MIC: An Effective Defense Against Word-Level Textual Backdoor Attacks
NEURAL INFORMATION PROCESSING, ICONIP 2023, PT VI(2024)
摘要
Backdoor attacks, which manipulate model output, have garnered significant attention from researchers. However, some existing word-level backdoor attack methods in NLP models are difficult to defend effectively due to their concealment and diversity. These covert attacks use two words that appear similar to the naked eye but will be mapped to different word vectors by the NLP model as a way of bypassing existing defenses. To address this issue, we propose incorporating triple metric learning into the standard training phase of NLP models to defend against existing word-level backdoor attacks. Specifically, metric learning is used to minimize the distance between vectors of similar words while maximizing the distance between them and vectors of other words. Additionally, given that metric learning may reduce a model's sensitivity to semantic changes caused by subtle perturbations, we added contrastive learning after the model's standard training. Experimental results demonstrate that our method performs well against the two most stealthy existing word-level backdoor attacks.
更多查看译文
关键词
Defense Against Textual Backdoor Attacks,Triple Metric Learning,Contrastive Learning,Natural Language Processing (NLP) Models
AI 理解论文
溯源树
样例
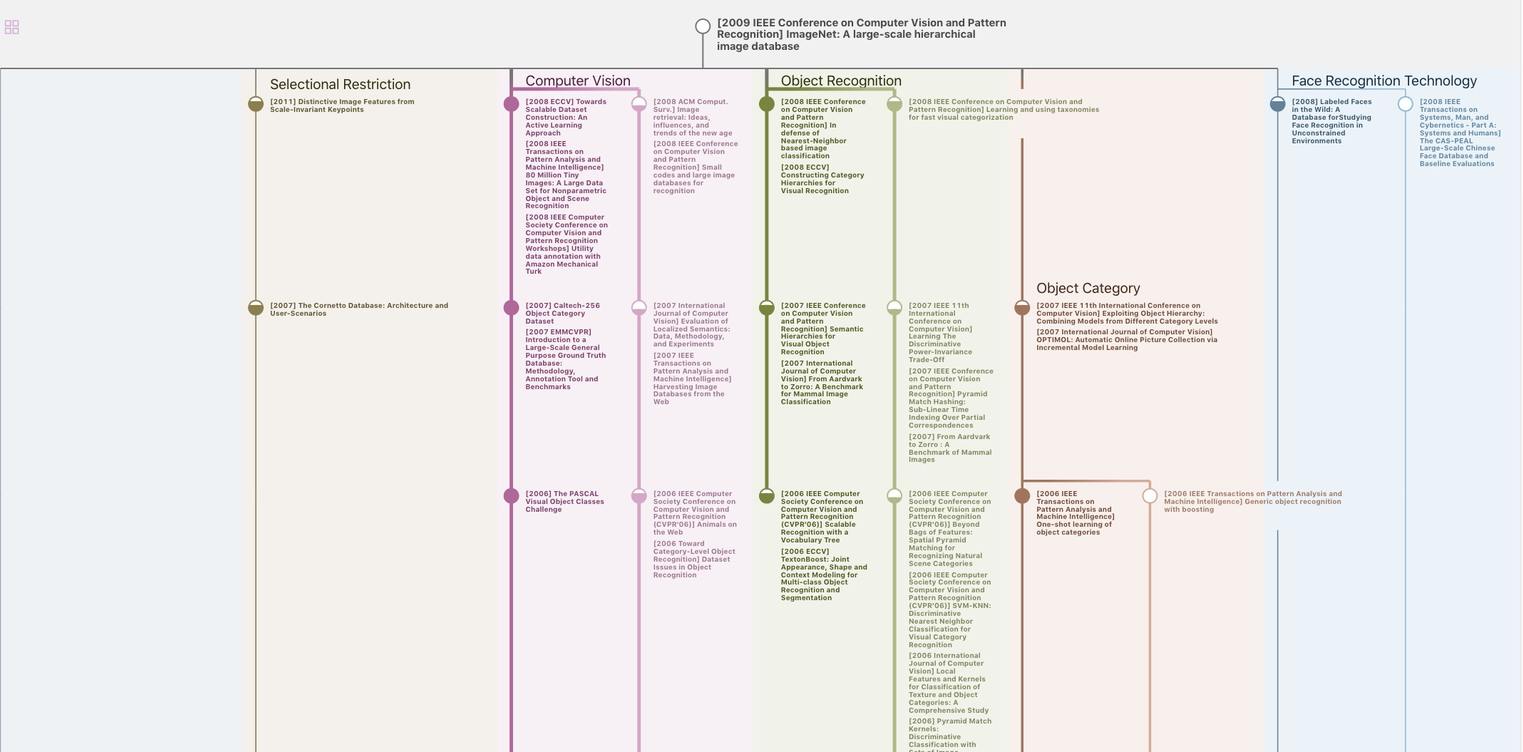
生成溯源树,研究论文发展脉络
Chat Paper
正在生成论文摘要