Can Machine Learning Aid the Selection of Percutaneous vs Surgical Revascularization?
JOURNAL OF THE AMERICAN COLLEGE OF CARDIOLOGY(2023)
摘要
BACKGROUND In patients with 3-vessel coronary artery disease (CAD) and/or left main CAD, individual risk prediction plays a key role in deciding between percutaneous coronary intervention (PCI) and coronary artery bypass grafting (CABG).OBJECTIVES The aim of this study was to assess whether these individualized revascularization decisions can be improved by applying machine learning (ML) algorithms and integrating clinical, biological, and anatomical factors.METHODS In the SYNTAX (Synergy between PCI with Taxus and Cardiac Surgery) study, ML algorithms (Lasso regression, gradient boosting) were used to develop a prognostic index for 5-year death, which was combined, in the second stage, with assigned treatment (PCI or CABG) and prespecified effect-modifiers: disease type (3-vessel or left main CAD) and anatomical SYNTAX score. The model's discriminative ability to predict the risk of 5-year death and treatment benefit between PCI and CABG was cross-validated in the SYNTAX trial (n = 1,800) and externally validated in the CREDO-Kyoto (Coronary REvascularization Demonstrating Outcome Study in Kyoto) registry (n = 7,362), and then compared with the original SYNTAX score II 2020 (SSII-2020).RESULTS The hybrid gradient boosting model performed best for predicting 5-year all-cause death with C-indexes of 0.78 (95% CI: 0.75-0.81) in cross-validation and 0.77 (95% CI: 0.76-0.79) in external validation. The ML models discriminated 5-year mortality better than the SSII-2020 in the external validation cohort and identified heterogeneity in the treatment benefit of CABG vs PCI.CONCLUSIONS An ML-based approach for identifying individuals who benefit from CABG or PCI is feasible and effective. Implementation of this model in health care systems-trained to collect large numbers of parameters-may harmonize decision making globally.(c) 2023 Published by Elsevier on behalf of the American College of Cardiology Foundation.
更多查看译文
关键词
CABG,decision-making,long-term clinical outcomes,machine learning,PCI
AI 理解论文
溯源树
样例
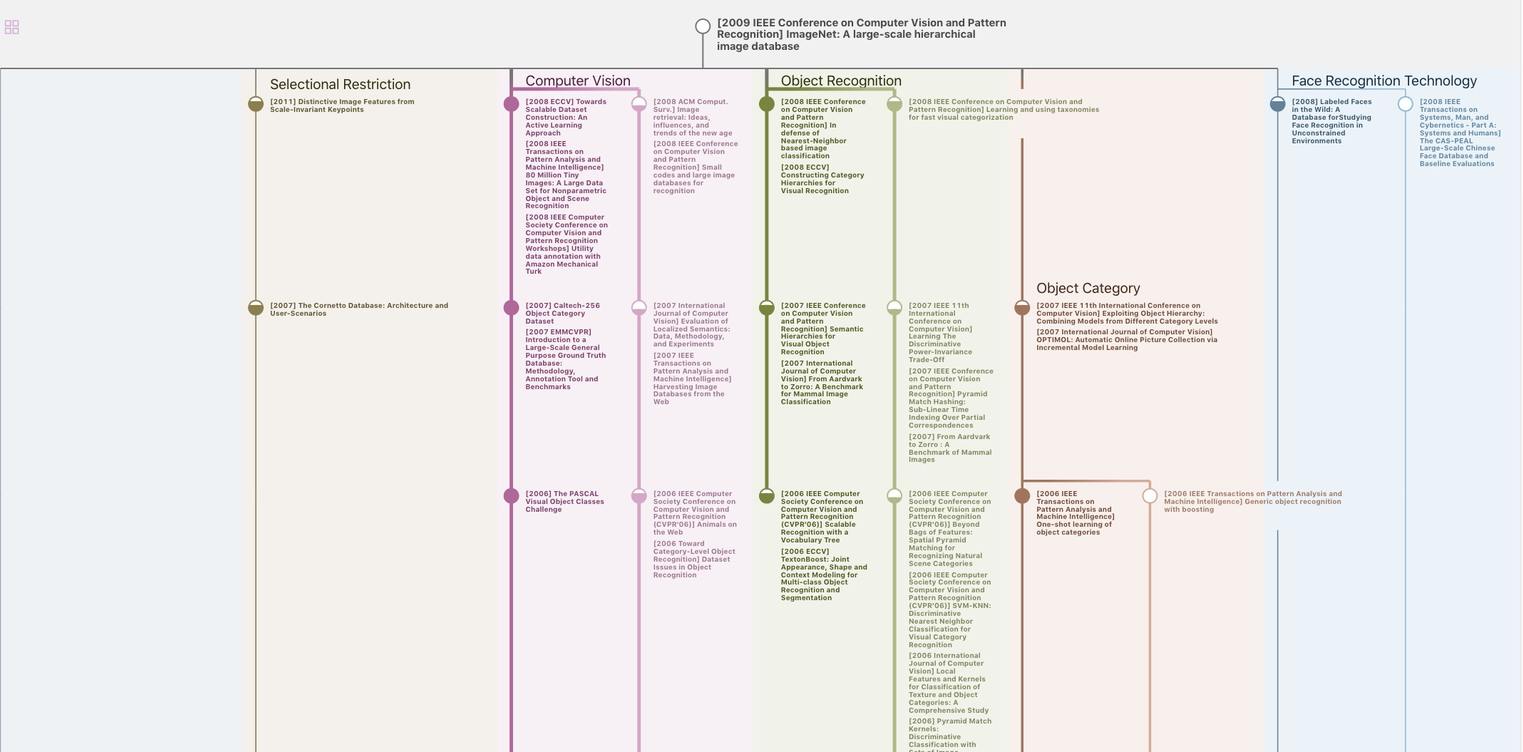
生成溯源树,研究论文发展脉络
Chat Paper
正在生成论文摘要