Theoretical Analysis of Gradient-Zhang Neural Network for Time-Varying Equations and Improved Method for Linear Equations
NEURAL INFORMATION PROCESSING, ICONIP 2023, PT I(2024)
摘要
Solving time-varying equations is fundamental in science and engineering. This paper aims to find a fast-converging and high-precision method for solving time-varying equations. We combine two classes of feedback neural networks, i.e., gradient neural network (GNN) and Zhang neural network (ZNN), to construct a continuous gradient-Zhang neural network (GZNN) model. Our research shows that GZNN has the advantages of high convergence precision of ZNN and fast convergence speed of GNN in certain cases, i.e., all the eigenvalues of Jacobian matrix of the time-varying equations multiplied by its transpose are larger than 1. Furthermore, we conduct the different detailed mathematical proof and theoretical analysis to establish the stability and convergence of the GZNN model. Additionally, we discretize the GZNN model by utilizing time discretization formulas (i.e., Euler and Taylor-Zhang discretization formulas), to construct corresponding discrete GZNN algorithms for solving discrete time-varying problems. Different discretization formulas can construct discrete algorithms with varying precision. As the number of time sampling instants increases, the precision of discrete algorithms can be further improved. Furthermore, we improve the matrix inverse operation in the GZNN model and develop inverse-free GZNN algorithms to solve linear problems, effectively reducing their time complexity. Finally, numerical experiments are conducted to validate the feasibility of GZNN model and the corresponding discrete algorithms in solving time-varying equations, as well as the efficiency of the inverse-free method in solving linear equations.
更多查看译文
关键词
Gradient-Zhang Neural Network,Time-Varying Equations,Discrete Algorithms
AI 理解论文
溯源树
样例
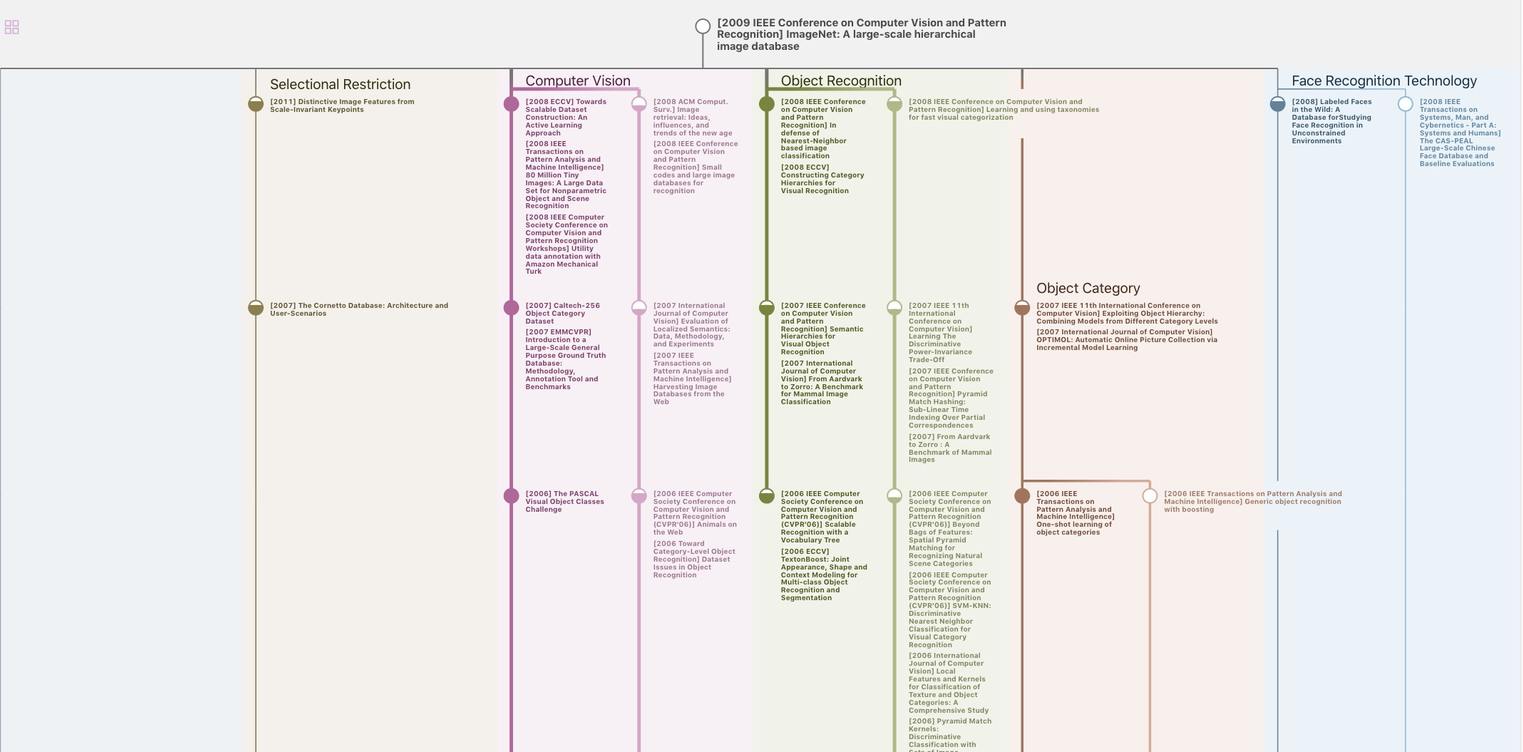
生成溯源树,研究论文发展脉络
Chat Paper
正在生成论文摘要