Latent Causal Dynamics Model for Model-Based Reinforcement Learning
NEURAL INFORMATION PROCESSING, ICONIP 2023, PT II(2024)
摘要
Learning an accurate dynamics model is the key task for model-based reinforcement learning (MBRL). Most existing MBRL methods learn the dynamics model over states. But in most cases, the relationships among states are complex because the states are affected by the interaction of various factors in the environment. Recently some works are proposed to learn the dynamics model on latent representations space. But the learned model is dense and may contain spurious associations between latent representations. To deal with these problems, we introduce a latent causal dynamics model over latent representations and provide a learning method for MBRL. Specifically, we first learn the latent representations from the observed state space. Second, we learn a latent causal dynamics model among latent representations by a causal discovery method. Finally, the latent causal dynamics model is used to aid policy learning. The above steps are iterative to update the unified loss function until convergence. Experimental results on four tasks show that the performance of our proposed method benefits from the causality and the learned latent representations.
更多查看译文
关键词
Reinforcement learning,Causal discovery,Latent representation,Dynamics model
AI 理解论文
溯源树
样例
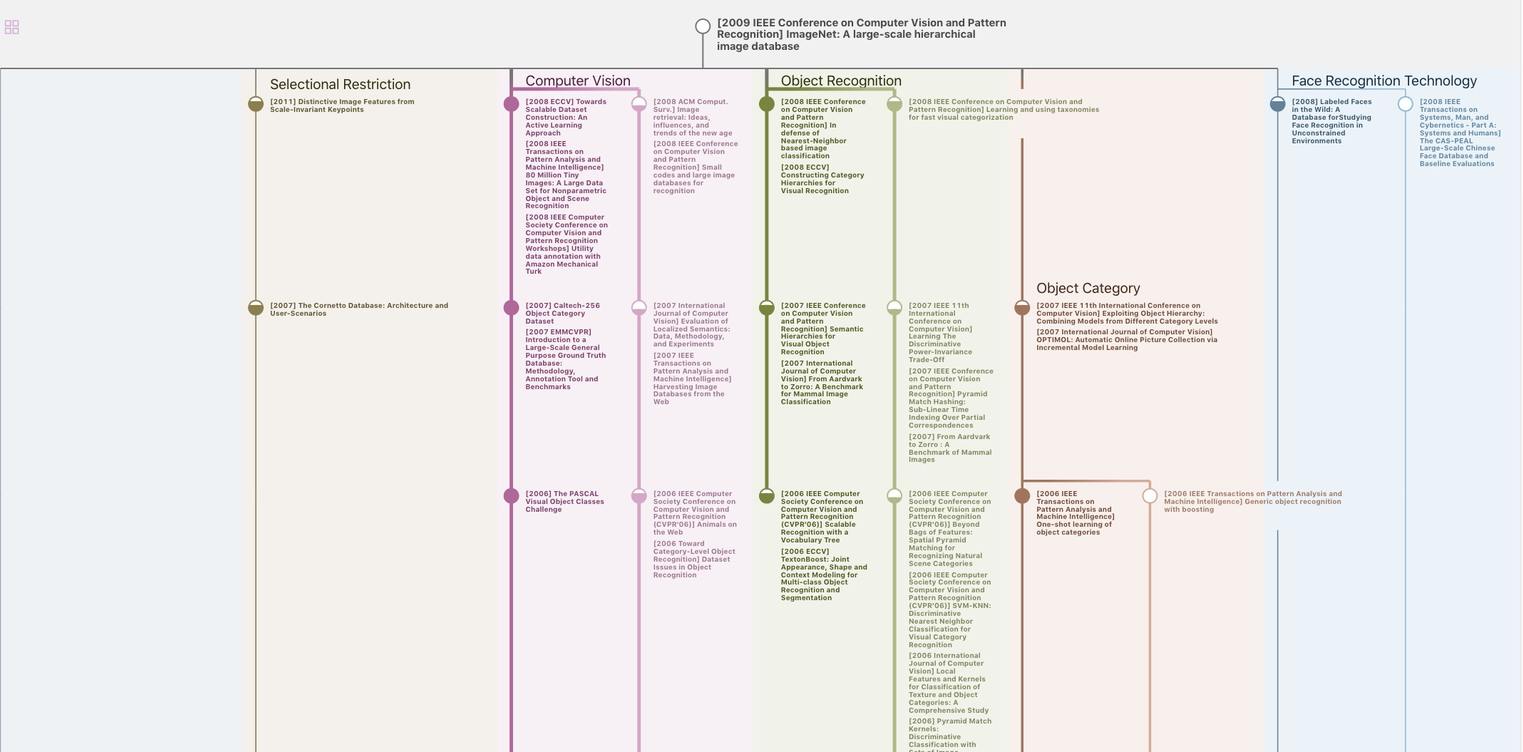
生成溯源树,研究论文发展脉络
Chat Paper
正在生成论文摘要