A Methodology of Condition Monitoring System Utilizing Supervised and Semi-Supervised Learning in Railway
SENSORS(2023)
摘要
In this paper, research was conducted on anomaly detection of wheel flats. In the railway sector, conducting tests with actual railway vehicles is challenging due to safety concerns for passengers and maintenance issues as it is a public industry. Therefore, dynamics software was utilized. Next, STFT (short-time Fourier transform) was performed to create spectrogram images. In the case of railway vehicles, control, monitoring, and communication are performed through TCMS, but complex analysis and data processing are difficult because there are no devices such as GPUs. Furthermore, there are memory limitations. Therefore, in this paper, the relatively lightweight models LeNet-5, ResNet-20, and MobileNet-V3 were selected for deep learning experiments. At this time, the LeNet-5 and MobileNet-V3 models were modified from the basic architecture. Since railway vehicles are given preventive maintenance, it is difficult to obtain fault data. Therefore, semi-supervised learning was also performed. At this time, the Deep One Class Classification paper was referenced. The evaluation results indicated that the modified LeNet-5 and MobileNet-V3 models achieved approximately 97% and 96% accuracy, respectively. At this point, the LeNet-5 model showed a training time of 12 min faster than the MobileNet-V3 model. In addition, the semi-supervised learning results showed a significant outcome of approximately 94% accuracy when considering the railway maintenance environment. In conclusion, considering the railway vehicle maintenance environment and device specifications, it was inferred that the relatively simple and lightweight LeNet-5 model can be effectively utilized while using small images.
更多查看译文
关键词
condition monitoring,STFT (short time Fourier transform),CNN,supervised learning,LeNet-5,ResNet-20,MobileNet,semi-supervised learning,Deep One Class Classification,TCMS (train control and management system)
AI 理解论文
溯源树
样例
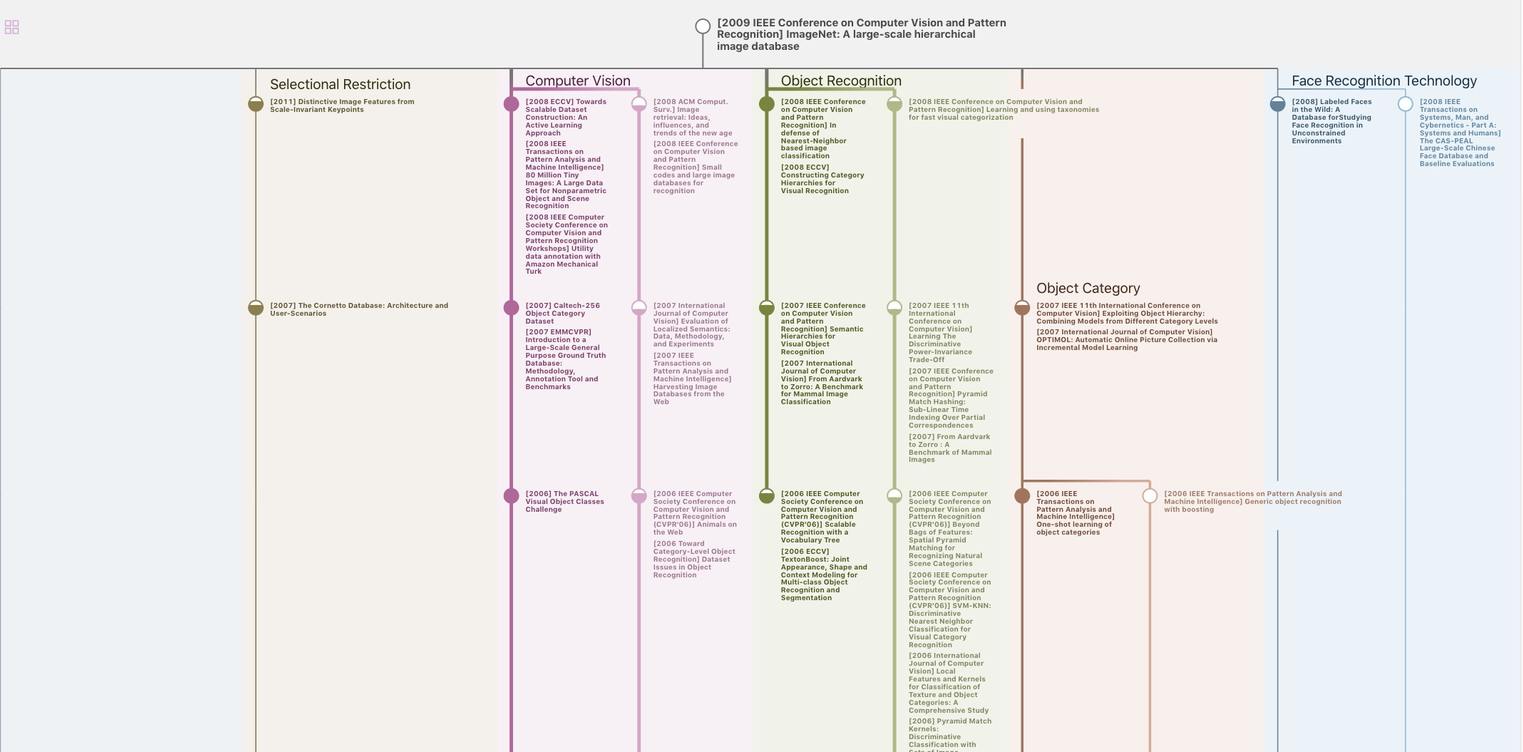
生成溯源树,研究论文发展脉络
Chat Paper
正在生成论文摘要