Explainable AI for Energy Prediction and Anomaly Detection in Smart Energy Buildings
PROCEEDINGS OF THE 10TH ACM INTERNATIONAL CONFERENCE ON SYSTEMS FOR ENERGY-EFFICIENT BUILDINGS, CITIES, AND TRANSPORTATION, BUILDSYS 2023(2023)
摘要
In recent years, the advancement of Artificial Intelligence (AI) and Advanced Metering Infrastructure (AMI) has led to the development of data-driven methods for energy prediction and anomaly detection. These methods provide automated decision support to building operators in managing and preventing energy loss. Despite the advantages of having sophisticated data-driven models, one major drawback is their lack of transparency, which limits their widespread use. The paper explores the use of the SHapely Additive exPlanations (SHAP), an explainable AI algorithm, to enhance transparency in energy prediction and anomaly detection models. Energy prediction is treated as a regression task, while anomaly detection as a binary classification. The study employs LightGBM models for both anomaly detection and energy prediction, which are tested on a large dataset containing hourly smart metering data from over 200 real buildings. The energy prediction model achieves an R-2 score of 0.975, while the anomaly detection model obtains an AUC-ROC score of 0.942. These models are augmented with SHAP value-based visualizations, which provide both local and global explanations of these models, offering valuable insights into the factors influencing their predictions. Additionally, the present study introduces a framework that seamlessly integrates feature transformations within the model, while SHAP operates on the interpretable feature space, enhancing the explanations provided by SHAP values.
更多查看译文
关键词
XAI,Energy Prediction,Anomaly Detection,LightGBM
AI 理解论文
溯源树
样例
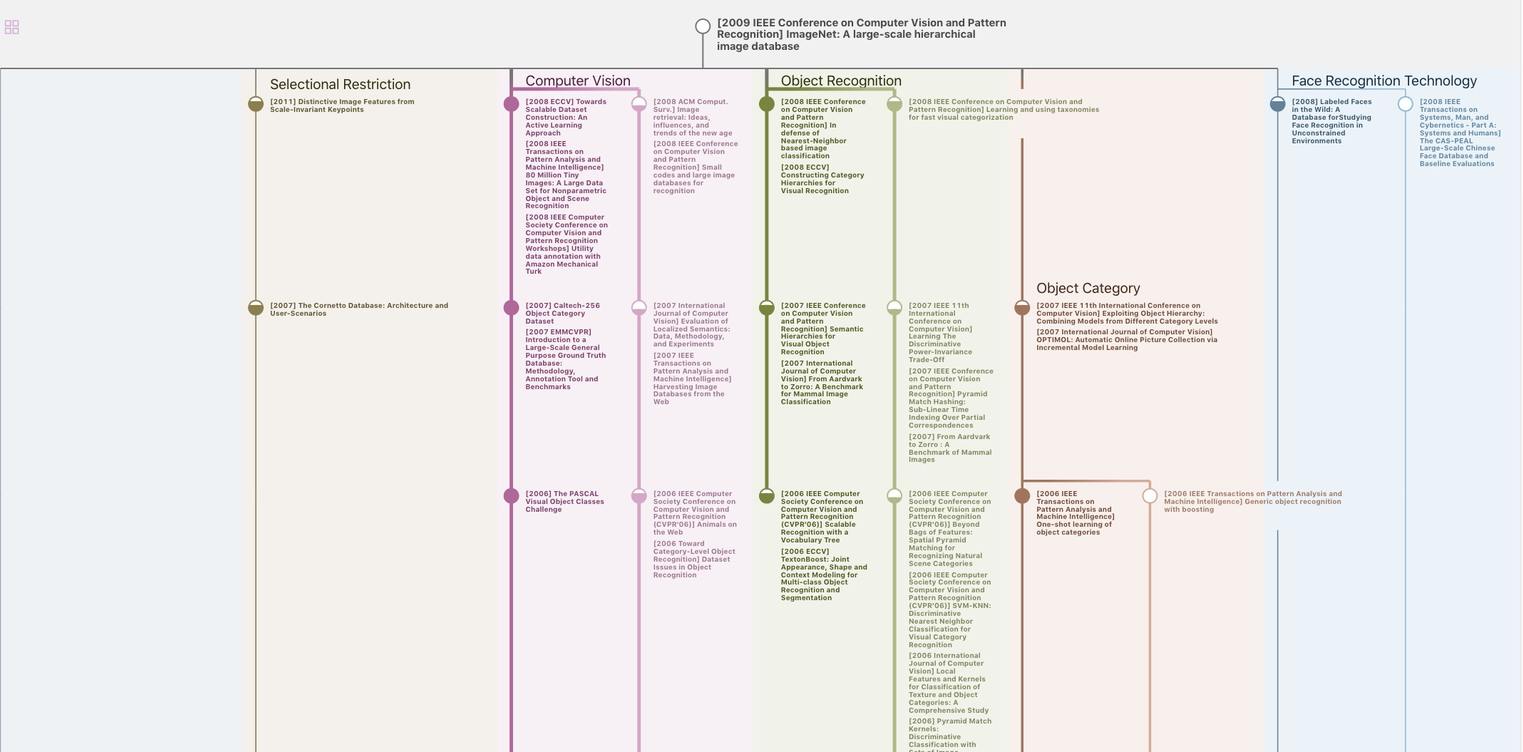
生成溯源树,研究论文发展脉络
Chat Paper
正在生成论文摘要