Gaussian Process-Based Transfer Kernel Learning for Unsupervised Domain Adaptation
MATHEMATICS(2023)
摘要
The discriminability and transferability of models are two important factors for the success of domain adaptation methods. Recently, some domain adaptation methods have improved models by adding a discriminant information extraction module. However, these methods need to carefully balance the discriminability and transferability of a model. To address this problem, we propose a new deep domain adaptation method, Gaussian Process-based Transfer Kernel Learning (GPTKL), which can perform domain knowledge transfer and improve the discrimination ability of the model simultaneously. GPTKL uses the kernel similarity between all samples in the source and target domains as a priori information to establish a cross-domain Gaussian process. By maximizing its likelihood function, GPTKL reduces the domain discrepancy between the source and target domains, thereby enhancing generalization across domains. At the same time, GPTKL introduces the deep kernel learning strategy into the cross-domain Gaussian process to learn a transfer kernel function based on deep features. Through transfer kernel learning, GPTKL learns a deep feature space with both discriminability and transferability. In addition, GPTKL uses cross-entropy and mutual information to learn a classification model shared by the source and target domains. Experiments on four benchmarks show that GPTKL achieves superior classification performance over state-of-the-art methods.
更多查看译文
关键词
unsupervised domain adaptation,Gaussian process,deep learning,kernel method
AI 理解论文
溯源树
样例
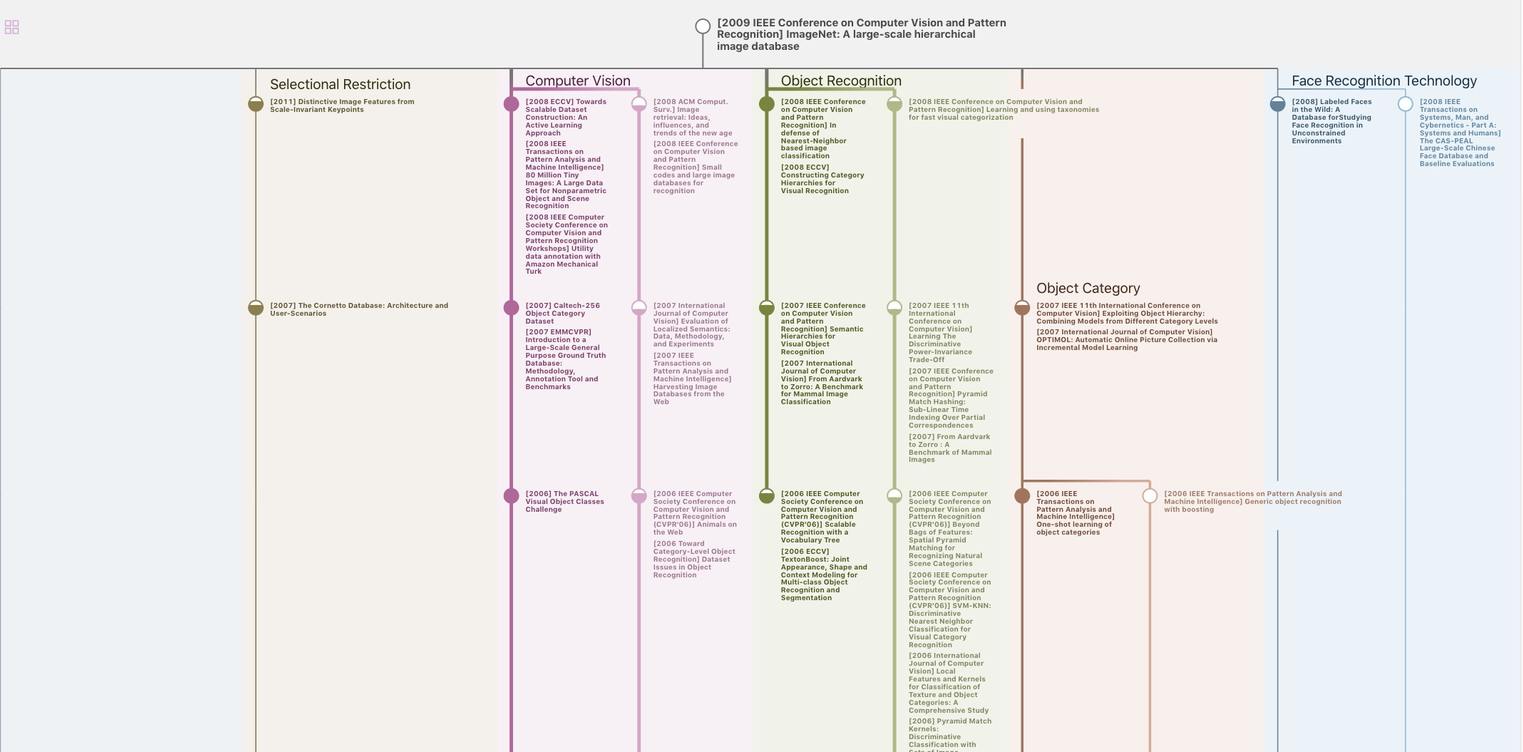
生成溯源树,研究论文发展脉络
Chat Paper
正在生成论文摘要