Non-uniform sampling to enhance the performance of compact NMR for characterizing new psychoactive substances
MAGNETIC RESONANCE IN CHEMISTRY(2024)
摘要
Efficient and robust analytical methods are needed to improve the identification and subsequent regulation of new psychoactive substances (NPS). NMR spectroscopy is a unique method able to determine the structure of small molecules such as NPS even in mixtures. However, high-field NMR analysis is associated with expensive purchase and maintenance costs. For more than a decade, compact NMR spectrometers have changed this paradigm. It was recently shown that a dedicated analytical workflow combining compact NMR and databases could identify the molecular structure of NPS, in spite of the lower spectral dispersion and sensitivity of compact spectrometers. This approach relies on 1H-13C HSQC to both recognize NPS and elucidate the structure of unknown substances. Still, its performance is limited by the need to compromise between resolution and experiment time. Here, we show that this strategy can be significantly improved by implementing non-uniform sampling (NUS) to improve spectral resolution in the 13C dimension of HSQC at no cost in terms of experiment time. Gains in the range of 3 to 4 in resolution are achieved for pure NPS and for a mixture. Finally, 2D HSQC with NUS was applied to improve the identification of NPS with the assistance of databases. The resulting method appears as a useful tool for the characterization of NPS in mixtures, which is essential for forensic laboratories. In the field of new psychoactive substance characterization, compact NMR needs improved performances. Thus, 2D HSQC with non-uniform sampling along 13C dimension, NUS 2D HSQC, is proposed to improve by 3 to 4 the spectral resolution as compared to the standard HSQC with the same time. NUS 2D HSQC was applied for the first time to better identify each NPS of a mixture with the assistance of a database.image
更多查看译文
关键词
2D HSQC,compact NMR,databases,new psychoactive substances,non-uniform sampling
AI 理解论文
溯源树
样例
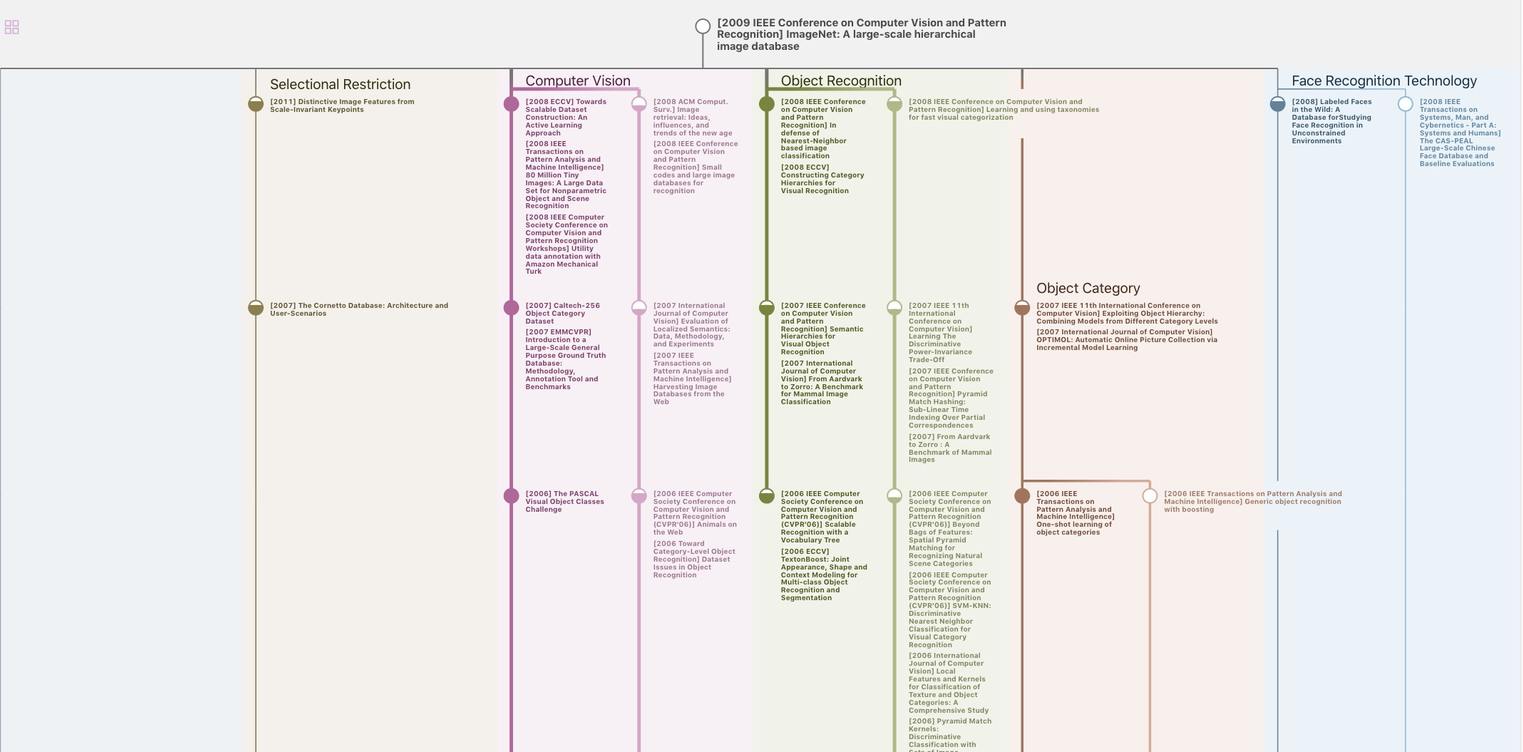
生成溯源树,研究论文发展脉络
Chat Paper
正在生成论文摘要