CKR-Calibrator: Convolution Kernel Robustness Evaluation and Calibration
NEURAL INFORMATION PROCESSING, ICONIP 2023, PT V(2024)
摘要
Recently, Convolution Neural Networks (CNN) have achieved excellent performance in some areas of computer vision, including face recognition, character recognition, and autonomous driving. However, there are still many CNN-based models that cannot be deployed in real-world scenarios due to poor robustness. In this paper, focusing on the classification task, we attempt to evaluate and optimize the robustness of CNN-based models from a new perspective: the convolution kernel. Inspired by the discovery that the root cause of the model decision error lies in the wrong response of the convolution kernel, we propose a convolution kernel robustness evaluation metric based on the distribution of convolution kernel responses. Then, we devise the Convolution Kernel Robustness Calibrator, termed as CKR-Calibrator, to optimize key but not robust convolution kernels. Extensive experiments demonstrate that CKR-Calibrator improves the accuracy of existing CNN classifiers by 1%-4% in clean datasets and 1%-5% in corrupt datasets, and improves the accuracy by about 2% over SOTA methods. The evaluation and calibration source code is open-sourced at https://github.com/cym- heu/CKR-Calibrator.
更多查看译文
关键词
Robustness,Convolution kernel,Evaluation,Calibration
AI 理解论文
溯源树
样例
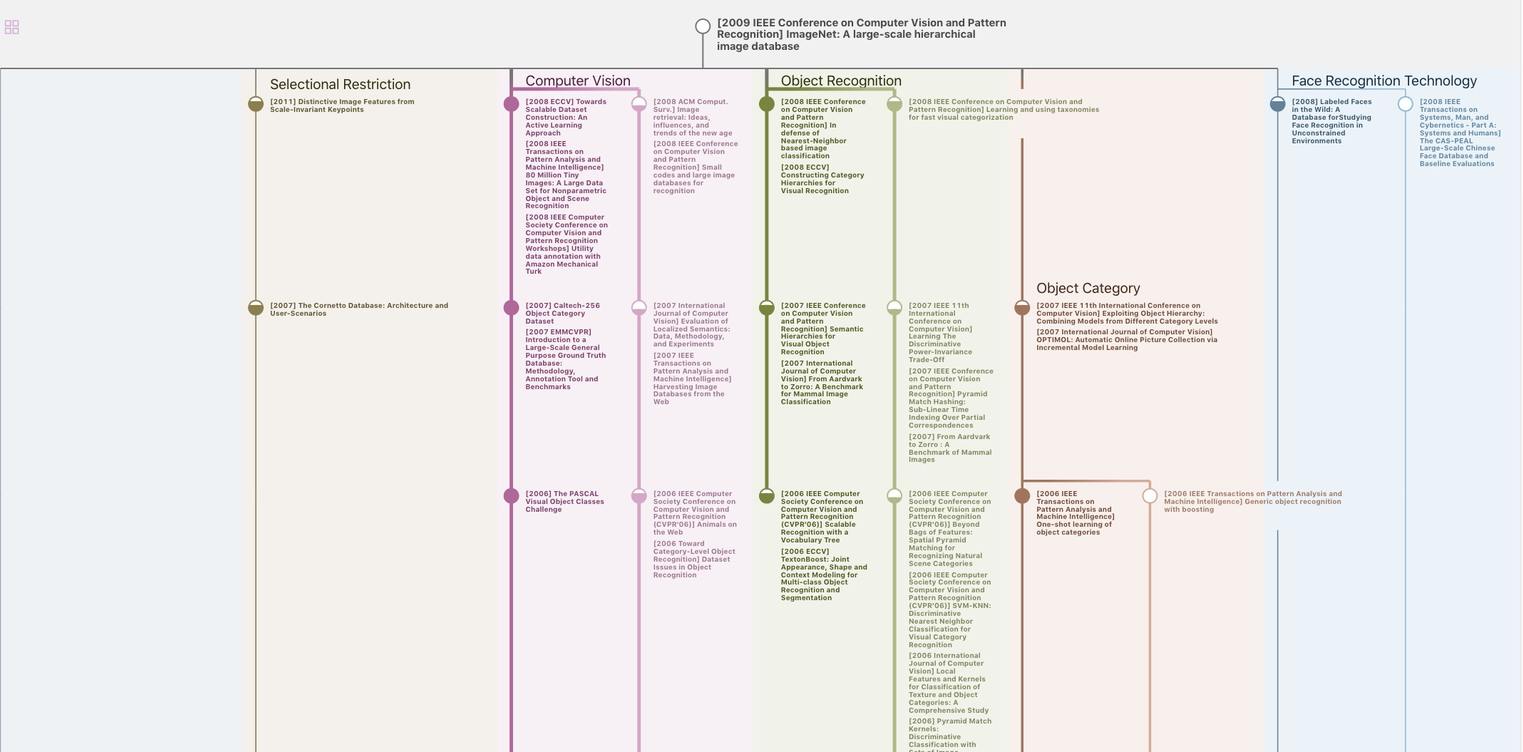
生成溯源树,研究论文发展脉络
Chat Paper
正在生成论文摘要