Privacy through Diffusion: A White-listing Approach to Sensor Data Anonymization
PROCEEDINGS OF THE 5TH WORKSHOP ON CPS & IOT SECURITY AND PRIVACY, CPSIOTSEC 2023(2023)
摘要
Generative models have shown great promise in synthesizing high-quality time-series data that resemble the sensor data generated by mobile and IoT devices, but do not reveal the user's private attributes. These synthesized data can be treated as the obfuscated version of the sensor data and sent to downstream applications. However, existing obfuscation techniques that rely on generative models require the user to enumerate all inferences they deem intrusive. This black-listing approach would inevitably result in privacy loss if the definition of intrusive inferences changes after releasing the obfuscated data. In this work, we propose a white-listed approach to sensor data obfuscation based on a guided denoising diffusion model and a surrogate model for the desired inference. We evaluate this obfuscation model on a human activity recognition dataset and show that the proposed obfuscation model provides an acceptable privacy-utility trade-off, without assuming knowledge of the private attributes.
更多查看译文
关键词
Privacy-utility trade-off,deep generative models
AI 理解论文
溯源树
样例
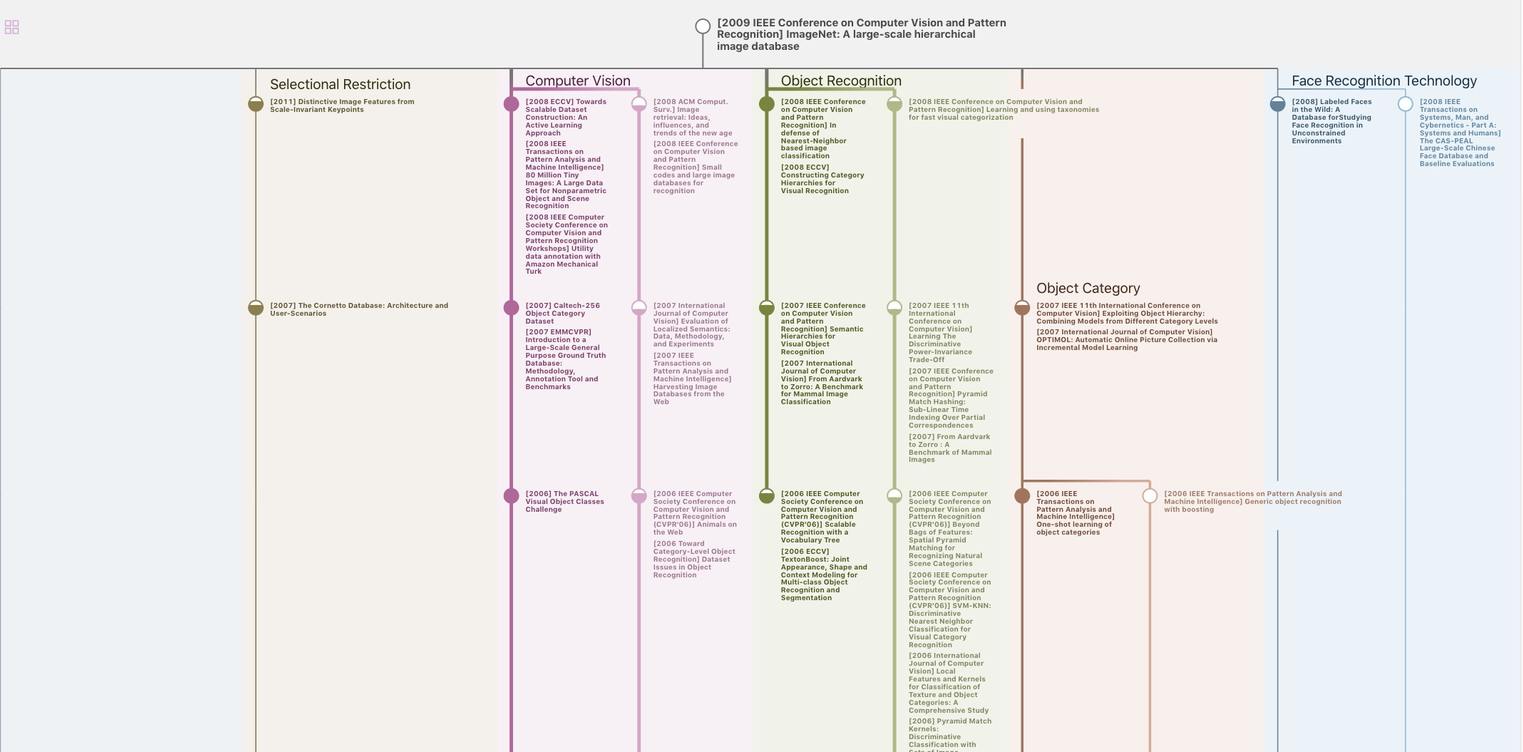
生成溯源树,研究论文发展脉络
Chat Paper
正在生成论文摘要