What's The Point: AutoEncoding Building Point Names
PROCEEDINGS OF THE 10TH ACM INTERNATIONAL CONFERENCE ON SYSTEMS FOR ENERGY-EFFICIENT BUILDINGS, CITIES, AND TRANSPORTATION, BUILDSYS 2023(2023)
摘要
Deciphering the point names of various equipment within commercial buildings remains a vexing challenge. Both the lack of standards, and the usual idiosyncrasies in naming conventions, add to the complexity faced by engineers as they attempt to analyse and optimise a building's systems. For example, T, Tmp, Temp, C, and Celsius could all be used to denote a temperature sensor. Likewise, the delimiters (e.g.,., -, or _) that apportion parts of a point name varies considerably. This results in the possibility of both f1-AHU_tmp and Floor02.AHU.Temperature appearing in the same dataset (and often even within the same building). The initial work here explores foundational deep-learning techniques to help a human expert understand previously unseen building point names. We've applied a simplified yet effective neural-network architecture called AutoEncoders (AE) to the task, with tests done against an expansive dataset comprising of point names gathered from publicly available Brick and Mortar datasets, as well as a private corpus. This novel implementation is augmented by a unique sequential tokenising method that applies tri-grams in a sliding window over the point names, which preserves both the linearity and delimiters within point names. This is diametrically opposed to prior approaches that have either ignored the character sequences and/or removed the presence of delimiting characters. Early results show promising 91% accuracy levels achieved against previously unseen point names.
更多查看译文
关键词
brick schema,building point names,metadata inference,building management systems,auto-encoders,neural-networks
AI 理解论文
溯源树
样例
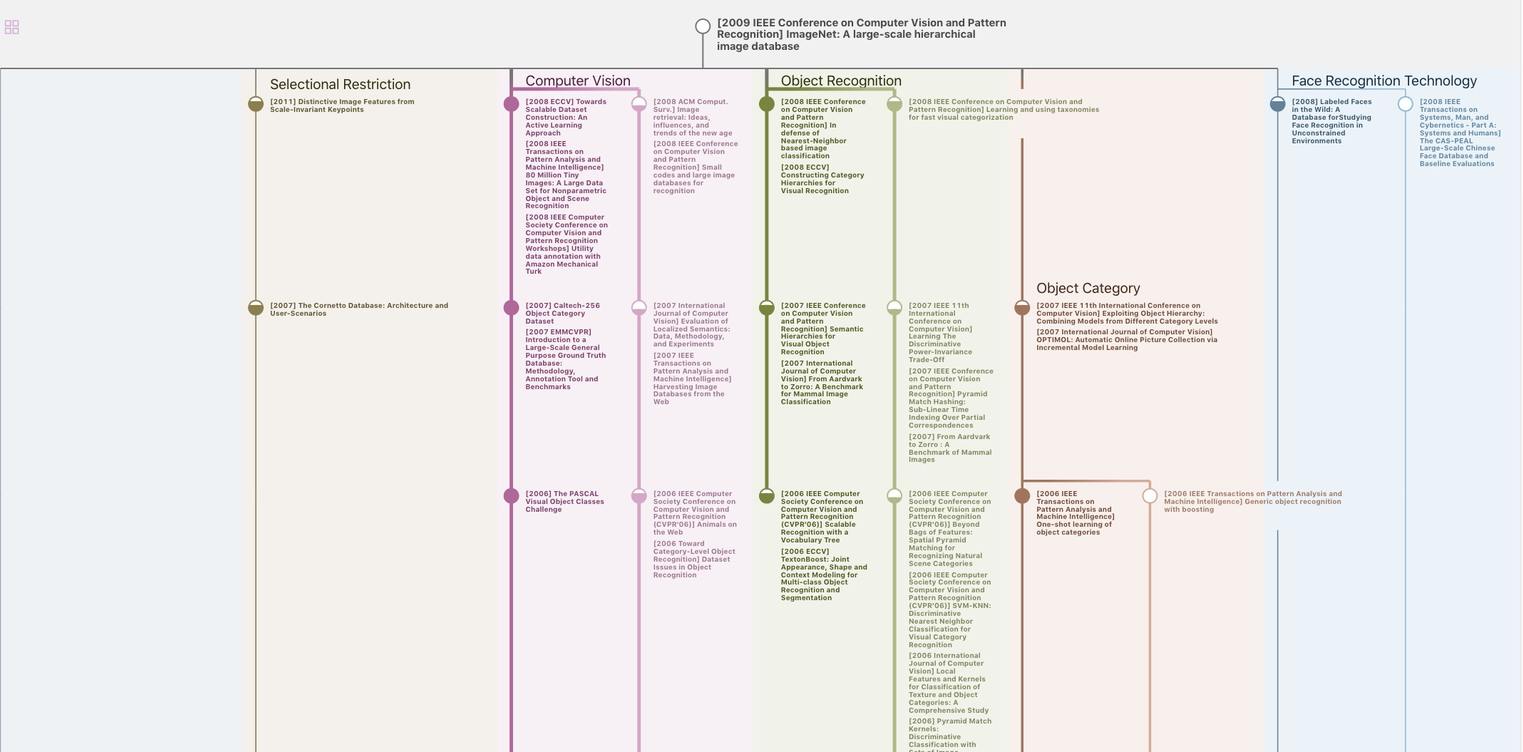
生成溯源树,研究论文发展脉络
Chat Paper
正在生成论文摘要