Pyramid Swin Transformer for Multi-task: Expanding to More Computer Vision Tasks
ADVANCED CONCEPTS FOR INTELLIGENT VISION SYSTEMS, ACIVS 2023(2023)
摘要
We presented the Pyramid Swin Transformer, a versatile and efficient architecture tailored for object detection and image classification. This time we applied it to a wider range of tasks, such as object detection, image classification, semantic segmentation, and video recognition tasks. Our architecture adeptly captures local and global contextual information by employing more shift window operations and integrating diverse window sizes. The Pyramid Swin Transformer for Multi-task is structured in four stages, each consisting of layers with varying window sizes, facilitating a robust hierarchical representation. Different numbers of layers with distinct windows and window sizes are utilized at the same scale. Our architecture has been extensively evaluated on multiple benchmarks, including achieving 85.4% top-1 accuracy on ImageNet for image classification, 51.6 AP(box) with Mask R-CNN and 54.3 AP(box) with Cascade Mask R-CNN on COCO for object detection, 49.0 mIoU on ADE20K for semantic segmentation, and 83.4% top-1 accuracy on Kinetics-400 for video recognition. The Pyramid Swin Transformer for Multi-task outperforms state-of-the-art models in all tasks, demonstrating its effectiveness, adaptability, and scalability across various vision tasks. This breakthrough in multi-task learning architecture opens the door to new research and applications in the field.
更多查看译文
关键词
Computer Vision,Transformer Vision,Swin Transformer
AI 理解论文
溯源树
样例
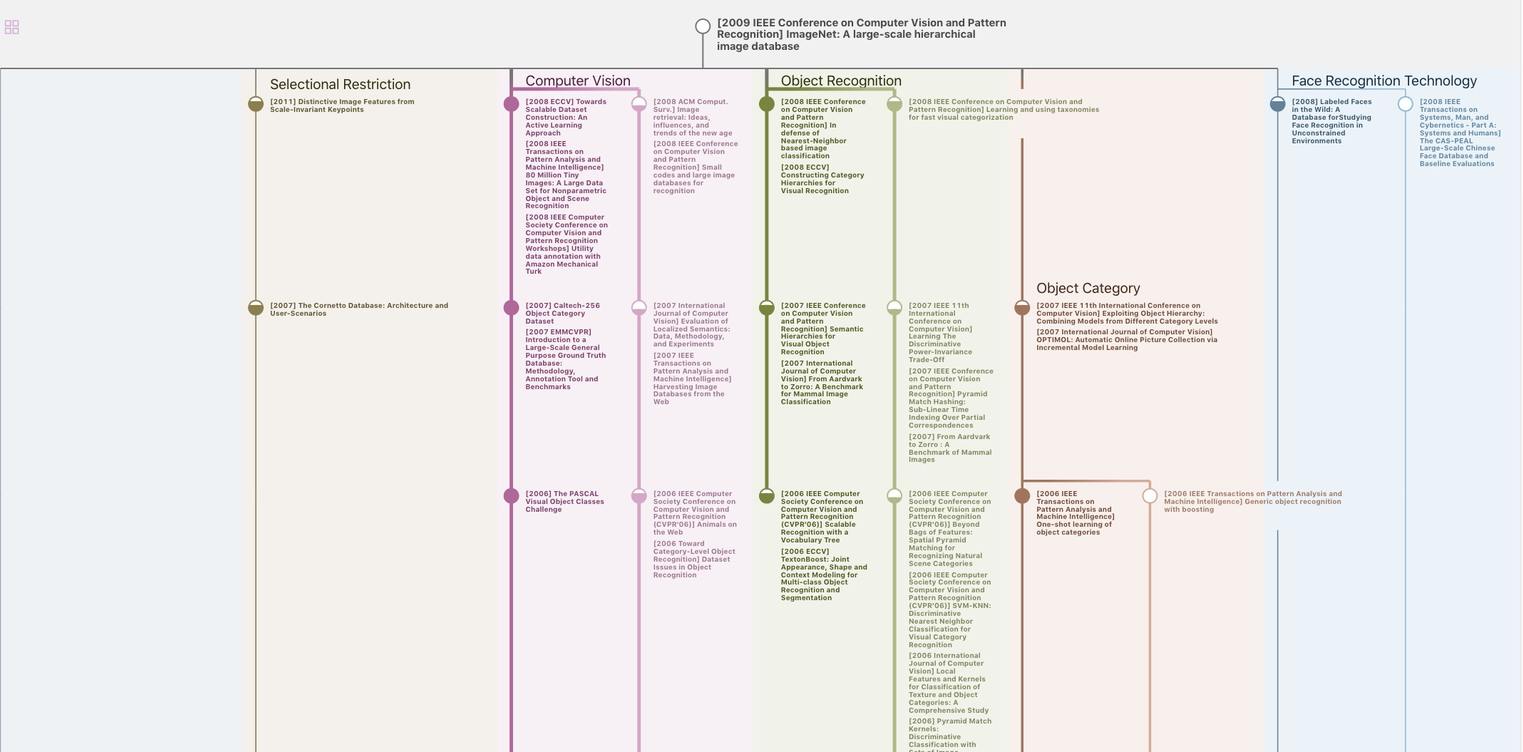
生成溯源树,研究论文发展脉络
Chat Paper
正在生成论文摘要