Anatomy-Aware Masking for Inpainting in Medical Imaging
SHAPE IN MEDICAL IMAGING, SHAPEMI 2023(2023)
摘要
Inpainting has recently been employed as a successful deep-learning technique for unsupervised model discovery in medical image analysis by taking advantage of the strong priors learned by models to reconstruct the structure and texture of missing parts in images. Even though the learned features depend on the masks as well as the images, the masks used for inpainting are typically random and independent of the dataset, due to the unpredictability of the content of images, i.e., different objects and shapes can appear in different locations in images. However, this is rarely the case for medical imaging data since they are obtained from similar anatomies. Still, random square masks are the most popular technique for inpainting in medical imaging. In this work, we propose a pipeline to generate, position and sample the masks to efficiently learn the shape and structures of the anatomy and generate a myriad of diverse anatomy-aware masks, aiding the model in learning the statistical shape prior to the topology of the organs of interest. We demonstrate the impact of our approach compared to other masking mechanisms in the reconstruction of anatomy. We compare the effectiveness of our proposed masking approach over square-shaped masks, which are traditionally used in medical imaging, and irregular shape masks, which are used in SOTA inpainting literature. Project Page: https://anatomyaware.github.io/.
更多查看译文
关键词
Inpainting,Mask Generation,Superpixel,Self-supervised
AI 理解论文
溯源树
样例
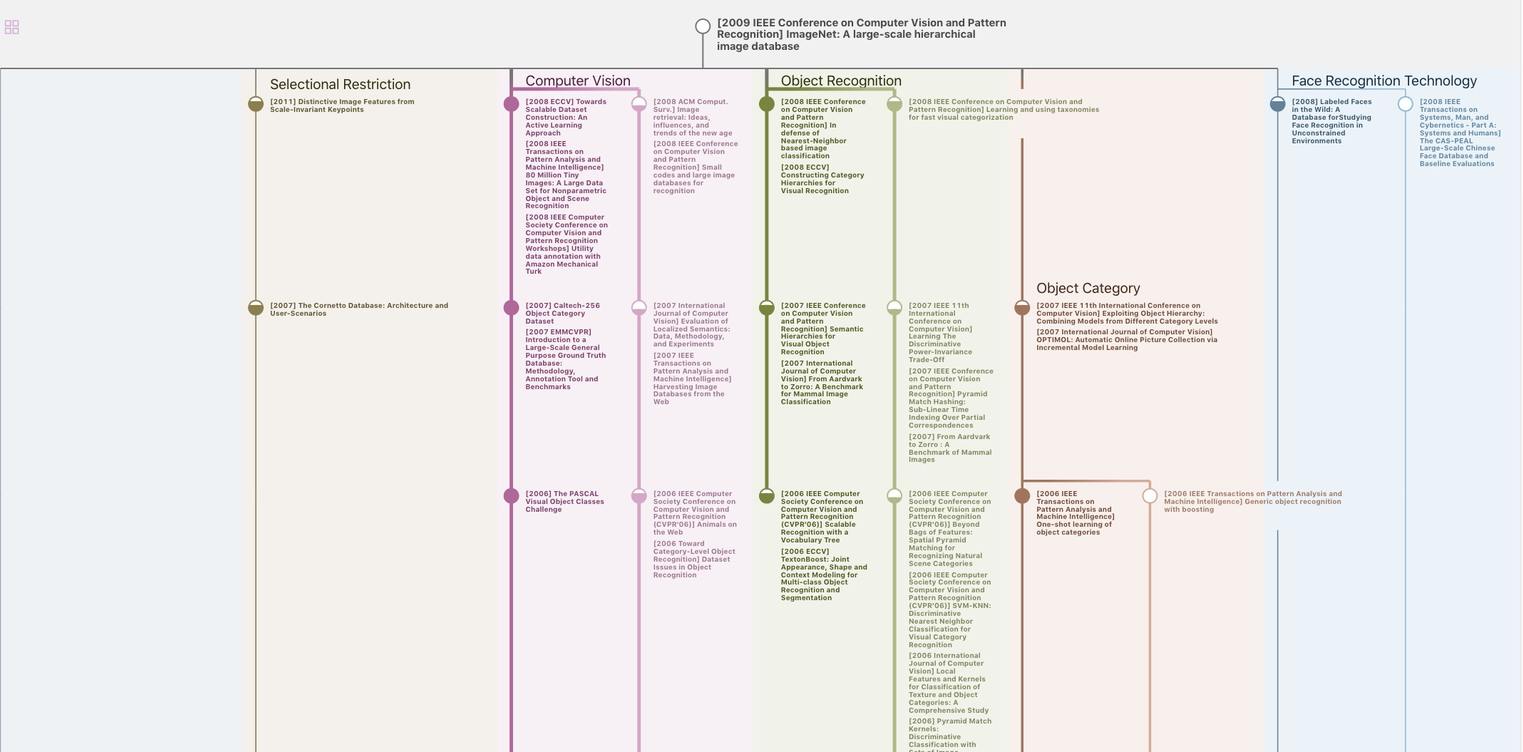
生成溯源树,研究论文发展脉络
Chat Paper
正在生成论文摘要