Dense Re-Ranking with Weak Supervision for RDF Dataset Search
SEMANTIC WEB, ISWC 2023, PART I(2023)
摘要
Dataset search aims to find datasets that are relevant to a keyword query. Existing dataset search engines rely on conventional sparse retrieval models (e.g., BM25). Dense models (e.g., BERT-based) remain under-investigated for two reasons: the limited availability of labeled data for fine-tuning such a deep neural model, and its limited input capacity relative to the large size of a dataset. To fill the gap, in this paper, we study dense re-ranking for RDF dataset search. Our re-ranking model encodes the metadata of RDF datasets and also their actual RDF data-by extracting a small yet representative subset of data to accommodate large datasets. To address the insufficiency of training data, we adopt a coarse-to-fine tuning strategy where we warm up the model with weak supervision from a large set of automatically generated queries and relevance labels. Experiments on the ACORDAR test collection demonstrate the effectiveness of our approach, which considerably improves the retrieval accuracy of existing sparse models.
更多查看译文
关键词
Dataset search,Dense re-ranking,Data augmentation
AI 理解论文
溯源树
样例
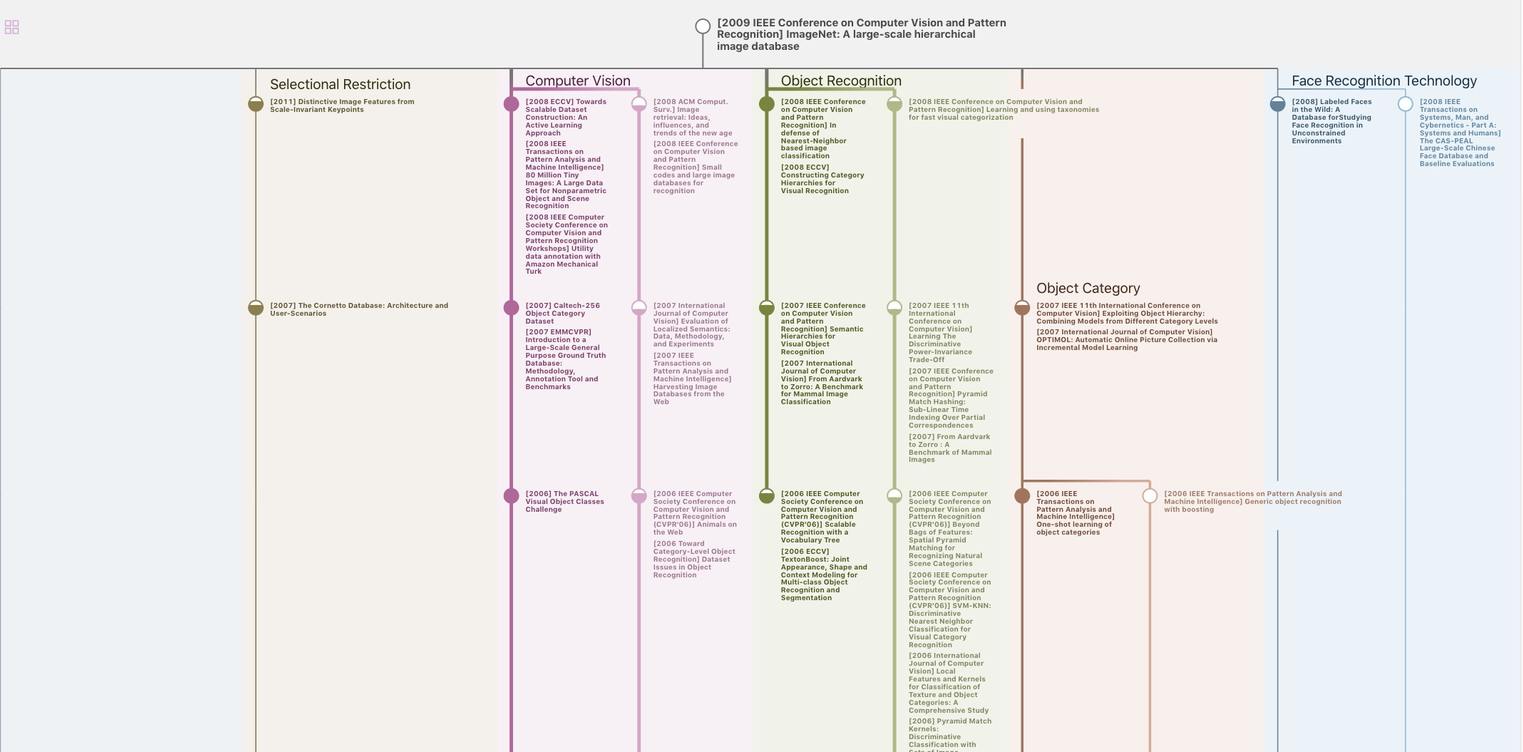
生成溯源树,研究论文发展脉络
Chat Paper
正在生成论文摘要