Machine Learning-Based Approach to Wind Turbine Wake Prediction under Yawed Conditions
JOURNAL OF MARINE SCIENCE AND ENGINEERING(2023)
摘要
As wind energy continues to be a crucial part of sustainable power generation, the need for precise and efficient modeling of wind turbines, especially under yawed conditions, becomes increasingly significant. Addressing this, the current study introduces a machine learning-based symbolic regression approach for elucidating wake dynamics. Utilizing WindSE's actuator line method (ALM) and Large Eddy Simulation (LES), we model an NREL 5-MW wind turbine under yaw conditions ranging from no yaw to 40 degrees. Leveraging a hold-out validation strategy, the model achieves robust hyper-parameter optimization, resulting in high predictive accuracy. While the model demonstrates remarkable precision in predicting wake deflection and velocity deficit at both the wake center and hub height, it shows a slight deviation at low downstream distances, which is less critical to our focus on large wind farm design. Nonetheless, our approach sets the stage for advancements in academic research and practical applications in the wind energy sector by providing an accurate and computationally efficient tool for wind farm optimization. This study establishes a new standard, filling a significant gap in the literature on the application of machine learning-based wake models for wind turbine yaw wake prediction.
更多查看译文
关键词
yaw wake prediction,NREL 5-MW wind turbine,wind energy optimization,symbolic regression,AI,wind turbine wake deflection,wind turbine velocity deficit,wind farm design studies,WindSE,machine learning
AI 理解论文
溯源树
样例
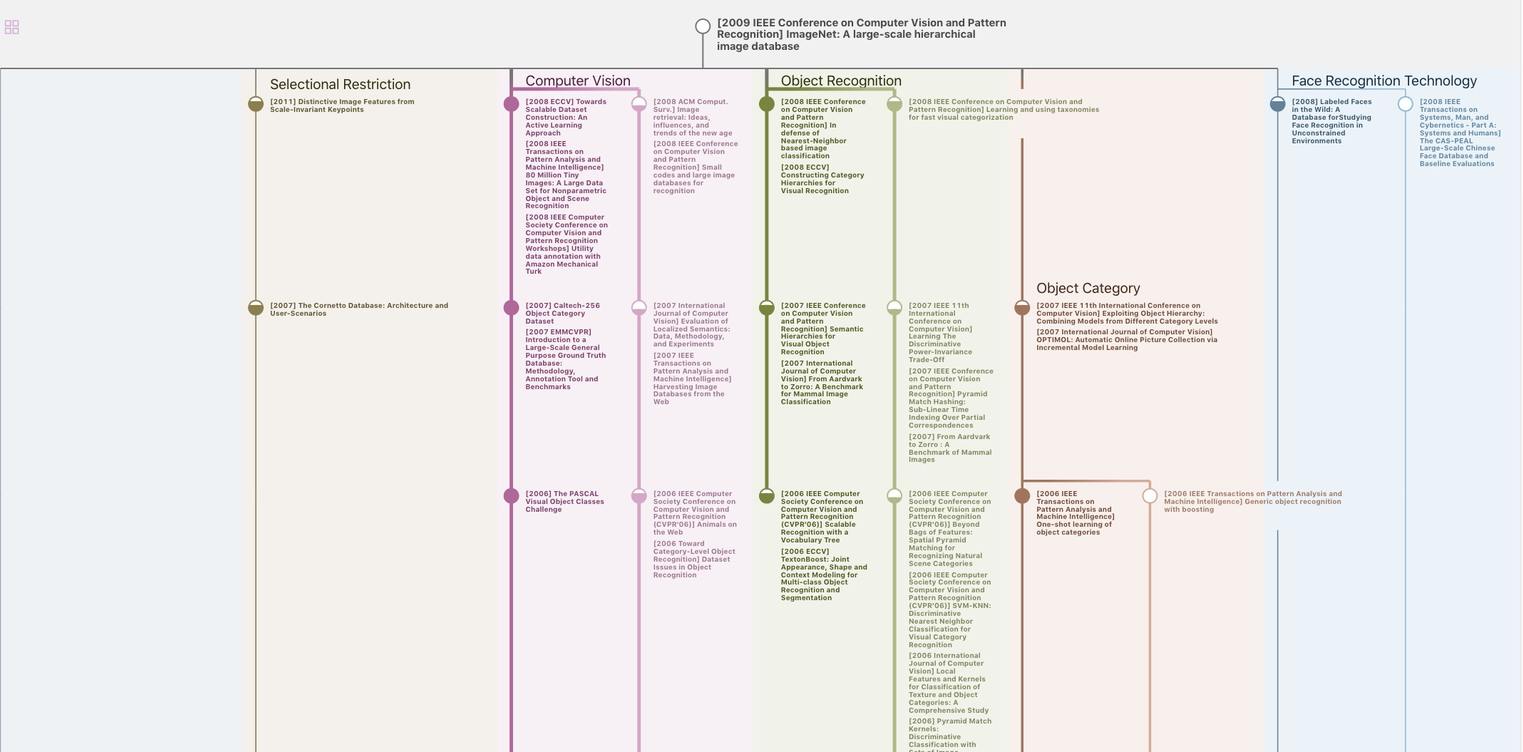
生成溯源树,研究论文发展脉络
Chat Paper
正在生成论文摘要