Web Service for HIV Drug Resistance Prediction Based on Analysis of Amino Acid Substitutions in Main Drug Targets
Viruses(2023)
摘要
Predicting viral drug resistance is a significant medical concern. The importance of this problem stimulates the continuous development of experimental and new computational approaches. The use of computational approaches allows researchers to increase therapy effectiveness and reduce the time and expenses involved when the prescribed antiretroviral therapy is ineffective in the treatment of infection caused by the human immunodeficiency virus type 1 (HIV-1). We propose two machine learning methods and the appropriate models for predicting HIV drug resistance related to amino acid substitutions in HIV targets: (i) k-mers utilizing the random forest and the support vector machine algorithms of the scikit-learn library, and (ii) multi-n-grams using the Bayesian approach implemented in MultiPASSR software. Both multi-n-grams and k-mers were computed based on the amino acid sequences of HIV enzymes: reverse transcriptase and protease. The performance of the models was estimated by five-fold cross-validation. The resulting classification models have a relatively high reliability (minimum accuracy for the drugs is 0.82, maximum: 0.94) and were used to create a web application, HVR (HIV drug Resistance), for the prediction of HIV drug resistance to protease inhibitors and nucleoside and non-nucleoside reverse transcriptase inhibitors based on the analysis of the amino acid sequences of the appropriate HIV proteins from clinical samples.
更多查看译文
关键词
HIV/AIDS,antiretrovirals,resistance,machine learning,random forest,supporting vector machines,web-service
AI 理解论文
溯源树
样例
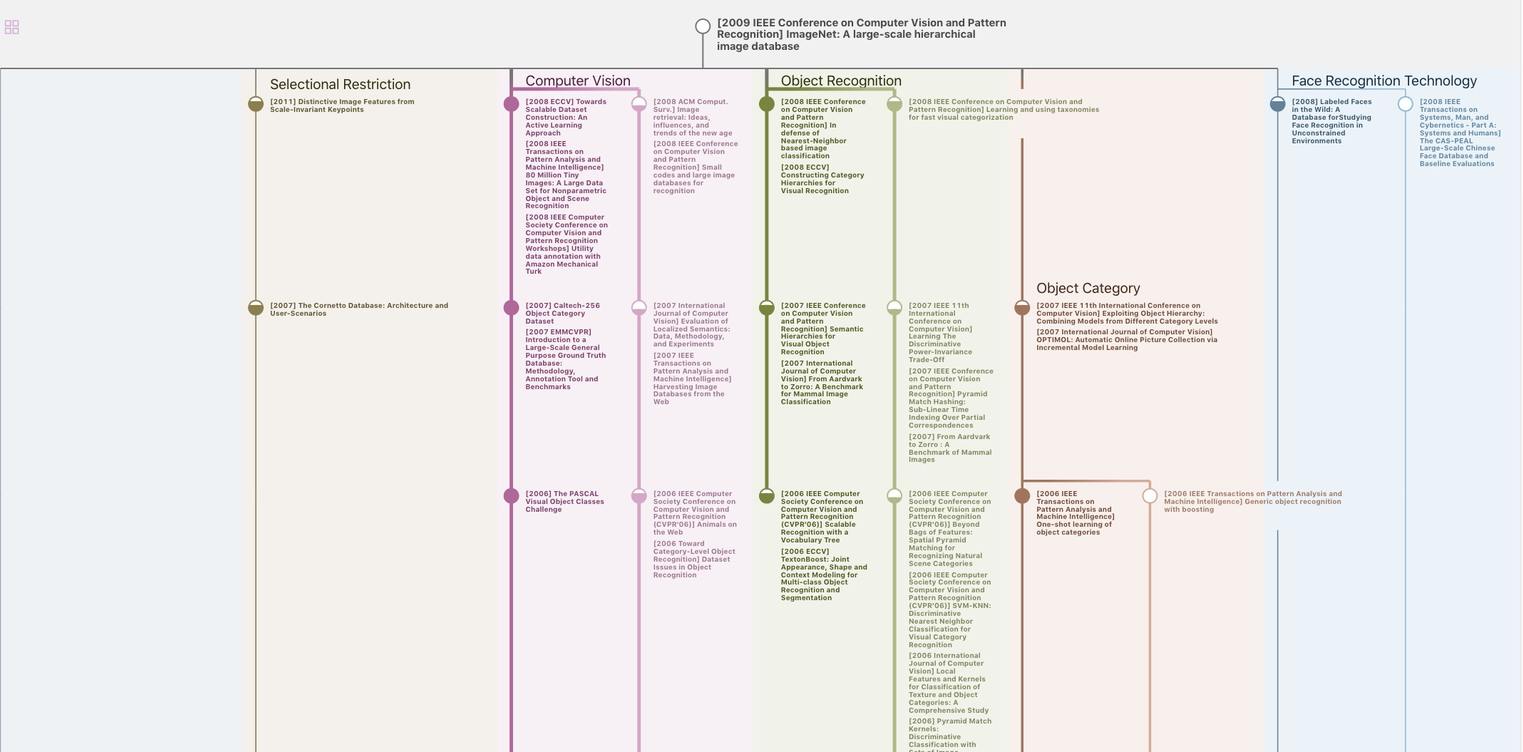
生成溯源树,研究论文发展脉络
Chat Paper
正在生成论文摘要