Zone-Level Anomaly Detection in VAV Terminal Units Using an Unsupervised Learning Approach
PROCEEDINGS OF THE 10TH ACM INTERNATIONAL CONFERENCE ON SYSTEMS FOR ENERGY-EFFICIENT BUILDINGS, CITIES, AND TRANSPORTATION, BUILDSYS 2023(2023)
摘要
Effective operation of HVAC systems is crucial to minimize energy inefficiencies and occupant discomfort. However, these systems can experience various problems, including hardware and software-related anomalies. On the other hand, conventional fault detection and diagnosis techniques mostly depend on pre-established lists of fault alarms resulting in the potential for anomalous occurrences that defy these fault classifications. This study presents a novel unsupervised approach for detecting anomalous zones in variable air volume (VAV) air handling units (AHUs). The method utilizes autoencoders (AE) and principal component analysis (PCA), based on zone-level trend logs. To evaluate the effectiveness of the proposed approach, a case study was conducted using data collected from a 71-zone VAV AHU system of an educational building in Ottawa, Canada. The proposed PCA-AE method successfully identified four anomalous zones by considering four zone-level trend logs. The findings demonstrated great adaptability for detecting a wide range of zone anomalies in VAV AHUs giving operators valuable insights about the system and notify of potential faults at early stage.
更多查看译文
关键词
HVAC,Anomaly Detection,PCA,Autoencoders
AI 理解论文
溯源树
样例
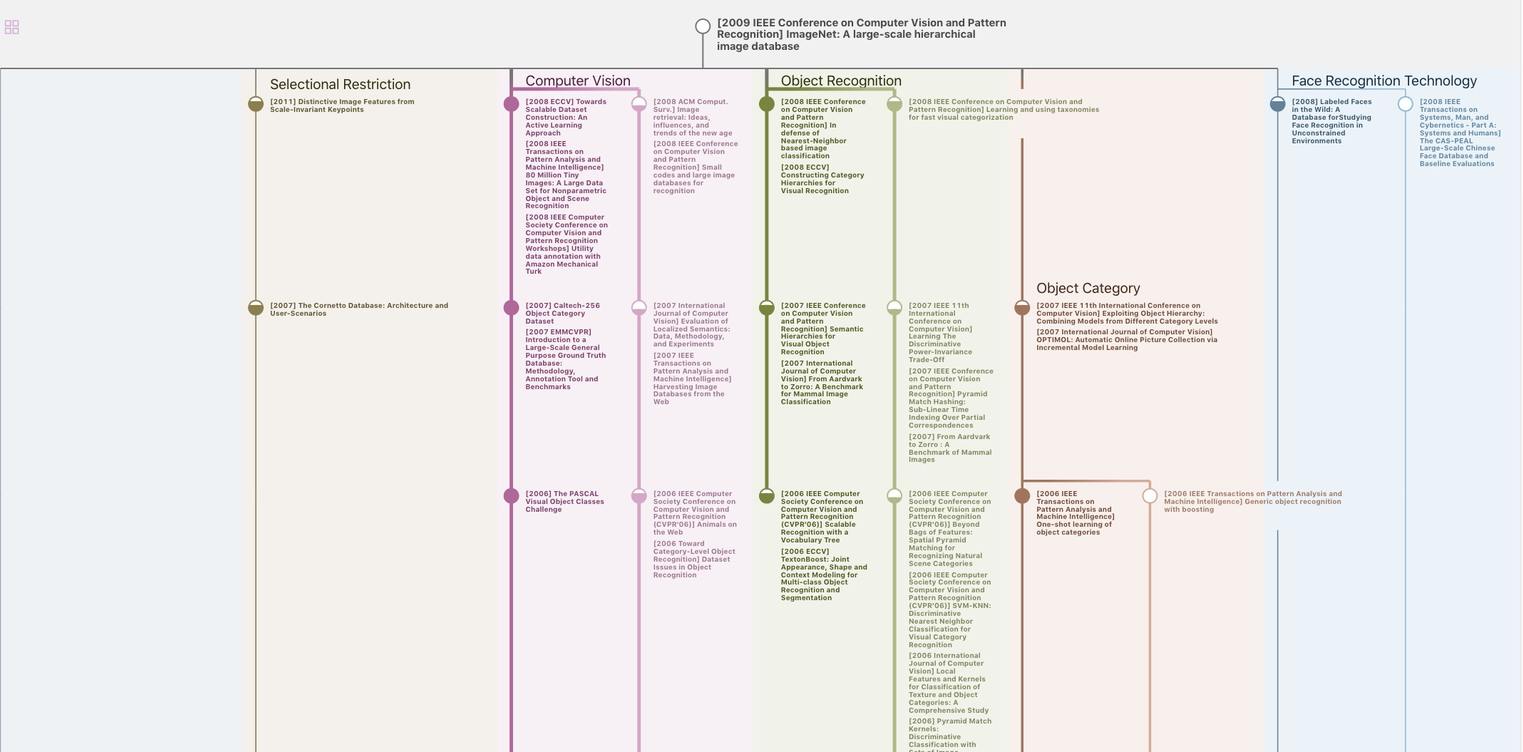
生成溯源树,研究论文发展脉络
Chat Paper
正在生成论文摘要