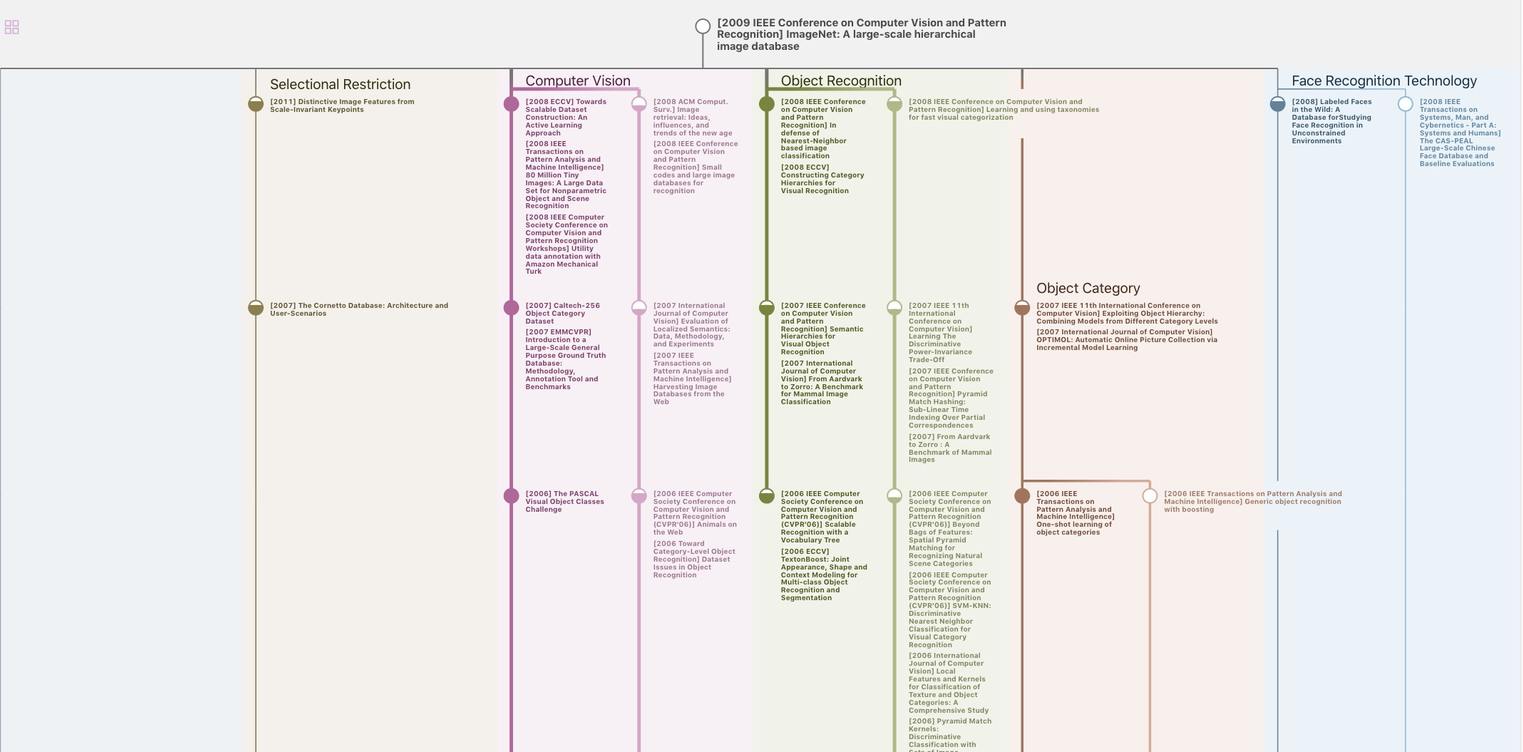
生成溯源树,研究论文发展脉络
Chat Paper
正在生成论文摘要