Empirical Analysis of Multi-label Classification on GitterCom Using BERT and ML Classifiers
NEURAL INFORMATION PROCESSING, ICONIP 2023, PT V(2024)
摘要
To maintain development consciousness, simplify project coordination, and prevent misinterpretation, communication is essential for software development teams. Instant private messaging, group chats, and sharing code are just a few of the capabilities that chat rooms provide to assist and meet the communication demands of software development teams. All of this is capacitated to happen in real-time. Consequently, chat rooms have gained popularity among developers. Gitter is one of these platforms that has gained popularity, and the conversations it contains may be a treasure trove of data for academics researching open-source software systems. This research made use of the GitterCom dataset, The largest collection of Gitter developer messages that have been carefully labelled and curated and perform multi-label classification for the 'Purpose' category in the dataset. An extensive empirical analysis is performed on 6 feature selection techniques, 14 machine learning classifiers, and BERT transformer layer architecture with layer-by-layer comparison. Consequently, we achieve proficient results through our research pipeline involving Extra Trees Classifier and Random Forest classifiers with AUC (OvR) median performance of 0.94 and 0.92 respectively. Furthermore, The research proposed research pipeline could be utilized for generic multi-label text classification on software developer forum text data.
更多查看译文
关键词
GitterCom,BERT analysis,Data Imbalance Methods,Feature Selection,Ensemble models,Sentence Embedding
AI 理解论文
溯源树
样例
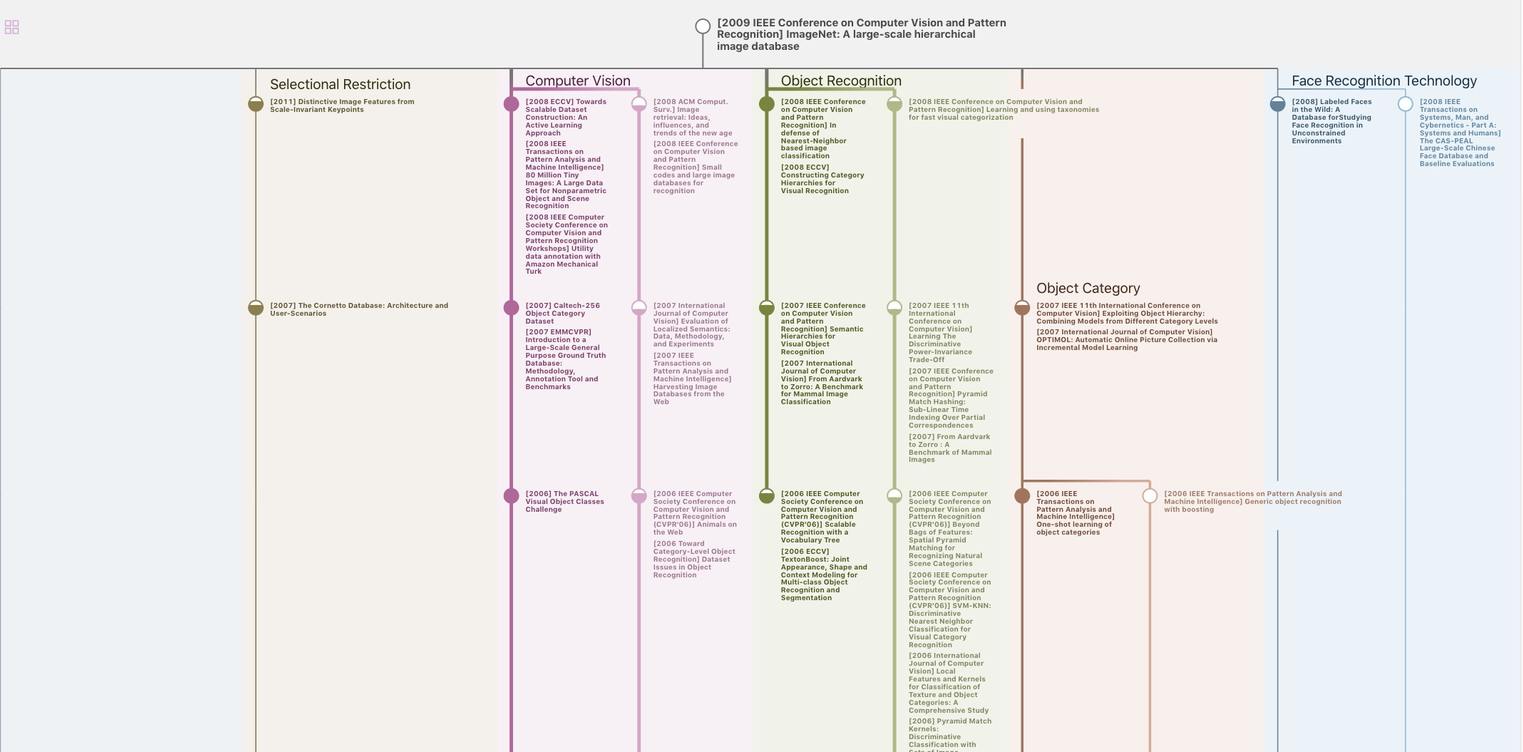
生成溯源树,研究论文发展脉络
Chat Paper
正在生成论文摘要