Personalized Federated Learning Algorithm with Adaptive Clustering for Non-IID IoT Data Incorporating Multi-Task Learning and Neural Network Model Characteristics
SENSORS(2023)
摘要
The proliferation of IoT devices has led to an unprecedented integration of machine learning techniques, raising concerns about data privacy. To address these concerns, federated learning has been introduced. However, practical implementations face challenges, including communication costs, data and device heterogeneity, and privacy security. This paper proposes an innovative approach within the context of federated learning, introducing a personalized joint learning algorithm for Non-IID IoT data. This algorithm incorporates multi-task learning principles and leverages neural network model characteristics. To overcome data heterogeneity, we present a novel clustering algorithm designed specifically for federated learning. Unlike conventional methods that require a predetermined number of clusters, our approach utilizes automatic clustering, eliminating the need for fixed cluster specifications. Extensive experimentation demonstrates the exceptional performance of the proposed algorithm, particularly in scenarios with specific client distributions. By significantly improving the accuracy of trained models, our approach not only addresses data heterogeneity but also strengthens privacy preservation in federated learning. In conclusion, we offer a robust solution to the practical challenges of federated learning in IoT environments. By combining personalized joint learning, automatic clustering, and neural network model characteristics, we facilitate more effective and privacy-conscious machine learning in Non-IID IoT data settings.
更多查看译文
关键词
Non-IID IoT data,adaptive clustering,personalized federated learning
AI 理解论文
溯源树
样例
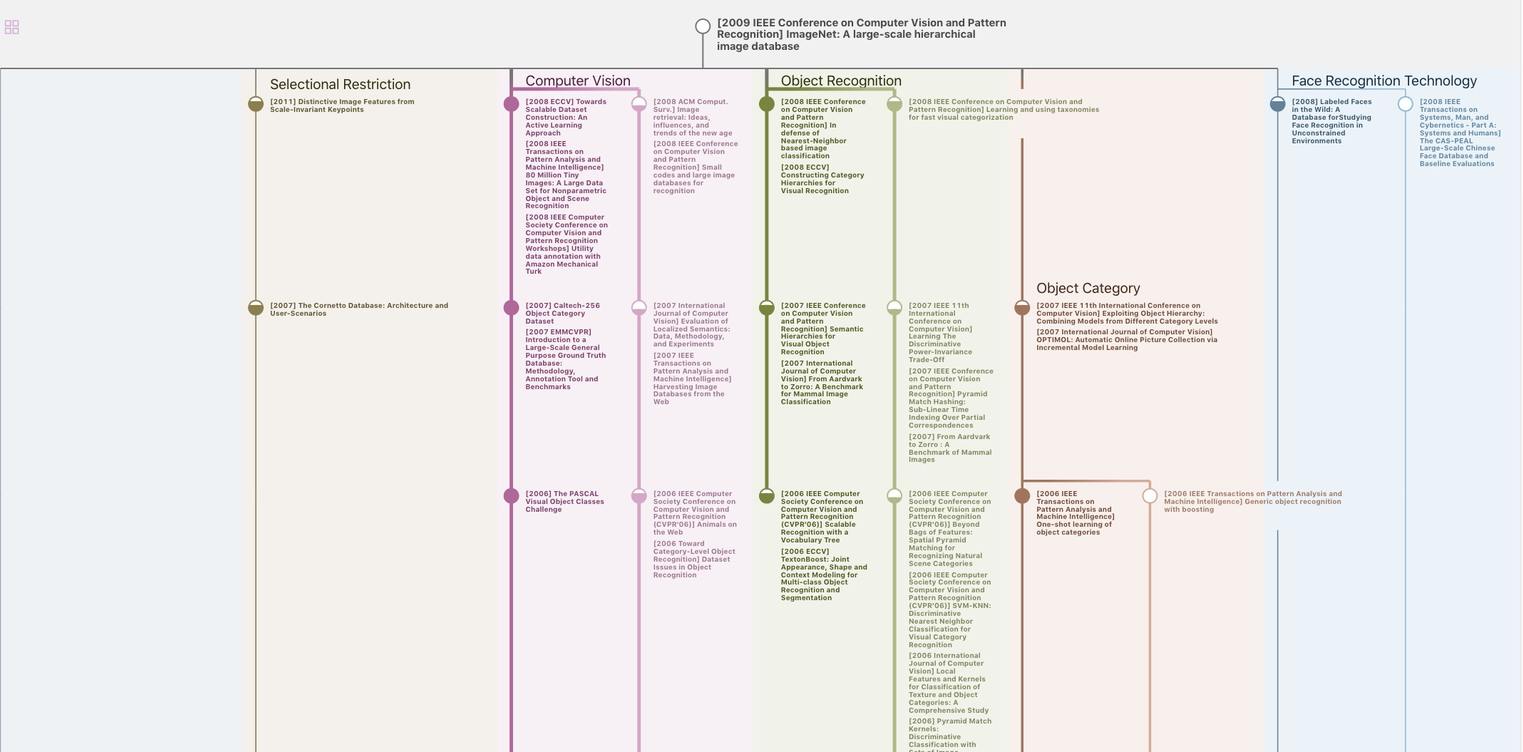
生成溯源树,研究论文发展脉络
Chat Paper
正在生成论文摘要