Enhancing Heterogeneous Graph Contrastive Learning with Strongly Correlated Subgraphs
NEURAL INFORMATION PROCESSING, ICONIP 2023, PT VI(2024)
摘要
Graph contrastive learning maximizes the mutual information between the embedding representations of the same data instances in different augmented views of a graph, obtaining feature representations for graph data in an unsupervised manner without the need for manual labeling. Most existing node-level graph contrastive learning models only consider embeddings of the same node in different views as positive sample pairs, ignoring rich inherent neighboring relation and resulting in certain contrastive information loss. To address this issue, we propose a heterogeneous graph contrastive learning model that incorporates strongly correlated subgraph features. We design a contrastive learning framework suitable for heterogeneous graphs and introduce high-level neighborhood information during the contrasting process. Specifically, our model selects a strongly correlated subgraph for each target node in the heterogeneous graph based on both topological structure information and node attribute feature information. In the calculation of contrastive loss, we perform feature shifting operations on positive and negative samples based on subgraph encoding to enhance the model's ability to discriminate between approximate samples. We conduct node classification and ablation experiments on multiple public heterogeneous datasets and the results verify the effectiveness of the research contributions of our model.
更多查看译文
关键词
Graph representation learning,Contrastive learning,Correlated subgraph
AI 理解论文
溯源树
样例
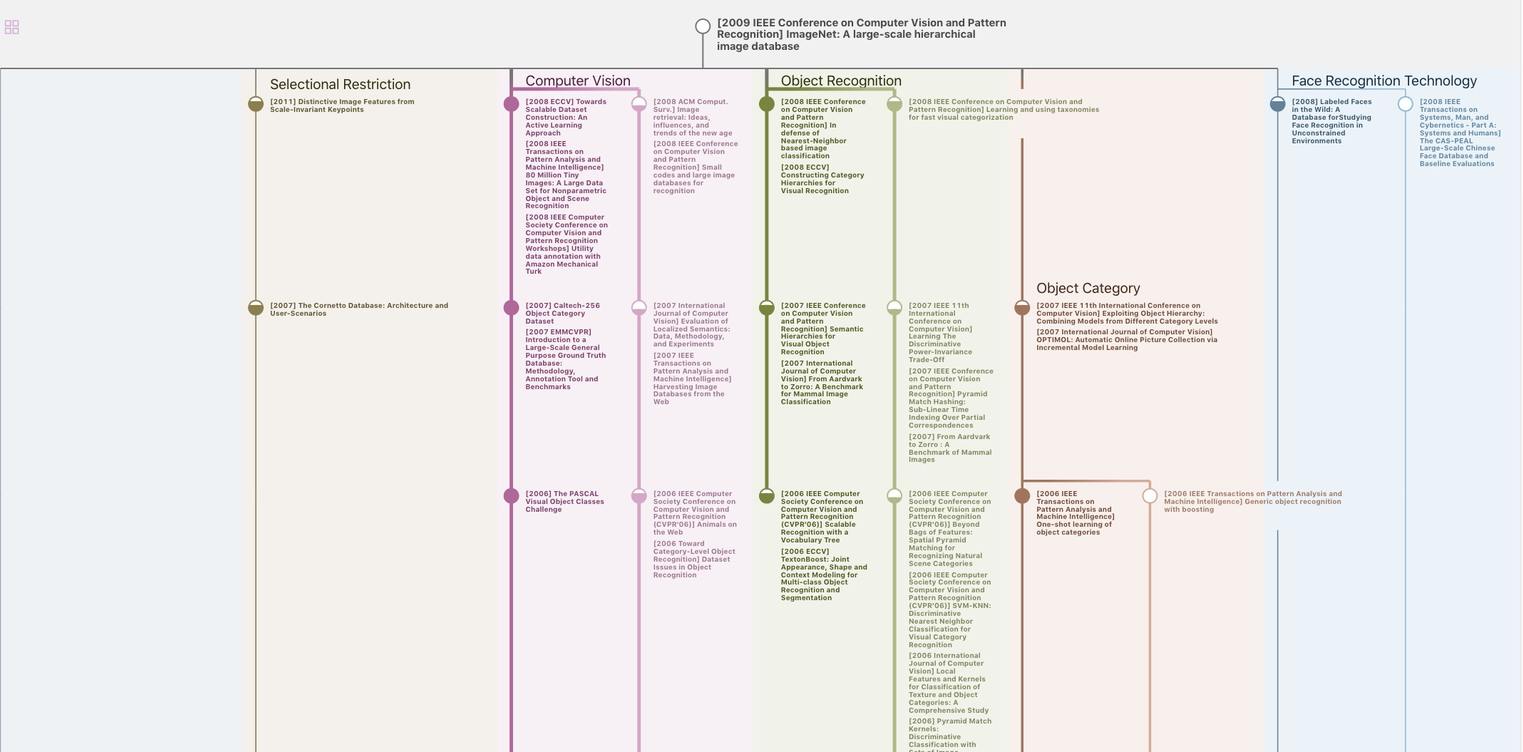
生成溯源树,研究论文发展脉络
Chat Paper
正在生成论文摘要