Artifact Augmentation for Enhanced Tissue Detection in Microscope Scanner Systems
SENSORS(2023)
摘要
As the field of routine pathology transitions into the digital realm, there is a surging demand for the full automation of microscope scanners, aiming to expedite the process of digitizing tissue samples, and consequently, enhancing the efficiency of case diagnoses. The key to achieving seamless automatic imaging lies in the precise detection and segmentation of tissue sample regions on the glass slides. State-of-the-art approaches for this task lean heavily on deep learning techniques, particularly U-Net convolutional neural networks. However, since samples can be highly diverse and prepared in various ways, it is almost impossible to be fully prepared for and cover every scenario with training data. We propose a data augmentation step that allows artificially modifying the training data by extending some artifact features of the available data to the rest of the dataset. This procedure can be used to generate images that can be considered synthetic. These artifacts could include felt pen markings, speckles of dirt, residual bubbles in covering glue, or stains. The proposed approach achieved a 1-6% improvement for these samples according to the F1 Score metric.
更多查看译文
关键词
tissue detection,convolutional neural network,deep learning,augmentation,U-Net,segmentation,classification,digital microscope scanner,digital pathology
AI 理解论文
溯源树
样例
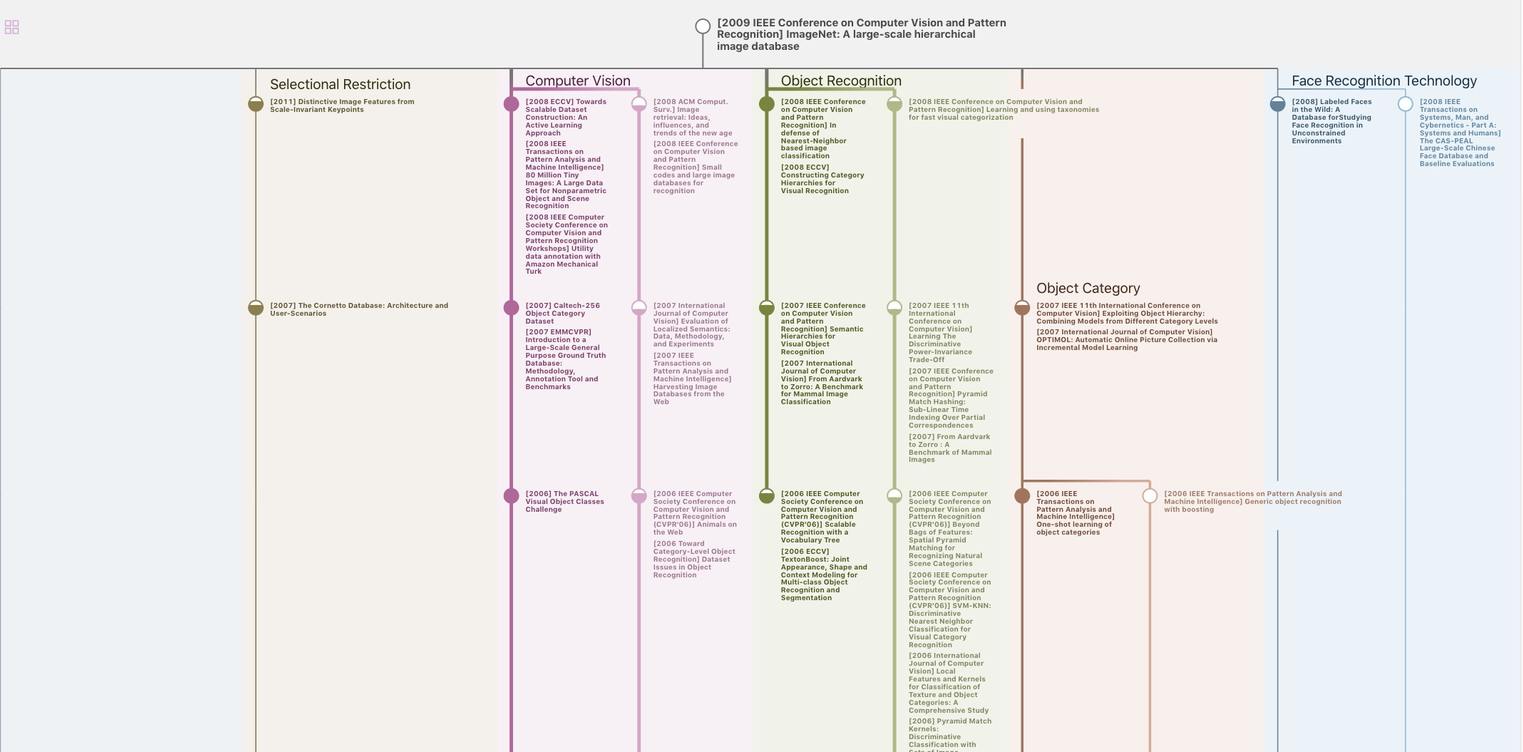
生成溯源树,研究论文发展脉络
Chat Paper
正在生成论文摘要