Multiscale Information Diffusion Prediction With Minimal Substitution Neural Network
IEEE TRANSACTIONS ON NEURAL NETWORKS AND LEARNING SYSTEMS(2023)
摘要
Information diffusion prediction is a complex task due to the dynamic of information substitution present in large social platforms, such as Weibo and Twitter. This task can be divided into two levels: the macroscopic popularity prediction and the microscopic information diffusion prediction (who is next), which share the essence of modeling the dynamic spread of information. While many researchers have focused on the internal influence of individual cascades, they often overlook other influential factors that affect information diffusion, such as competition and cooperation among information, the attractiveness of information to users, and the potential impact of content anticipation on further diffusion. To address this issue, we propose a multiscale information diffusion prediction with minimal substitution (MIDPMS) neural network. This model simultaneously enables macroscale popularity prediction and microscale diffusion prediction. Specifically, information diffusion is modeled as a substitution system among different information. First, the life cycle of content, user preferences, and potential content anticipation are considered in this system. Second, a minimal-substitution-theory-based neural network is first proposed to model this substitution system to facilitate joint training of macroscopic and microscopic diffusion prediction. Finally, extensive experiments are conducted on Weibo and Twitter datasets to validate the performance of our proposed model on multiscale tasks. The results confirmed that the proposed model performed well on both multiscale tasks on Weibo and Twitter.
更多查看译文
关键词
Cascade prediction,information diffusion,minimal substitution,multiscale,popularity prediction
AI 理解论文
溯源树
样例
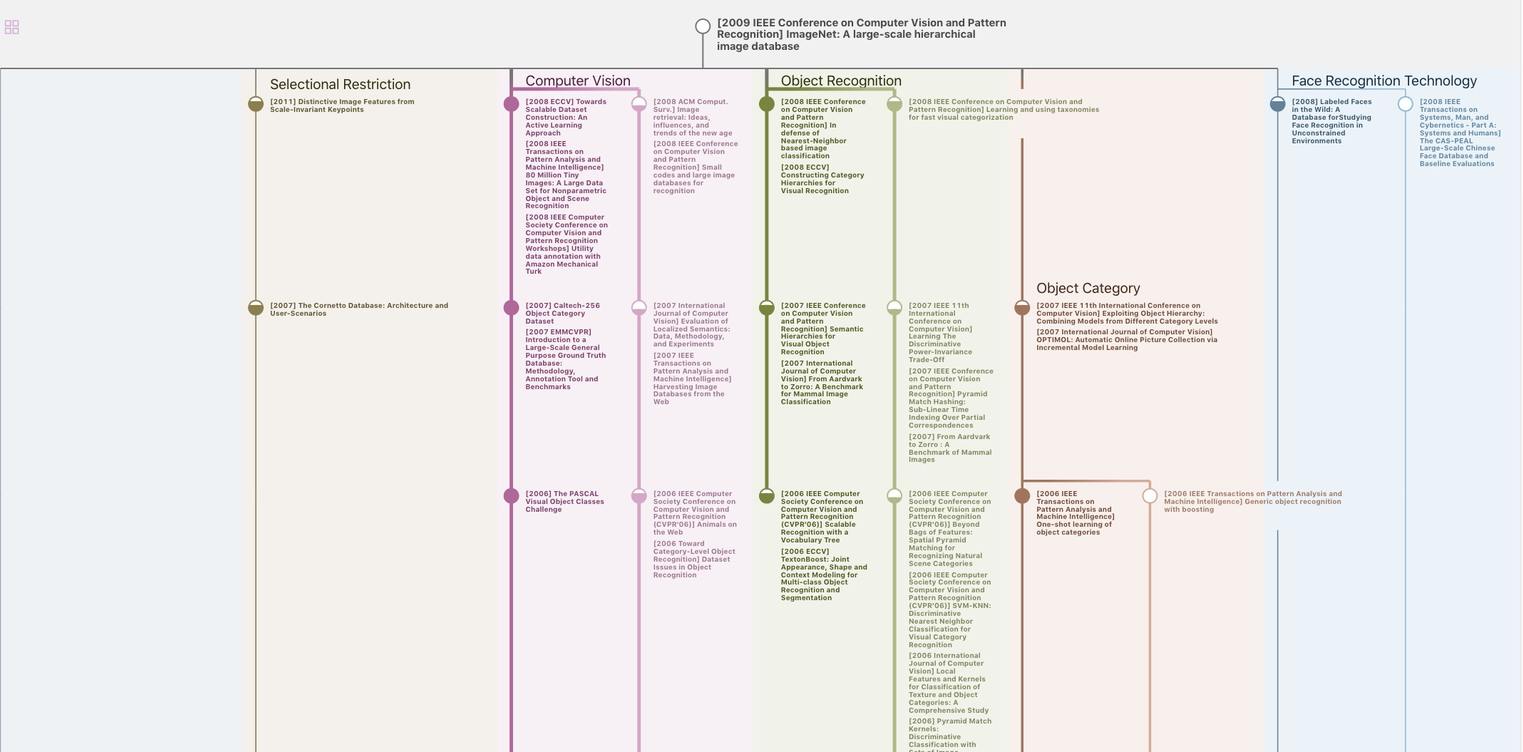
生成溯源树,研究论文发展脉络
Chat Paper
正在生成论文摘要