BEMF-Net: Semantic Segmentation of Large-Scale Point Clouds via Bilateral Neighbor Enhancement and Multi-Scale Fusion
Remote Sensing(2023)
摘要
The semantic segmentation of point clouds is a crucial undertaking in 3D reconstruction and holds great importance. However, achieving precise semantic segmentation represents a significant hurdle. In this paper, we present BEMF-Net, an efficient method for large-scale environments. It starts with an effective feature extraction method. Unlike images, 3D data comprise not only geometric relations but also texture information. To accurately depict the scene, it is crucial to take into account the impacts of texture and geometry on the task, and incorporate modifications to improve feature description. Additionally, we present a multi-scale feature fusion technique that effectively promotes the interaction between features at different resolutions. The approach mitigates the problem of the smoothing of detailed information caused by downsampling mechanisms, while ensuring the integrity of features across different layers, allowing a more comprehensive representation of the point cloud. We confirmed the effectiveness of this method by testing it on benchmark datasets such as S3DIS, SensatUrban, and Toronto3D.
更多查看译文
关键词
urban scene,point cloud,semantic segmentation,deep learning,feature enhancement,multi-scale fusion
AI 理解论文
溯源树
样例
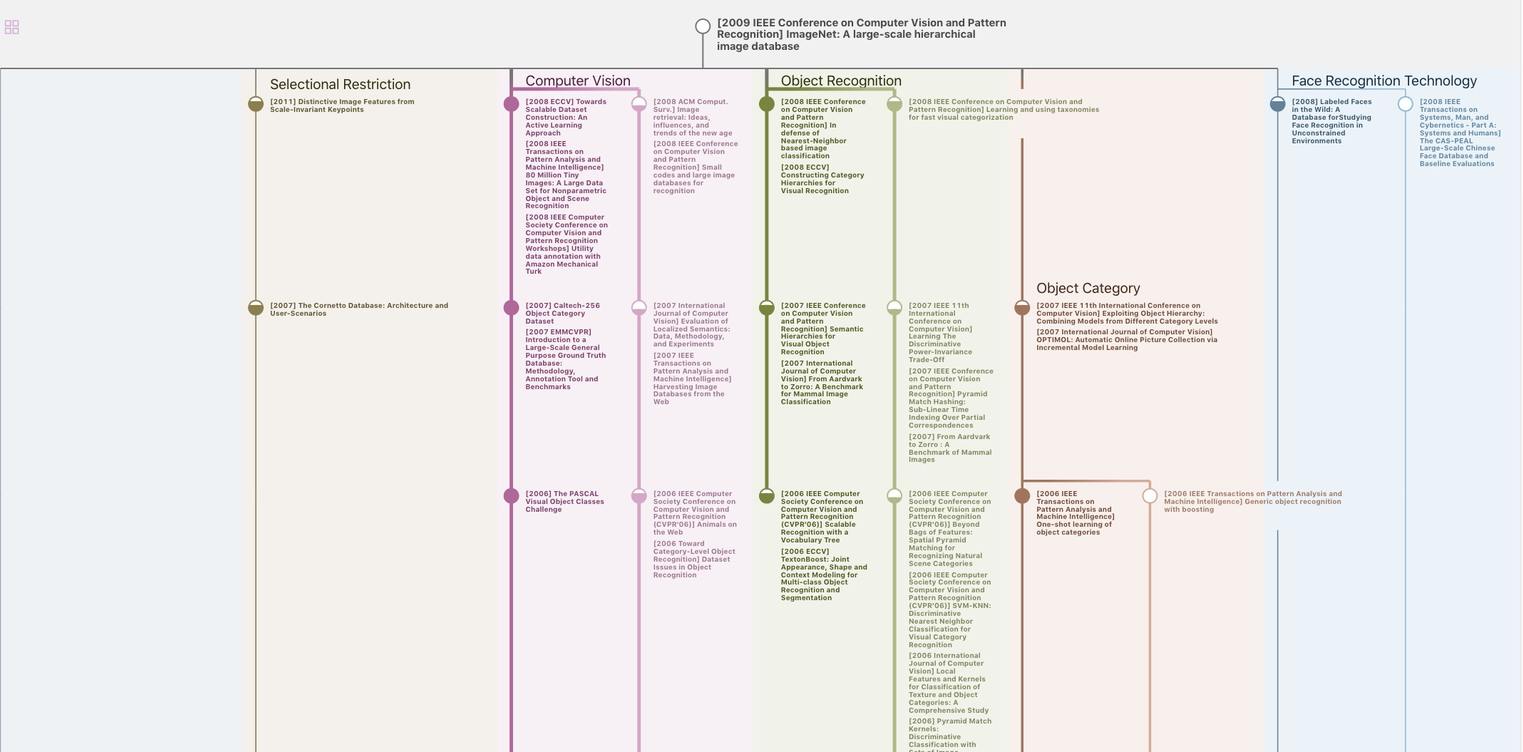
生成溯源树,研究论文发展脉络
Chat Paper
正在生成论文摘要