AGGDN: A Continuous Stochastic Predictive Model for Monitoring Sporadic Time Series on Graphs
NEURAL INFORMATION PROCESSING, ICONIP 2023, PT I(2024)
摘要
Monitoring data of real-world networked systems could be sparse and irregular due to node failures or packet loss, which makes it a challenge to model the continuous dynamics of system states. Representing a network as graph, we propose a deep learning model, Adversarial Graph-Gated Differential Network (AGGDN). To accurately capture the spatial-temporal interactions and extract hidden features from data, AGGDN introduces a novel module, dynDC-ODE, which empowers Ordinary Differential Equation (ODE) with learning-based Diffusion Convolution (DC) to effectively infer relations among nodes and parameterize continuous-time system dynamics over graph. It further incorporates a Stochastic Differential Equation (SDE) module and applies it over graph to efficiently capture the underlying uncertainty of the networked systems. Different from any single differential equation model, the ODE part also works as a control signal to modulate the SDE propagation. With the recurrent running of the two modules, AGGDN can serve as an accurate online predictive model that is effective for either monitoring or analyzing the real-world networked objects. In addition, we introduce a soft masking scheme to capture the effects of partial observations caused by the random missing of data from nodes. As training a model with SDE component could be challenging, Wasserstein adversarial training is exploited to fit the complicated distribution. Extensive results demonstrate that AGGDN significantly outperforms existing methods for online prediction.
更多查看译文
关键词
Graph Sequence Prediction,Sporadic Time Series,Continuous Model,Stochastic Model
AI 理解论文
溯源树
样例
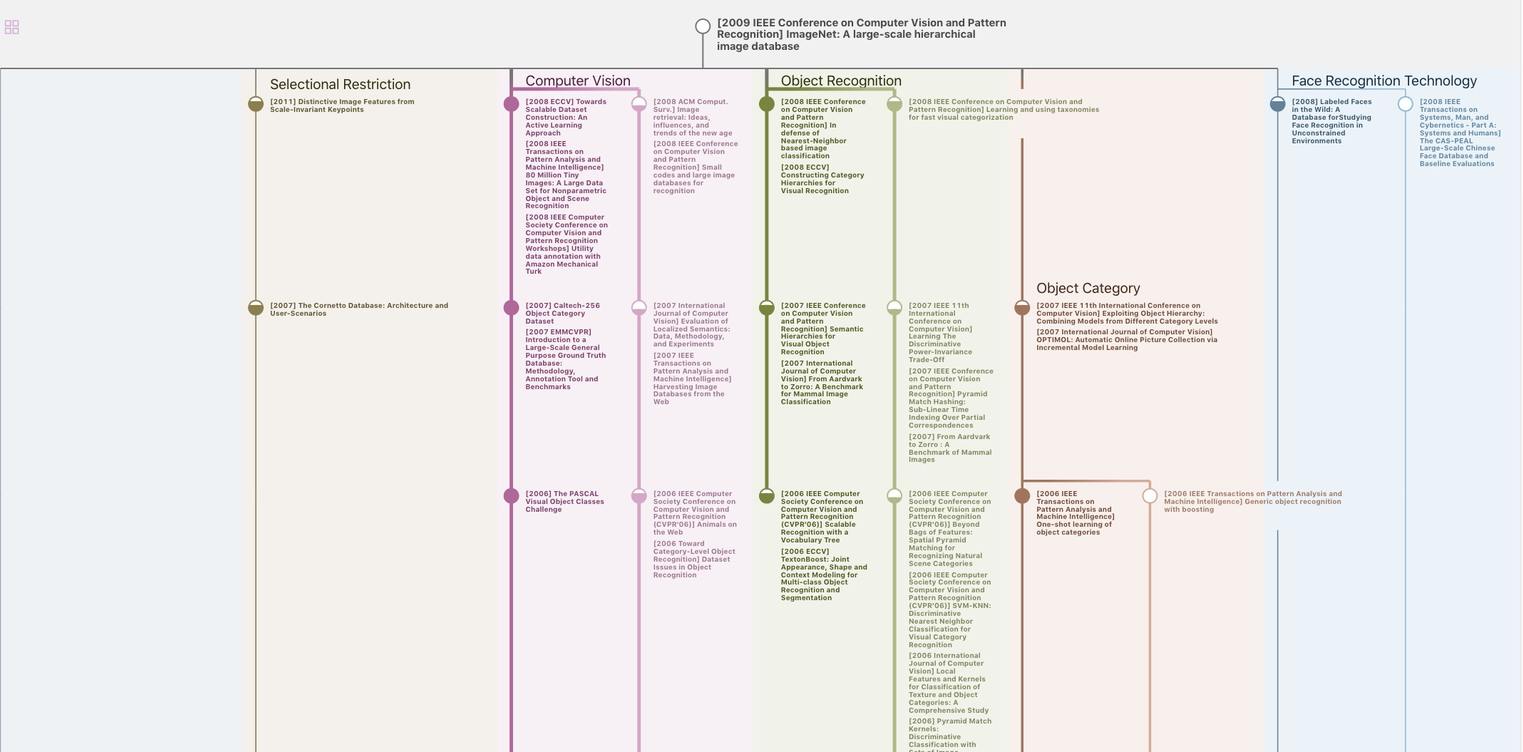
生成溯源树,研究论文发展脉络
Chat Paper
正在生成论文摘要