Probabilistic Logic Programming Semantics For Procedural Content Generation.
AIIDE '23: Proceedings of the Nineteenth AAAI Conference on Artificial Intelligence and Interactive Digital Entertainment(2023)
摘要
Research in procedural content generation (PCG) has recently heralded two major methodologies: machine learning (PCGML) and declarative programming. The former shows promise by automating the specification of quality criteria through latent patterns in data, while the latter offers significant advantages for authorial control. In this paper, we propose the use of probabilistic logic as a unifying framework that combines the benefits of both methodologies. We propose a Bayesian formalization of content generators as probability distributions and show how common PCG tasks map naturally to operations on the distribution. Further, through a series of experiments with maze generation, we demonstrate how probabilistic logic semantics allows us to leverage the authorial control of declarative programming and the flexibility of learning from data.
更多查看译文
关键词
probabilistic logic programming semantics,procedural content generation
AI 理解论文
溯源树
样例
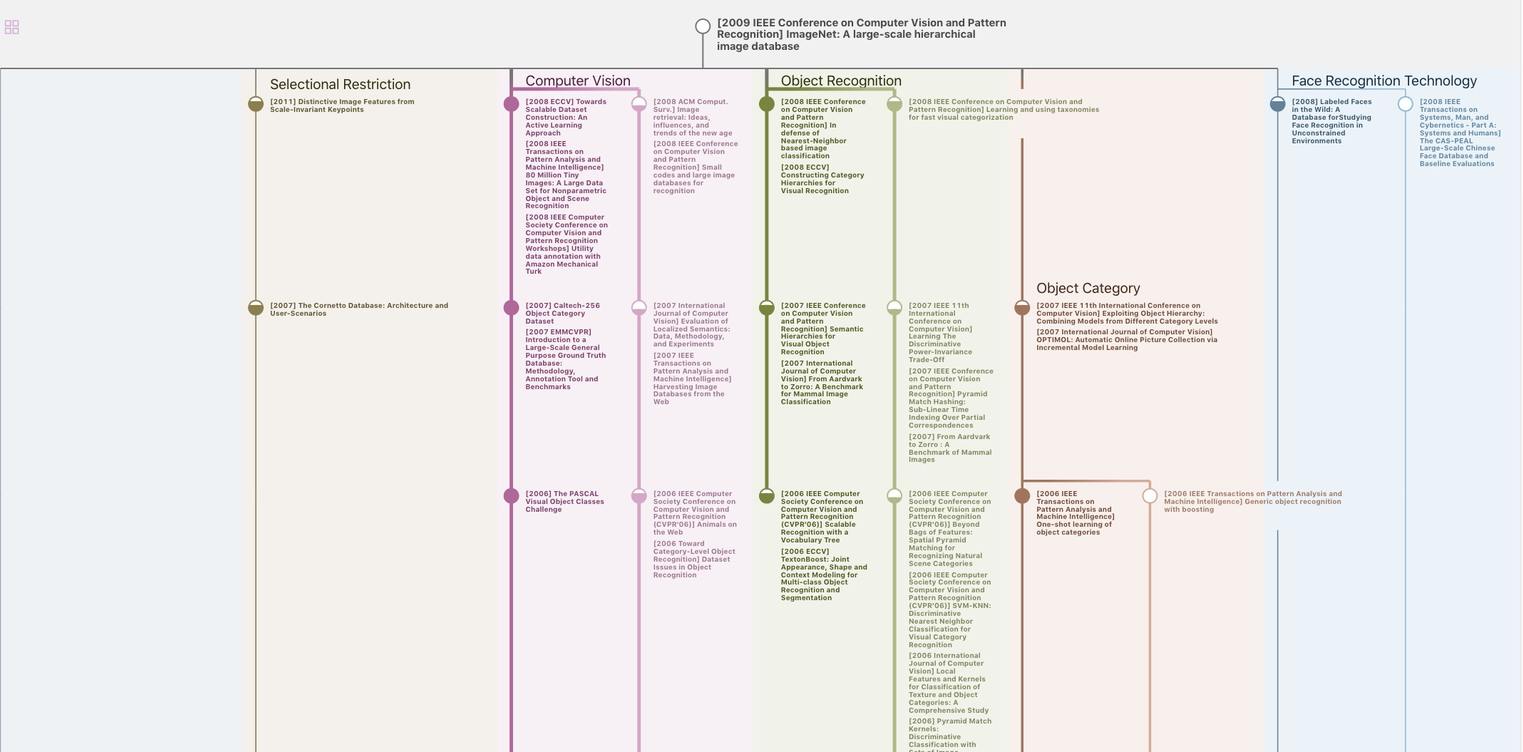
生成溯源树,研究论文发展脉络
Chat Paper
正在生成论文摘要