Disentangled Contrastive Learning for Knowledge-Aware Recommender System
SEMANTIC WEB, ISWC 2023, PART I(2023)
摘要
Knowledge Graphs (KGs) play an increasingly important role as useful side information in recommender systems. Recently, developing end-to-end models based on graph neural networks (GNNs) becomes the technical trend of knowledge-aware recommendation. However, we argue that prior methods are insufficient to discover multi-faceted user preferences based on diverse aspects of item attributes, since they only learn a single representation for each user and item. To alleviate this limitation, we focus on exploring user preferences from multiple aspects of item attributes, and propose a novel disentangled contrastive learning framework for knowledge-aware recommendation (DCLKR). Technically, we first disentangle item knowledge graph into multiple aspects for the knowledge view, and user-item interaction graph for the collaborative view, equipped with attentive neighbor assignment and embedding propagation mechanisms. Then we perform intra-view contrastive learning to encourage differences among disentangled representations in each view, and inter-view contrastive learning to transfer knowledge between the two views. Extensive experiments conducted on three benchmark datasets demonstrate the superior performance of our proposed method over the state-of-the-arts. The implementations are available at: https://github.com/Jill5/DCLKR..
更多查看译文
关键词
Recommender System,Knowledge Graphs,Disentangled Representation Learning,Contrastive Learning,Graph Neural Networks
AI 理解论文
溯源树
样例
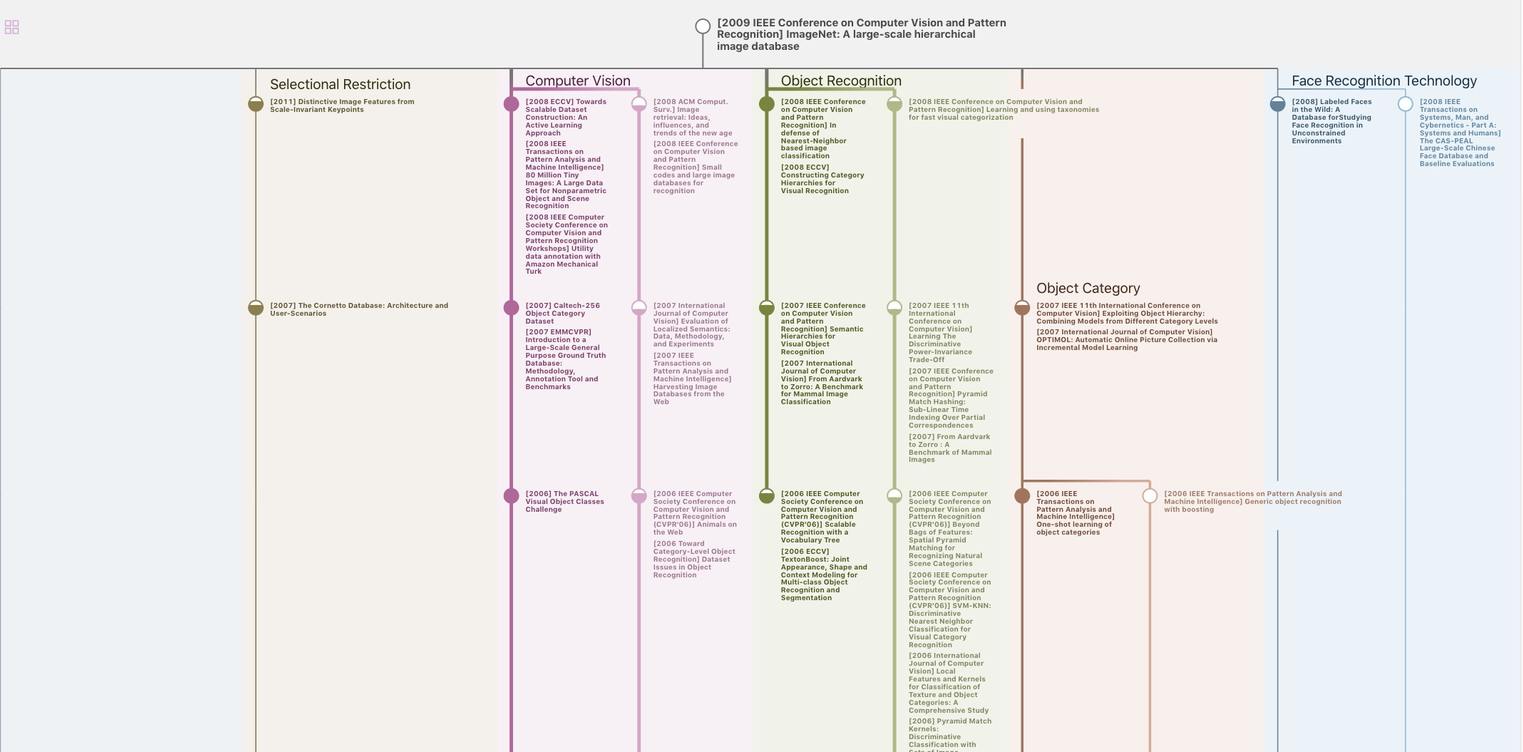
生成溯源树,研究论文发展脉络
Chat Paper
正在生成论文摘要