FLIBD: A Federated Learning-Based IoT Big Data Management Approach for Privacy-Preserving over Apache Spark with FATE
ELECTRONICS(2023)
摘要
In this study, we introduce FLIBD, a novel strategy for managing Internet of Things (IoT) Big Data, intricately designed to ensure privacy preservation across extensive system networks. By utilising Federated Learning (FL), Apache Spark, and Federated AI Technology Enabler (FATE), we skilfully investigated the complicated area of IoT data management while simultaneously reinforcing privacy across broad network configurations. Our FLIBD architecture was thoughtfully designed to safeguard data and model privacy through a synergistic integration of distributed model training and secure model consolidation. Notably, we delved into an in-depth examination of adversarial activities within federated learning contexts. The Federated Adversarial Attack for Multi-Task Learning (FAAMT) was thoroughly assessed, unmasking its proficiency in showcasing and exploiting vulnerabilities across various federated learning approaches. Moreover, we offer an incisive evaluation of numerous federated learning defence mechanisms, including Romoa and RFA, in the scope of the FAAMT. Utilising well-defined evaluation metrics and analytical processes, our study demonstrated a resilient framework suitable for managing IoT Big Data across widespread deployments, while concurrently presenting a solid contribution to the progression and discussion surrounding defensive methodologies within the federated learning and IoT areas.
更多查看译文
关键词
federated learning,privacy preserving,data poisoning,Big Data systems,Apache Spark,FATE,IoT data management
AI 理解论文
溯源树
样例
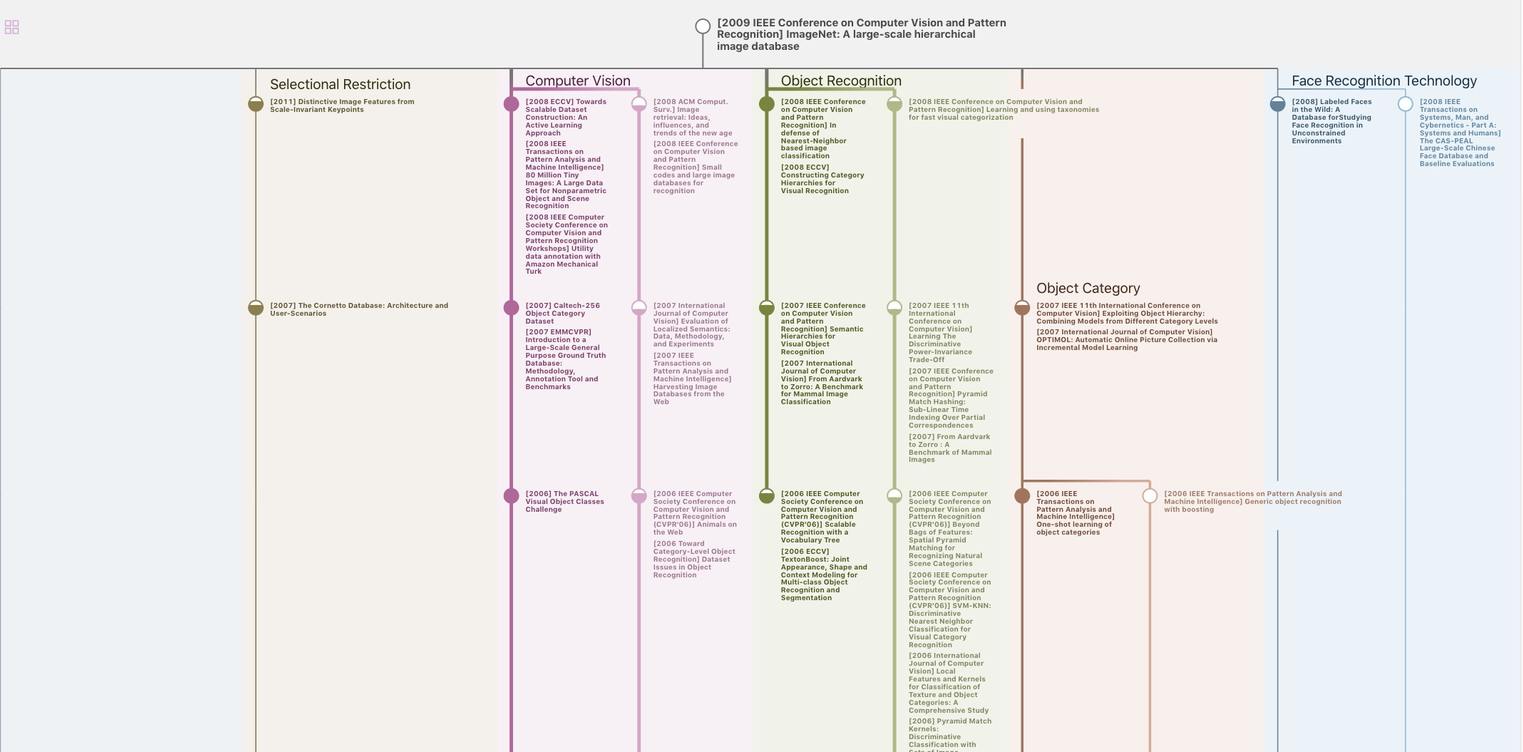
生成溯源树,研究论文发展脉络
Chat Paper
正在生成论文摘要