Transformer-Based Composite Language Models for Text Evaluation and Classification
MATHEMATICS(2023)
摘要
Parallel natural language processing systems were previously successfully tested on the tasks of part-of-speech tagging and authorship attribution through mini-language modeling, for which they achieved significantly better results than independent methods in the cases of seven European languages. The aim of this paper is to present the advantages of using composite language models in the processing and evaluation of texts written in arbitrary highly inflective and morphology-rich natural language, particularly Serbian. A perplexity-based dataset, the main asset for the methodology assessment, was created using a series of generative pre-trained transformers trained on different representations of the Serbian language corpus and a set of sentences classified into three groups (expert translations, corrupted translations, and machine translations). The paper describes a comparative analysis of calculated perplexities in order to measure the classification capability of different models on two binary classification tasks. In the course of the experiment, we tested three standalone language models (baseline) and two composite language models (which are based on perplexities outputted by all three standalone models). The presented results single out a complex stacked classifier using a multitude of features extracted from perplexity vectors as the optimal architecture of composite language models for both tasks.
更多查看译文
关键词
language modeling,language models,composite structures,machine learning,Serbian language,text classification
AI 理解论文
溯源树
样例
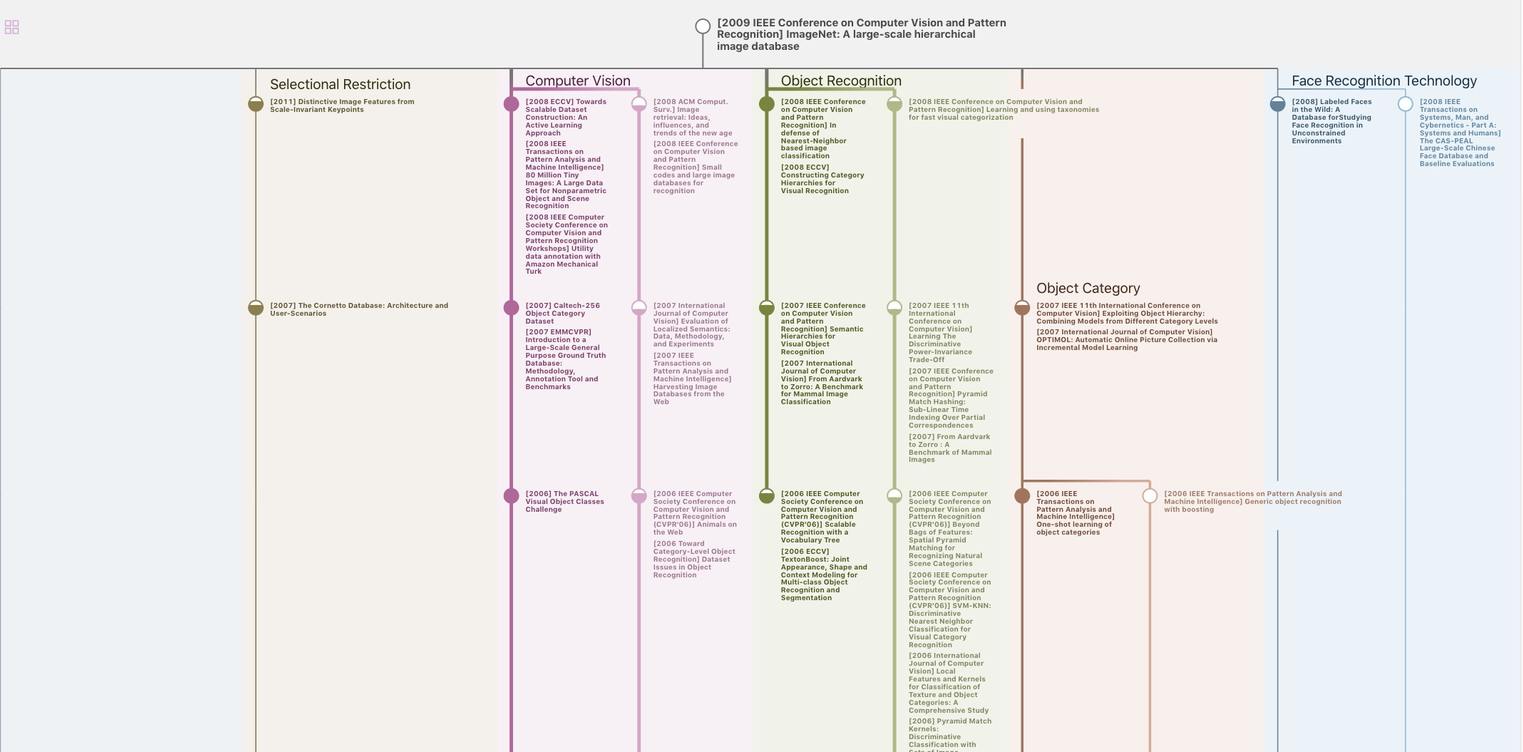
生成溯源树,研究论文发展脉络
Chat Paper
正在生成论文摘要