Fault Diagnosis of a Switch Machine to Prevent High-Speed Railway Accidents Combining Bi-Directional Long Short-Term Memory with the Multiple Learning Classification Based on Associations Model
MACHINES(2023)
摘要
The fault diagnosis of a switch machine is vital for high-speed railway operations because switch machines play an important role in the safe operation of high-speed railways, which often have faults because of their complicated working conditions. To improve the accuracy of turnout fault diagnosis for high-speed railways and prevent accidents from occurring, a combination of bi-directional long short-term memory (BiLSTM) with the multiple learning classification based on associations (MLCBA) model using the operation and maintenance text data of switch machines is proposed in this research. Due to the small probability of faults for a switch machine, it is difficult to form a diagnosis with the small amount of sample data, and more fault text features can be extracted with feedforward in a BiLSTM model. Then, the high-quality rules of the text data can be acquired by replacing the SoftMax classification with MLCBA in the output of the BiLSTM model. In this way, the identification of switch machine faults in a high-speed railway can be realized, and the experimental results show that the Accuracy and Recall of the fault diagnosis can reach 95.66% and 96.29%, respectively, as shown in the analysis of the ZYJ7 turnout fault text data of a Chinese railway bureau from five recent years. Therefore, the combined BiLSTM and MLCBA model can not only realize the accurate diagnosis of small-probability turnout faults but can also prevent high-speed railway accidents from occurring and ensure the safe operation of high-speed railways.
更多查看译文
关键词
high-speed railway,switch machine,fault diagnosis,text data,BiLSTM and MLCBA
AI 理解论文
溯源树
样例
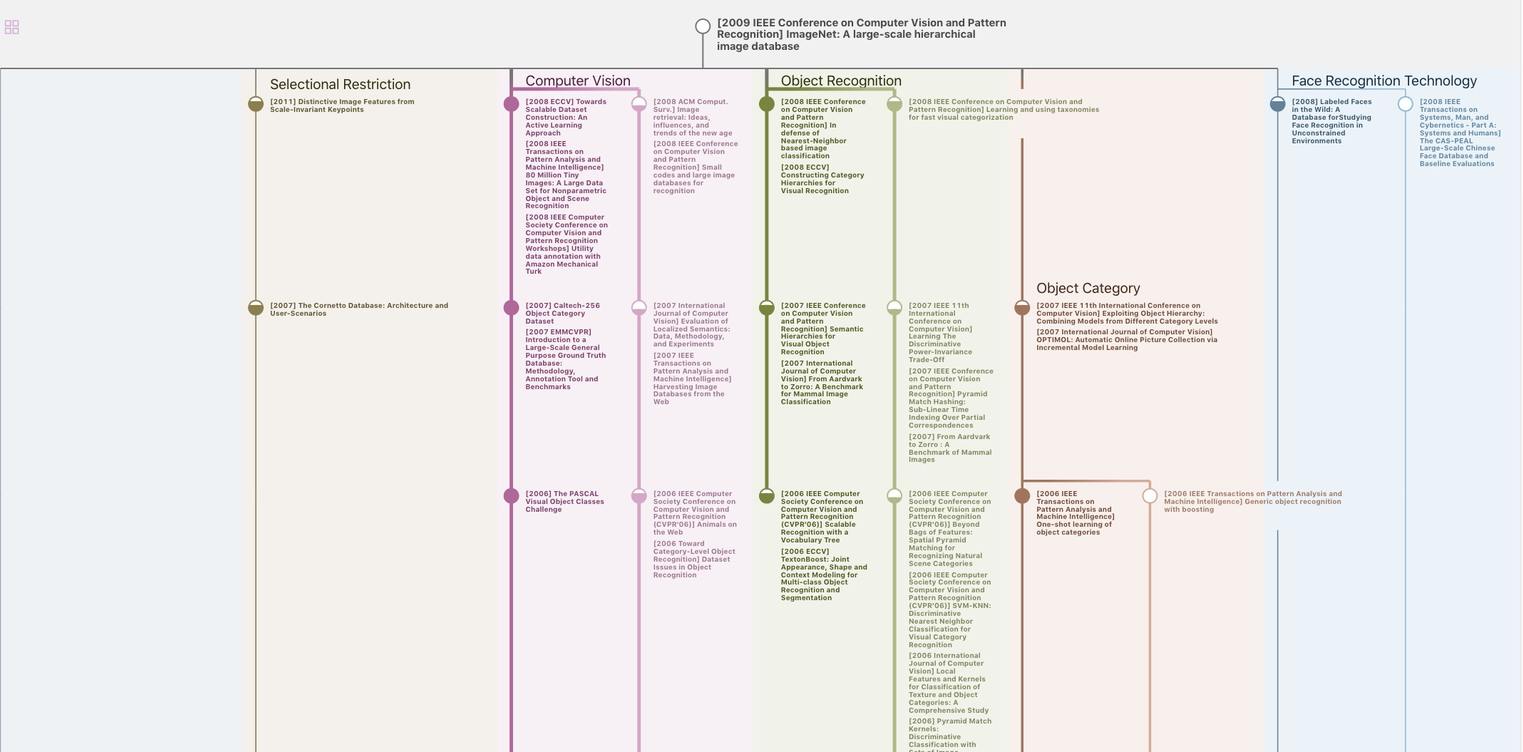
生成溯源树,研究论文发展脉络
Chat Paper
正在生成论文摘要