Discovering Graph Differential Dependencies
DATABASES THEORY AND APPLICATIONS, ADC 2023(2024)
摘要
Graph differential dependencies (GDDs) are a novel class of integrity constraints in property graphs for capturing and expressing the semantics of difference in graph data. They are more expressive, and subsume other graph dependencies; and thus, are more useful for addressing many real-world graph data quality/management problems. In this paper, we study the general discovery problem for GDDs - the task of finding a non-redundant and succinct set of GDDs that hold in a given property graph. Indeed, we present characterisations of GDDs based on their semantics, extend existing data structures, and device pruning strategies to enable our proposed level-wise discovery algorithm, GDDMiner, returns a minimal cover of valid GDDs efficiently. Further, we perform experiments over three real-world graphs to demonstrate the feasibility, scalability, and effectiveness of our solution.
更多查看译文
关键词
Graph differential dependency,Graph dependencies,Data dependencies,Dependency discovery
AI 理解论文
溯源树
样例
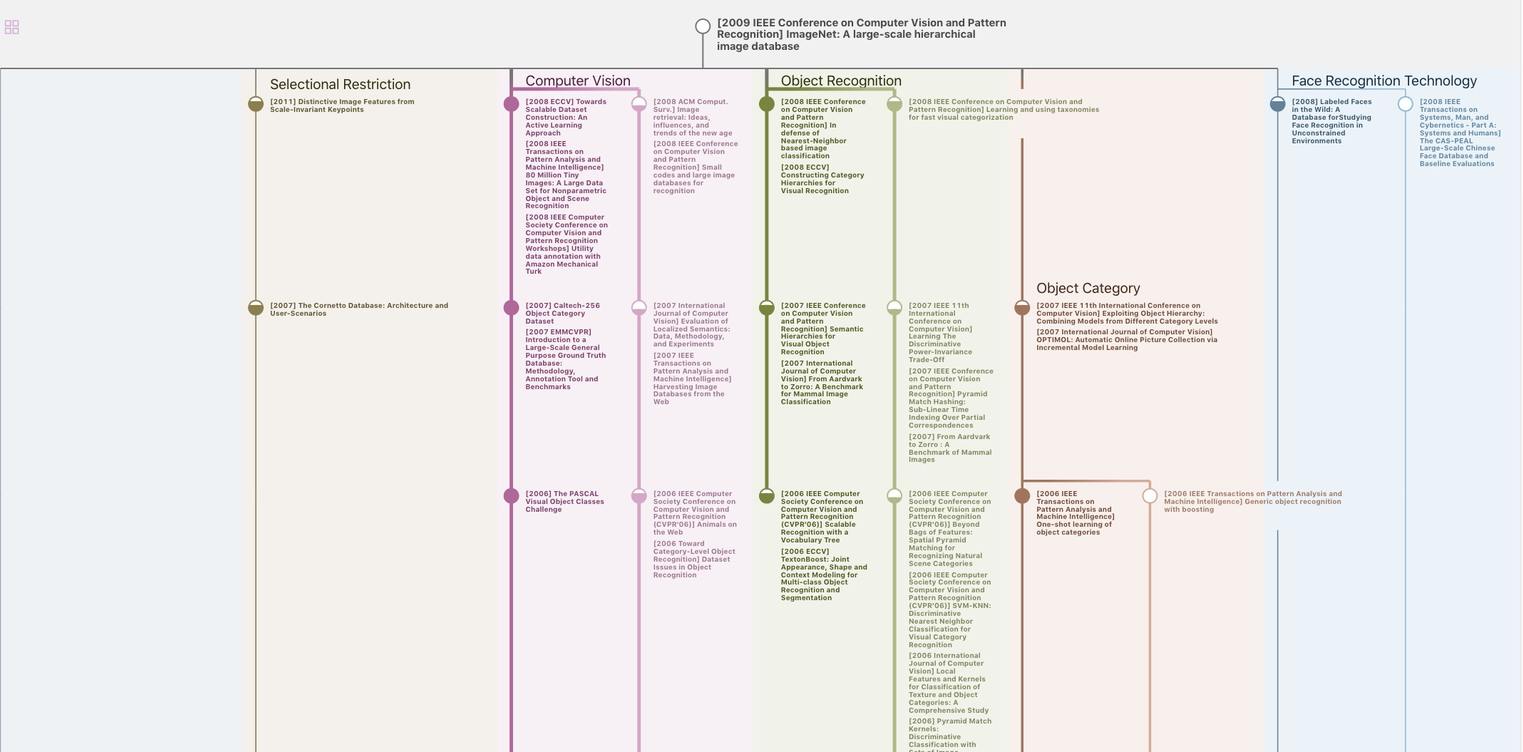
生成溯源树,研究论文发展脉络
Chat Paper
正在生成论文摘要