AdaReX: Cross-Domain, Adaptive and Explainable Recommender System
ANNUAL INTERNATIONAL ACM SIGIR CONFERENCE ON RESEARCH AND DEVELOPMENT IN INFORMATION RETRIEVAL IN THE ASIA PACIFIC REGION, SIGIR-AP 2023(2023)
摘要
Explainability is an inherent issue of recommender systems and has received a lot of attention recently. Generative explainable recommendation, which provides personalized explanations by generating textual rationales, is emerging as an effective solution. Despite promising, current methods face limitations in their reliance on dense training data, which hinders the generalizability of explainable recommender systems. Our work tackles a novel problem of cross-domain explainable recommendation aiming to extend the generalizability of explainable recommender systems. To solve this, we propose a novel approach that models aspects extracted from past reviews, to empower the explainable recommender systems by leveraging knowledge from other domains. Specifically, we propose AdaReX (Adaptive eXplainable Recommendation), to model auxiliary and target domains simultaneously. By performing specific tasks in respective domains and their interconnection via a discriminator model, AdaReX allows the aspect sequences to learn common knowledge across different domains and tasks. Furthermore, through our proposed optimization objective, the learning of aspect sequence is deeply cross-interacted with in-domain users and items' latent factors, enabling the enhanced sharing of knowledge between domains. Our extensive experiments on real datasets demonstrate that our approach not only generates better explanations and recommendations for sparse users but also improves performance for general users.
更多查看译文
关键词
Explainable Recommender System,Natural Language Generation
AI 理解论文
溯源树
样例
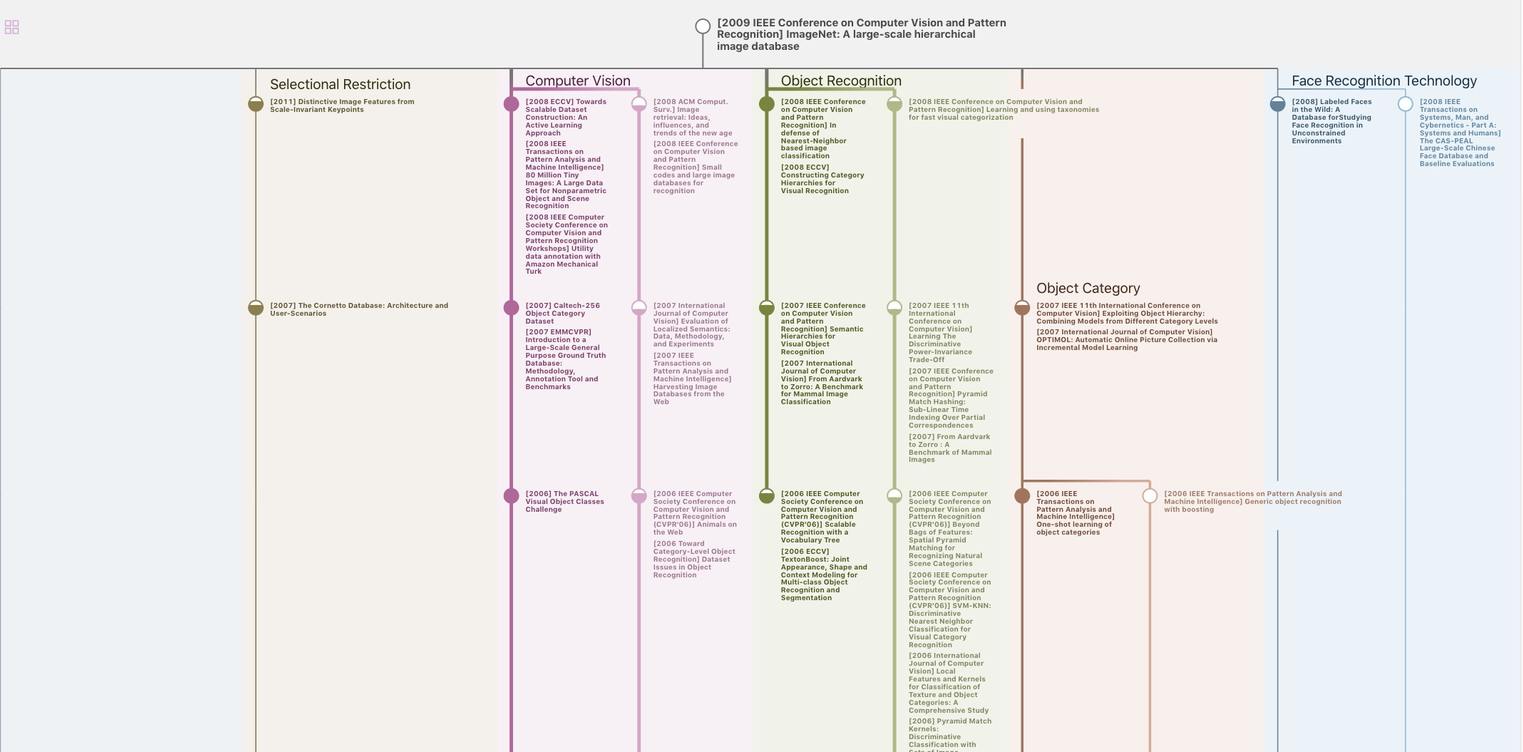
生成溯源树,研究论文发展脉络
Chat Paper
正在生成论文摘要