Quantum Autoencoder Frameworks for Network Anomaly Detection
NEURAL INFORMATION PROCESSING, ICONIP 2023, PT V(2024)
摘要
Detecting anomalous activities in network traffic is important for the timely identification of emerging cyber-attacks. Accurate analysis of the emerging patterns in the network traffic is critical to identify suspicious behaviors. This paper proposes novel quantum deep autoencoder-based anomaly detection frameworks for accurately detecting the security attacks that emerge in the network. In particular, we propose three frameworks, one by constructing several reconstruction error thresholds-based methods; second, a union of a quantum autoencoder and a one-class support vector machine-based method; and third a union of a quantum autoencoder and quantum random forest-based method. The quantum frameworks' effectiveness in accurately detecting the attacks is evaluated using a publicly available benchmark dataset. Our empirical evaluations demonstrate the improvements in accuracy and F1-score for the three frameworks.
更多查看译文
关键词
Quantum Machine Learning,Quantum Autoencoder,Quantum Anomaly Detection,Network Anomaly Detection
AI 理解论文
溯源树
样例
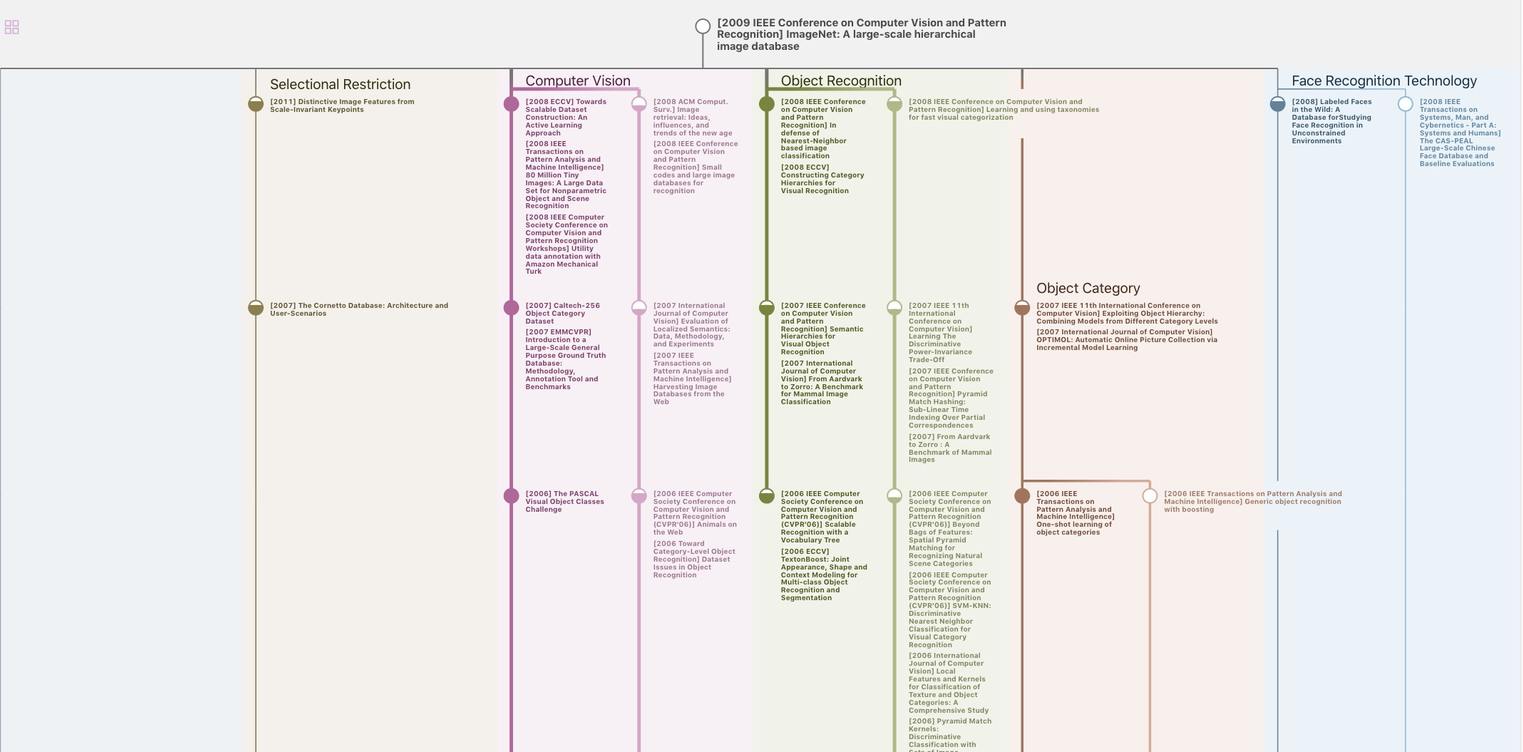
生成溯源树,研究论文发展脉络
Chat Paper
正在生成论文摘要